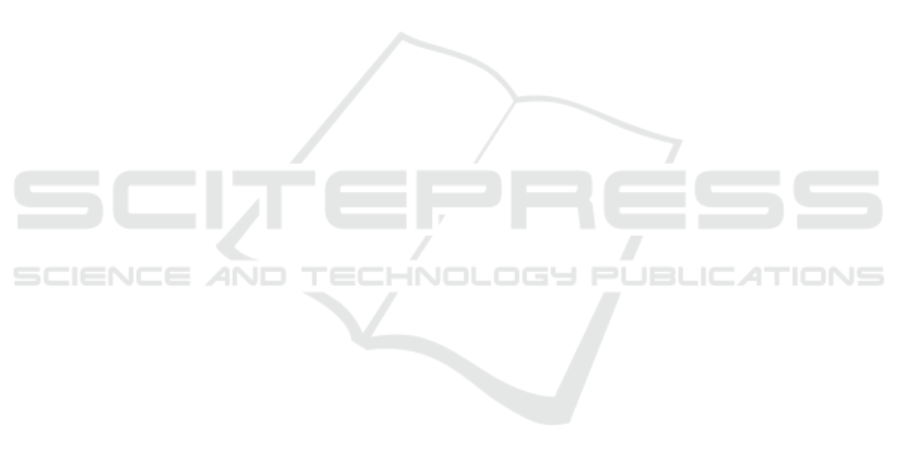
Domingos, P. and Pazzani, M. (1997). On the optimality
of the simple bayesian classifier under zero-one loss.
Machine learning, 29(2-3):103–130.
Dwork, C., Immorlica, N., Kalai, A. T., and Leiserson,
M. D. (2018). Decoupled classifiers for group-fair and
efficient machine learning. In Conference on Fairness,
Accountability and Transparency, pages 119–133.
Friedler, S. A., Scheidegger, C., Venkatasubramanian,
S., Choudhary, S., Hamilton, E. P., and Roth, D.
(2018). A comparative study of fairness-enhancing
interventions in machine learning. arXiv preprint
arXiv:1802.04422.
Garcia, M. (2016). Racist in the machine: The disturbing
implications of algorithmic bias. World Policy Jour-
nal, 33(4):111–117.
Goel, N., Yaghini, M., and Faltings, B. (2018). Non-
discriminatory machine learning through convex fair-
ness criteria. In Proceedings of the Thirty-Second
AAAI Conference on Artificial Intelligence, New Or-
leans, Louisiana, USA.
Goldberg, D., Nichols, D., Oki, B. M., and Terry, D. (1992).
Using collaborative filtering to weave an information
tapestry. Communications of the ACM, 35(12):61–70.
Griffiths, T. L. and Kalish, M. L. (2005). A bayesian view
of language evolution by iterated learning. In Procee-
dings of the Cognitive Science Society, volume 27.
Hajian, S., Bonchi, F., and Castillo, C. (2016). Algorithmic
bias: From discrimination discovery to fairness-aware
data mining. In Proceedings of the 22nd ACM
SIGKDD international conference on knowledge dis-
covery and data mining, pages 2125–2126. ACM.
Hosmer Jr, D. W., Lemeshow, S., and Sturdivant, R. X.
(2013). Applied logistic regression, volume 398. John
Wiley & Sons.
Jannach, D., Kamehkhosh, I., and Bonnin, G. (2016). Bi-
ases in automated music playlist generation: A com-
parison of next-track recommending techniques. In
Proceedings of the 2016 Conference on User Mo-
deling Adaptation and Personalization, pages 281–
285. ACM.
Joachims, T., Swaminathan, A., and Schnabel, T. (2017).
Unbiased learning-to-rank with biased feedback. In
Proceedings of the Tenth ACM International Confe-
rence on Web Search and Data Mining, pages 781–
789. ACM.
Kirby, S., Griffiths, T., and Smith, K. (2014). Iterated lear-
ning and the evolution of language. Current opinion
in neurobiology, 28:108–114.
Klayman, J. and Ha, Y.-W. (1987). Confirmation, discon-
firmation, and information in hypothesis testing. Psy-
chological review, 94(2):211.
Kleinberg, J., Ludwig, J., Mullainathan, S., and Ramba-
chan, A. (2018). Algorithmic fairness. In AEA Papers
and Proceedings, volume 108, pages 22–27.
Koren, Y., Bell, R., and Volinsky, C. (2009). Matrix factori-
zation techniques for recommender systems. Compu-
ter, 42(8).
Lambrecht, A. and Tucker, C. E. (2018). Algorithmic bias?
an empirical study into apparent gender-based discri-
mination in the display of stem career ads.
Liang, D., Charlin, L., McInerney, J., and Blei, D. M.
(2016). Modeling user exposure in recommendation.
In Proceedings of the 25th International Conference
on World Wide Web, pages 951–961. International
World Wide Web Conferences Steering Committee.
McNair, D. S. (2018). Preventing disparities: Bayesian and
frequentist methods for assessing fairness in machine-
learning decision-support models.
Nasraoui, O. and Pavuluri, M. (2004). Complete this puz-
zle: a connectionist approach to accurate web recom-
mendations based on a committee of predictors. In
International Workshop on Knowledge Discovery on
the Web, pages 56–72. Springer.
Nasraoui, O. and Shafto, P. (2016). Human-algorithm
interaction biases in the big data cycle: A markov
chain iterated learning framework. arXiv preprint
arXiv:1608.07895.
Pazzani, M. and Billsus, D. (1997). Learning and revising
user profiles: The identification of interesting web si-
tes. Machine learning, 27(3):313–331.
Robertson, S. E. (1977). The probability ranking principle
in ir. Journal of documentation, 33(4):294–304.
Rothman, K. J., Greenland, S., and Lash, T. L. (2008). Mo-
dern epidemiology. Lippincott Williams & Wilkins.
Schnabel, T., Swaminathan, A., Singh, A., Chandak, N.,
and Joachims, T. (2016). Recommendations as treat-
ments: Debiasing learning and evaluation. arXiv pre-
print arXiv:1602.05352.
Settles, B. (2010). Active learning literature survey. Uni-
versity of Wisconsin, Madison, 52(55-66):11.
Shafto, P. and Nasraoui, O. (2016). Human-recommender
systems: From benchmark data to benchmark cogni-
tive models. In Proceedings of the 10th ACM Con-
ference on Recommender Systems, pages 127–130.
ACM.
Smith, K. (2009). Iterated learning in populations of baye-
sian agents. In Proceedings of the 31st annual confe-
rence of the cognitive science society, pages 697–702.
Citeseer.
Spark, K. J. (1978). Artificial intelligence: What can it offer
to information retrieval. Proceedings of the Informa-
tics 3, Aslib, ed., London.
Spinelli, L. and Crovella, M. (2017). Closed-loop opinion
formation. In Proceedings of the 2017 ACM on Web
Science Conference, pages 73–82. ACM.
Stuart, A., Ord, J. K., and Kendall, S. M. (1994). Distribu-
tion theory. Edward Arnold; New York.
Zhang, X., Zhao, J., and Lui, J. (2017). Modeling the
assimilation-contrast effects in online product rating
systems: Debiasing and recommendations. In Pro-
ceedings of the Eleventh ACM Conference on Recom-
mender Systems, pages 98–106. ACM.
KDIR 2018 - 10th International Conference on Knowledge Discovery and Information Retrieval
118