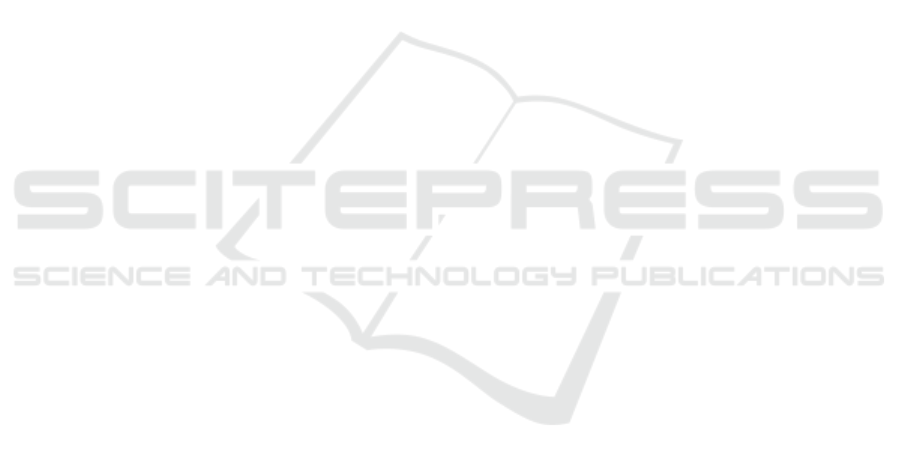
REFERENCES
Abisheva, A., Garcia, D., and Schweitzer, F. (2016). When
the filter bubble bursts: collective evaluation dynamics
in online communities. In Proceedings of the 8th ACM
Conference on Web Science, pages 307–308. ACM.
Badami, M., Nasraoui, O., Sun, W., and Shafto, P. (2017).
Detecting polarization in ratings: An automated pi-
peline and a preliminary quantification on several ben-
chmark data sets. In Big Data (Big Data), 2017 IEEE
International Conference on. IEEE.
Badami, M., Tafazzoli, F., and Nasraoui, O. (2018). A case
study for intelligent event recommendation. Interna-
tional Journal of Data Science and Analytics, pages
1–20.
Baeza-Yates, R. (2016). Data and algorithmic bias in the
web. In Proceedings of the 8th ACM Conference on
Web Science, pages 1–1. ACM.
Bobadilla, J., Ortega, F., Hernando, A., and Guti
´
errez, A.
(2013). Recommender systems survey. Knowledge-
based systems, 46:109–132.
Bozdag, E. (2013). Bias in algorithmic filtering and per-
sonalization. Ethics and information technology,
15(3):209–227.
Caliskan, A., Bryson, J. J., and Narayanan, A. (2017). Se-
mantics derived automatically from language corpora
contain human-like biases. Science, 356(6334):183–
186.
Dandekar, P., Goel, A., and Lee, D. T. (2013). Biased as-
similation, homophily, and the dynamics of polariza-
tion. Proceedings of the National Academy of Scien-
ces, 110(15):5791–5796.
Fish, B., Kun, J., and Lelkes,
´
A. D. (2016). A confidence-
based approach for balancing fairness and accuracy.
In Proceedings of the 2016 SIAM International Con-
ference on Data Mining, pages 144–152. SIAM.
Flaxman, S., Goel, S., and Rao, J. (2016). Filter bubbles,
echo chambers, and online news consumption. Public
Opinion Quarterly, page nfw006.
Garimella, K., De Francisci Morales, G., Gionis, A., and
Mathioudakis, M. (2016a). Quantifying controversy
in social media. In Proceedings of the Ninth ACM In-
ternational Conference on Web Search and Data Mi-
ning, pages 33–42. ACM.
Garimella, K., Morales, G. D. F., Gionis, A., and Mathiou-
dakis, M. (2016b). Balancing opposing views to re-
duce controversy. arXiv preprint arXiv:1611.00172.
Hajian, S., Bonchi, F., and Castillo, C. (2016). Algorithmic
bias: From discrimination discovery to fairness-aware
data mining. In Proceedings of the 22nd ACM
SIGKDD international conference on knowledge dis-
covery and data mining, pages 2125–2126. ACM.
Hardt, M., Price, E., Srebro, N., et al. (2016). Equality of
opportunity in supervised learning. In Advances in
neural information processing systems, pages 3315–
3323.
Isenberg, D. J. (1986). Group polarization: A critical review
and meta-analysis. Journal of personality and social
psychology, 50(6):1141.
Kamishima, T., Akaho, S., and Sakuma, J. (2011). Fairness-
aware learning through regularization approach. In
Data Mining Workshops (ICDMW), 2011 IEEE 11th
International Conference on, pages 643–650. IEEE.
Kirkpatrick, K. (2016). Battling algorithmic bias: How do
we ensure algorithms treat us fairly? Communications
of the ACM, 59(10):16–17.
Knijnenburg, B. P., Sivakumar, S., and Wilkinson, D.
(2016). Recommender systems for self-actualization.
In Proceedings of the 10th ACM Conference on Re-
commender Systems, pages 11–14. ACM.
Koren, Y., Bell, R., and Volinsky, C. (2009). Matrix factori-
zation techniques for recommender systems. Compu-
ter, 42(8).
Lambrecht, A. and Tucker, C. E. (2018). Algorithmic bias?
an empirical study into apparent gender-based discri-
mination in the display of stem career ads.
Liao, Q. V. and Fu, W.-T. (2014). Can you hear me now?:
mitigating the echo chamber effect by source position
indicators. In Proceedings of the 17th ACM confe-
rence on Computer supported cooperative work & so-
cial computing, pages 184–196. ACM.
Lord, C. G., Ross, L., and Lepper, M. R. (1979). Bi-
ased assimilation and attitude polarization: The ef-
fects of prior theories on subsequently considered evi-
dence. Journal of personality and social psychology,
37(11):2098.
Matakos, A. and Tsaparas, P. (2016). Temporal mechanisms
of polarization in online reviews. In Advances in So-
cial Networks Analysis and Mining (ASONAM), 2016
IEEE/ACM International Conference on, pages 529–
532. IEEE.
Mejova, Y., Zhang, A. X., Diakopoulos, N., and Castillo,
C. (2014). Controversy and sentiment in online news.
arXiv preprint arXiv:1409.8152.
Melville, P. and Sindhwani, V. (2011). Recommender sy-
stems. In Encyclopedia of machine learning, pages
829–838. Springer.
Morales, A., Borondo, J., Losada, J. C., and Benito,
R. M. (2015). Measuring political polarization:
Twitter shows the two sides of venezuela. Chaos:
An Interdisciplinary Journal of Nonlinear Science,
25(3):033114.
Munson, S. A. and Resnick, P. (2010). Presenting diverse
political opinions: how and how much. In Procee-
dings of the SIGCHI conference on human factors in
computing systems, pages 1457–1466. ACM.
Nasraoui, O. and Shafto, P. (2016). Human-algorithm inte-
raction biases in the big data cycle: A markov chain
iterated learning framework. CoRR, abs/1608.07895.
Shafto, P. and Nasraoui, O. (2016). Human-recommender
systems: From benchmark data to benchmark cogni-
tive models. In Proceedings of the 10th ACM Con-
ference on Recommender Systems, pages 127–130.
ACM.
Sun, W., Nasraoui, O., and shafto, P. (2018). Iterated algo-
rithmic bias in the interactive machine learning pro-
cess of information filtering. In Proceedings of the
10th Of the Knowledge Discovery and Information
Retrieval conference.
Zhang, X., Zhao, J., and Lui, J. C. (2017). Modeling the
assimilation-contrast effects in online product rating
systems: Debiasing and recommendations. In Procee-
dings of the Eleventh ACM Conference on Recommen-
der Systems, RecSys ’17, pages 98–106, New York,
NY, USA. ACM.
PrCP: Pre-recommendation Counter-Polarization
289