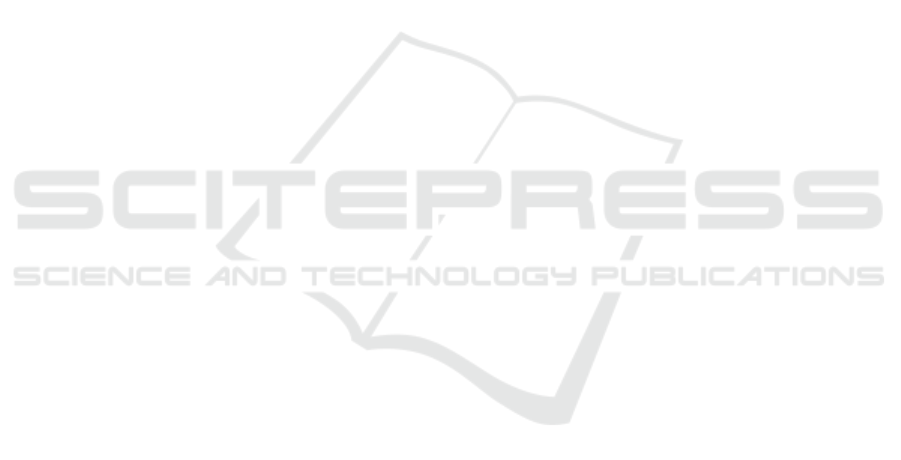
of Certainty keeping the size of the training dataset
constant.
REFERENCES
Checco, A., Roitero, A., Maddalena, E., Mizzaro, S., and
Demartini, G. (2017). Lets agree to disagree: Fix-
ing agreement measures for crowdsourcing. In Pro-
ceedings of the Fifth AAAI Conference on Human
Computation and Crowdsourcing (HCOMP-17), pa-
ges 11–20. AAAI Press.
Dawid, A. P. and Skene, A. M. (1979). Maximum likeli-
hood estimation of observer error-rates using the EM
algorithm. Applied Statistics, pages 20–28.
Ekman, P. (1992). An argument for basic emotions. Cogni-
tion & emotion, 6:169–200.
Gupta, U., Chatterjee, A., Srikanth, R., and Agrawal, P.
(2017). A sentiment-and-semantics-based approach
for emotion detection in textual conversations. arXiv
preprint arXiv:1707.06996.
Hochreiter, S. and Schmidhuber, J. (1997). Long short-term
memory. Neural computation, 9:1735–1780.
Joulin, A., Grave, E., Bojanowski, P., and Mikolov, T.
(2016). Bag of tricks for efficient text classification.
arXiv preprint arXiv:1607.01759.
Karger, D. R., Oh, S., and Shah, D. (2011). Iterative lear-
ning for reliable crowdsourcing systems. In Advan-
ces in Neural Information Processing Systems, pages
1953–1961.
Kazai, G., Kamps, J., and Milic-Frayling, N. (2011). Wor-
ker types and personality traits in crowdsourcing rele-
vance labels. In Proceedings of the 20th ACM Inter-
national Conference on Information and Knowledge
Management, pages 1941–1944. ACM.
LeCun, Y., Bengio, Y., and Hinton, G. (2015). Deep lear-
ning. Nature, 521(7553):436.
Li, H. and Yu, B. (2014). Error rate bounds and iterative
weighted majority voting for crowdsourcing. arXiv
preprint arXiv:1411.4086.
Liu, Q., Peng, J., and Ihler, A. T. (2012). Variational infe-
rence for crowdsourcing. In Advances in Neural In-
formation Processing Systems, pages 692–700.
Mikolov, T., Sutskever, I., Chen, K., Corrado, G. S., and
Dean, J. (2013). Distributed representations of words
and phrases and their compositionality. In Advances in
neural information processing systems, pages 3111–
3119.
Pennington, J., Socher, R., and Manning, C. D. (2014).
Glove: Global vectors for word representation. In
EMNLP, volume 14, pages 1532–1543.
Plutchik, R. and Kellerman, H. (1986). Emotion: theory,
research and experience. Academic press New York.
Raykar, V. C. and Yu, S. (2012). Eliminating spammers and
ranking annotators for crowdsourced labeling tasks.
Journal of Machine Learning Research, 13(Feb):491–
518.
Raykar, V. C., Yu, S., Zhao, L. H., Valadez, G. H., Flo-
rin, C., Bogoni, L., and Moy, L. (2010). Learning
from crowds. Journal of Machine Learning Research,
11(Apr):1297–1322.
Robbins, H. and Monro, S. (1951). A stochastic approxi-
mation method. The annals of mathematical statistics,
pages 400–407.
Smyth, P., Fayyad, U. M., Burl, M. C., Perona, P., and Baldi,
P. (1995). Inferring ground truth from subjective la-
belling of venus images. In Advances in Neural Infor-
mation Processing Systems, pages 1085–1092.
Steinhardt, J., Valiant, G., and Charikar, M. (2016). Avoi-
ding imposters and delinquents: Adversarial crowd-
sourcing and peer prediction. In Advances in Neural
Information Processing Systems, pages 4439–4447.
Tang, D., Wei, F., Yang, N., Zhou, M., Liu, T., and Qin, B.
(2014). Learning sentiment-specific word embedding
for twitter sentiment classification. In Proceedings of
the 52nd Annual Meeting of the Association for Com-
putational Linguistics, pages 1555–1565.
Thilmany, J. (2007). The emotional robot: Cognitive com-
puting and the quest for artificial intelligence. EMBO
reports, 8(11):992–994.
Wang, G., Wang, T., Zheng, H., and Zhao, B. Y. (2014).
Man vs. machine: Practical adversarial detection of
malicious crowdsourcing workers. In USENIX Secu-
rity Symposium, pages 239–254.
Wauthier, F. L. and Jordan, M. I. (2011). Bayesian bias
mitigation for crowdsourcing. In Advances in Neural
Information Processing Systems, pages 1800–1808.
Welinder, P., Branson, S., Perona, P., and Belongie, S. J.
(2010). The multidimensional wisdom of crowds. In
Advances in Neural Information Processing Systems,
pages 2424–2432.
Whitehill, J., Wu, T.-f., Bergsma, J., Movellan, J. R.,
and Ruvolo, P. L. (2009). Whose vote should count
more: Optimal integration of labels from labelers of
unknown expertise. In Advances in Neural Informa-
tion Processing Systems, pages 2035–2043.
Zhou, D., Basu, S., Mao, Y., and Platt, J. C. (2012). Le-
arning from the wisdom of crowds by minimax en-
tropy. In Advances in Neural Information Processing
Systems, pages 2195–2203.
Zhou, D., Liu, Q., Platt, J., and Meek, C. (2014). Aggrega-
ting ordinal labels from crowds by minimax conditio-
nal entropy. In International Conference on Machine
Learning, pages 262–270.
A Case Study on using Crowdsourcing for Ambiguous Tasks
247