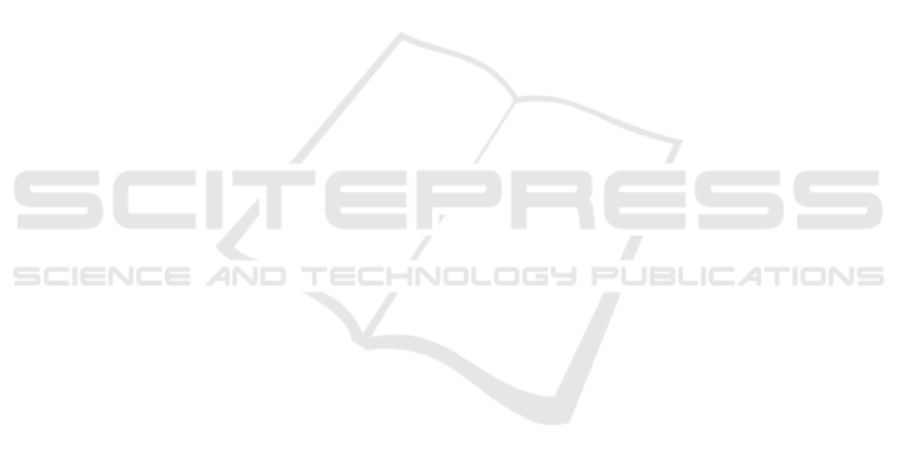
REFERENCES
Atanassov, K. T. (1986). Intuitionistic fuzzy sets. Fuzzy sets
and Systems, 20(1):87–96.
Atanassov, K. T. (2012). On Intuitionistic Fuzzy Sets The-
ory, volume 283 of Studies in Fuzziness and Soft Com-
puting. Springer Berlin Heidelberg, Berlin, Heidel-
berg.
Atanassova, V. (2010). Representation of fuzzy and intuiti-
onistic fuzzy data by radar charts. Notes on Intuitio-
nistic Fuzzy Sets, 16(1):21–26.
Bouyssou, D., Dubois, D., Prade, H., and Pirlot, M. (2013).
Decision Making Process: Concepts and Methods.
John Wiley & Sons.
Dong, Y., Xiao, J., Zhang, H., and Wang, T. (2016). Ma-
naging consensus and weights in iterative multiple-
attribute group decision making. Applied Soft Com-
puting, 48:80 – 90.
Herrera, F., Herrera-Viedma, E., and Verdegay, J. L. (1996).
A model of consensus in group decision making un-
der linguistic assessments. Fuzzy Sets and Systems,
78(1):73 – 87.
Herrera, F., Herrera-Viedma, E., and Verdegay, J. L. (1997).
A rational consensus model in group decision making
using linguistic assessments. Fuzzy Sets and Systems,
88(1):31 – 49.
Kacprzyk, J. and Fedrizzi, M. (1988). A ‘soft’ measure of
consensus in the setting of partial (fuzzy) preferences.
European Journal of Operational Research, 34(3):316
– 325.
Kacprzyk, J. and Zadro
˙
zny, S. (2005). Linguistic database
summaries and their protoforms: towards natural lan-
guage based knowledge discovery tools. Information
Sciences, 173(4):281 – 304. Dealing with Uncertainty
in Data Mining and Information Extraction.
Kacprzyk, J. and Zadro
˙
zny, S. (2010). Soft computing and
web intelligence for supporting consensus reaching.
Soft Computing, 14(8):833–846.
Liu, Y., Fan, Z.-P., and Zhang, X. (2016). A method
for large group decision-making based on evaluation
information provided by participators from multiple
groups. Information Fusion, 29:132–141.
Loor, M. and De Tr
´
e, G. (2017a). On the Need for Augmen-
ted Appraisal Degrees to Handle Experience-Based
Evaluations. Applied Soft Computing, 54C:284 – 295.
Loor, M. and De Tr
´
e, G. (2017b). In a Quest for Suitable
Similarity Measures to Compare Experience-Based
Evaluations. In Merelo, J. J., Rosa, A., Cadenas, J. M.,
Correia, A. D., Madani, K., Ruano, A., and Filipe,
J., editors, Computational Intelligence: International
Joint Conference, IJCCI 2015 Lisbon, Portugal, No-
vember 12-14, 2015, Revised Selected Papers, volume
669 of Studies in Computational Intelligence, pages
291–314. Springer International Publishing.
Loor, M. and De Tr
´
e, G. (2017c). An open-source software
package to assess similarity measures that compare
intuitionistic fuzzy sets. In 2017 IEEE International
Conference on Fuzzy Systems (FUZZ-IEEE), pages 1–
6.
Loor, M., Tapia-Rosero, A., and De Tr
´
e, G. (2018). Refo-
cusing attention on unobserved attributes to reach con-
sensus in decision making problems involving a hete-
rogeneous group of experts. In Kacprzyk, J., Szmidt,
E., Zadro
˙
zny, S., Atanassov, K. T., and Krawczak,
M., editors, Advances in Fuzzy Logic and Technology
2017, pages 405–416, Springer International Publis-
hing.
Szmidt, E. and Kacprzyk, J. (2002). Evaluation of
agreement in a group of experts via distances bet-
ween intuitionistic fuzzy preferences. In Proceedings
First International IEEE Symposium Intelligent Sys-
tems, volume 1, pages 166–170 vol.1.
Szmidt, E. and Kacprzyk, J. (2003). A consensus rea-
ching process under intuitionistic fuzzy preference re-
lations. International Journal of Intelligent Systems,
18(7):837–852.
Szmidt, E. and Kacprzyk, J. (2004). A concept of simila-
rity for intuitionistic fuzzy sets and its use in group
decision making. In IEEE International Conference
on Fuzzy Systems, pages 1129–1134.
APPENDIX
Evaluations about the ‘best smooth dip’
This appendix presents the evaluations given by 11
persons who tried to reach a consensus about the best
smooth dip(s), among 3 potential dips, to pair with
banana chips. These evaluations have been graphi-
cally represented by means of IFS contrasting charts
(IFSCCs) (see Section 3.2).
Figure 12 shows the IFSCCs corresponding to the
evaluations given or computed during the first round
of the consensus reaching process: while Figure 12(a)
represents the collective evaluations computed for the
group, Figures 12(b)-12(l) represent the individual
evaluations given by these 11 persons respectively.
In a similar way, Figure 13 shows the IFSCCs corre-
sponding to the evaluations given or computed during
the second round.
Usability of Concordance Indices in FAST-GDM Problems
77