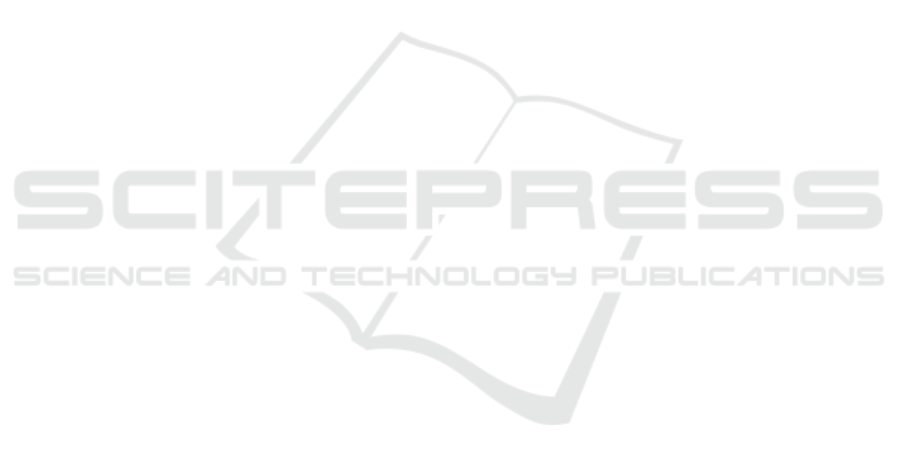
2004; Camerer et al., 2004; Henrich et al., 2005))
is a response to the desire to introduce psychologi-
cal realism and social influence into game theory by
incorporating notions such as fairness and reciprocity
into preferences in addition to considerations of ma-
terial benefit. The closely related field of psychologi-
cal game theory (cf. (Geanakoplos et al., 1989; Duf-
wenberg and Kirchsteiger, 2004; Colman, 2003; Bat-
tigalli and Dufwenberg, 2009; Gilboa and Schmeid-
ler, 1988)) also employs preferences that account for
beliefs as well as actions and takes into consideration
belief-dependent motivations such as guilt aversion,
reciprocity, regret, and shame. The concept of “team-
reasoning” has been promoted by (Sugden, 2015) and
(Bacharach, 2006), where individuals view themsel-
ves as members of a team, and therefore are moti-
vated to modify their behavior to conform with team
aspirations. (Hedahl and Huebner, 2018) focus on va-
lue sharing and discuss processes for providing nor-
mative grounding for pursuing shared ends. (Reisch-
mann and Oechssler, 2018) introduce a mechanism
for public good provision using conditional offers ba-
sed on the willingness of others to contribute.
A thread common to these approaches is that they
rely on ex ante linear preference orderings that are sta-
tic, immutable, global, and unconditional—they are
categorical. We argue that this single thread must be
replaced by a richer interweave of preference relati-
onships that involve explicit social influence.
The perspectives that comport most closely with
this paper are the views held by (Ross, 2014) and
(Bratman, 2014). Ross asserts that individual prefe-
rences are not formed in a social vacuum; rather, they
are the consequence of social processes, and must the-
refore be dependent on the social environment. Brat-
man argues similarly, and introduces a notion of aug-
mented individualism, where the intentions of an indi-
vidual are composed of relevant interrelated attitudes,
leading to a notion of shared agency. Essentially, con-
ditional game theory is the operationalization of these
two perspectives.
6 CONCLUSIONS
Conditional game theory offers a significant extension
of standard game theory as a framework for both the
analysis of human networks and the design and synt-
hesis of artificial social influence networks.
• Social influence is ex ante incorporated endoge-
nously into the payoffs rather than exogenously
imposed via an ex post solution concept.
• An operational definition of coordination is gene-
rated as a group-level attribute that is considered
parallel to the individual-level attribute of prefe-
rence.
• Individual coordinated decisions are deduced as a
consequence of the diffusion of social influence
throughout the network.
REFERENCES
Arrow, H., McGrath, J. E., and Berdahl, J. L. (2000). Small
Groups as Complex Systems. Sage Publications, Inc.,
Thousand Oaks, CA.
Axelrod, R. (1984). The Evolution of Cooperation. Basic
Books, New York.
Bacharach, M. (2006). Beyond Individual Choice: Teams
and Frames in Game Theory. Princeton University
Press, Princeton, NJ.
Battigalli, P. and Dufwenberg, M. (2009). Dynamic psycho-
logical games. Journal of Economic Theory, 144:1–
35.
Bicchieri, C. (2003). Rationality and Coordination. Cam-
bridge University Press, Cambridge, UK.
Bolton, G. E. and Ockenfels, A. (2005). A stress test of
fairness measures in models of social utility. Econo-
mic Theory, 24(4).
Bossert, W., Qi, C. X., and Weymark, J. A. (2012). Mea-
suring group fitness in a biological hierarchy: an ax-
iomatic social choice approach. Economics and Phi-
losophy, 29:301–323.
Bratman, M. (2014). Shared Agency. Oxford University
Press, Oxford, UK.
Camerer, C., Lowenstein, G., and Rabin, M., editors (2004).
Advances in Behavorial Economics. Princeton Univ.
Press, Princeton, NJ.
Colman, A. M. (2003). Cooperation, psychological game
theory, and limitations of rationality in social inte-
raction. Behavioral and Brain Sciences, 26:139–198.
Coser, L. A. (1971). Masters of Sociological Thought. Har-
court Brace Jovanovich, Inc., New York, NY.
DeGroot, M. H. (1974). Reaching a consensus. Journal of
the American Statistical Association, 69:118–121.
Dufwenberg, M. and Kirchsteiger, G. (2004). A theory of
sequential reciprocity. Games and Economic Beha-
vior, 47:268–298.
Easley, D. and Kleinberg, J. (2010). Networks, Crowds, and
Markets: Reasoning about a Highly Connected World.
Cambridge University Press, Cambridge.
Fefferman, N. H. and Ng, K. L. (2007). The role of in-
dividual choice in the evolution of social complexity.
Annales Zoologici Fennici, 44:58–69.
Fehr, E. and Schmidt, K. (1999). A theory of fairness, com-
petition, and cooperation. Quarterly Journal of Eco-
nomics, 114:817–868.
French, J. R. P. (1956). A formal theory of social power.
The Psychology Review, 63:181–194.
Friedkin, N. E. (1986). A formal theory of social power.
Journal of Mathematical Sociology, 12:103–136.
Conditional Game Theory as a Model for Coordinated Decision Making
301