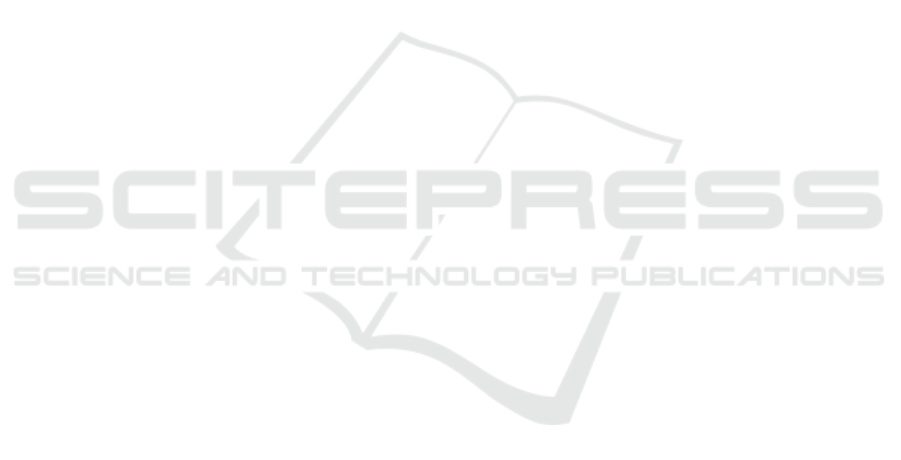
in this roadmap can in principle be also used for in-
ferential reasoning over ontologies: very briefly, the
probability distributions over ontologies provided by
such a model can be used to calculate quantities such
as e.g. the probability that an axiom will be contained
in an ontology given that certain other axioms are in
it and so forth. It is not possible to say much more at
this juncture regarding the feasibility of this type of
approach to statistical inference over ontologies; but
it is an intriguing idea that would certainly be deser-
ving of further examination after the development of
an adequate model for the generation of random on-
tologies.
REFERENCES
Airoldi, E. M., Blei, D. M., Fienberg, S. E., and Xing, E. P.
(2008). Mixed membership stochastic blockmodels.
Journal of Machine Learning Research, 9(Sep):1981–
2014.
Albert, R. and Barab
´
asi, A.-L. (2002). Statistical mecha-
nics of complex networks. Reviews of modern physics,
74(1):47.
Choi, N., Song, I.-Y., and Han, H. (2006). A survey on
ontology mapping. ACM Sigmod Record, 35(3):34–
41.
Codescu, M., Kuksa, E., Kutz, O., Mossakowski, T., and
Neuhaus, F. (2017). Ontohub: A semantic repository
engine for heterogeneous ontologies. Applied Onto-
logy, 12(3–4):275–298.
Cristani, M. and Cuel, R. (2005). A survey on onto-
logy creation methodologies. International Journal
on Semantic Web and Information Systems (IJSWIS),
1(2):49–69.
Dragisic, Z., Eckert, K., Euzenat, J., Faria, D., Ferrara, A.,
Granada, R., Ivanova, V., Jim
´
enez-Ruiz, E., Kempf,
A. O., Lambrix, P., et al. (2014). Results of the onto-
logy alignment evaluation initiative 2014. In Proc. of
the 9th Int. Conf. on Ontology Matching-Volume 1317,
pages 61–104. CEUR-WS. org.
Duque-Ramos, A., Fern
´
andez-Breis, J. T., Stevens, R.,
Aussenac-Gilles, N., et al. (2011). Oquare: A square-
based approach for evaluating the quality of ontolo-
gies. Journal of Research and Practice in Information
Technology, 43(2):159.
Erd
¨
os, P. and R
´
enyi, A. (1959). On random graphs, I. Pu-
blicationes Mathematicae (Debrecen), 6:290–297.
Garc
´
ıa, J., JoseGarc
´
ıa-Pe
˜
nalvo, F., and Ther
´
on, R. (2010).
A survey on ontology metrics. In World Summit on
Knowledge Society, pages 22–27. Springer.
Granitzer, M., Sabol, V., Onn, K. W., Lukose, D., and Toch-
termann, K. (2010). Ontology alignmenta survey with
focus on visually supported semi-automatic techni-
ques. Future Internet, 2(3):238–258.
Grover, A. and Leskovec, J. (2016). node2vec: Scalable
feature learning for networks. In Proc. of the 22nd
ACM SIGKDD Int. Conf. on Knowledge discovery and
data mining, pages 855–864. ACM.
Guo, Y., Pan, Z., and Heflin, J. (2005). LUBM: a bench-
mark for OWL knowledge base systems. Web Seman-
tics: Science, Services and Agents on the World Wide
Web, 3(2-3):158–182.
Hamilton, W., Ying, Z., and Leskovec, J. (2017a). Inductive
representation learning on large graphs. In Advan-
ces in Neural Information Processing Systems, pages
1025–1035.
Hamilton, W. L., Ying, R., and Leskovec, J. (2017b). Re-
presentation learning on graphs: Methods and appli-
cations. arXiv preprint arXiv:1709.05584.
Horridge, M., Bail, S., Parsia, B., and Sattler, U. (2011).
The cognitive complexity of owl justifications. In
International Semantic Web Conference, pages 241–
256. Springer.
Kang, Y.-B., Li, Y.-F., and Krishnaswamy, S. (2012). Pre-
dicting reasoning performance using ontology me-
trics. In International Semantic Web Conference, pa-
ges 198–214. Springer.
Katifori, A., Halatsis, C., Lepouras, G., Vassilakis, C.,
and Giannopoulou, E. (2007). Ontology visualization
methodsa survey. ACM Computing Surveys (CSUR),
39(4):10.
Leskovec, J., Chakrabarti, D., Kleinberg, J., Faloutsos, C.,
and Ghahramani, Z. (2010). Kronecker graphs: An
approach to modeling networks. Journal of Machine
Learning Research, 11(Feb):985–1042.
Lozano-Tello, A. and G
´
omez-P
´
erez, A. (2004). Ontometric:
A method to choose the appropriate ontology. Journal
of database management, 2(15):1–18.
Ma, L., Yang, Y., Qiu, Z., Xie, G., Pan, Y., and Liu, S.
(2006). Towards a complete OWL ontology bench-
mark. In European Semantic Web Conference, pages
125–139. Springer.
Matentzoglu, N. and Parsia, B. (2018). BioPortal. last
accessed, 2018/26/05.
McDaniel, M., Storey, V. C., and Sugumaran, V. (2018).
Assessing the quality of domain ontologies: Metrics
and an automated ranking system. Data & Knowledge
Engineering.
Mossakowski, T., Codescu, M., Neuhaus, F., and Kutz, O.
(2015). The Road to Universal Logic: Festschrift for
the 50th Birthday of Jean-Yves B
´
eziau, Volume II.
chapter The Distributed Ontology, Modeling and Spe-
cification Language – DOL, pages 489–520. Springer
International Publishing, Cham.
Neuhaus, F., Vizedom, A., Baclawski, K., Bennett, M.,
Dean, M., Denny, M., Gr
¨
uninger, M., Hashemi, A.,
Longstreth, T., Obrst, L., et al. (2013). Towards on-
tology evaluation across the life cycle. Applied Onto-
logy, 8(3):179–194.
Ongenae, F., Verstichel, S., De Turck, F., Dhaene, T.,
Dhoedt, B., and Demeester, P. (2008). OTAGen: A tu-
nable ontology generator for benchmarking ontology-
based agent collaboration. In 32nd Annual IEEE
Int. Computer Software and Applications Conf., pages
529–530.
KEOD 2018 - 10th International Conference on Knowledge Engineering and Ontology Development
356