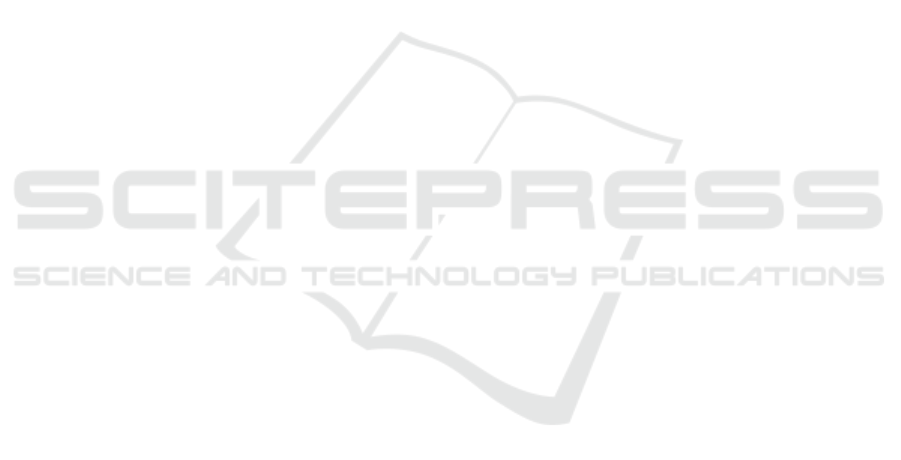
Evert, S. (2008). Corpora and collocations. Corpus linguis-
tics. An international handbook, 2:1212–1248.
Frermann, L. and Lapata, M. (2016). A bayesian model
of diachronic meaning change. Transactions of the
Association for Computational Linguistics, 4:31–45.
Gulordava, K. and Baroni, M. (2011). A distributional sim-
ilarity approach to the detection of semantic change
in the google books ngram corpus. In Proceedings of
the GEMS 2011 Workshop on GEometrical Models of
Natural Language Semantics, pages 67–71. Associa-
tion for Computational Linguistics.
Hamilton, W. L., Leskovec, J., and Jurafsky, D. (2016).
Diachronic word embeddings reveal statistical laws
of semantic change. In Proceedings of the 54th An-
nual Meeting of the Association for Computational
Linguistics (Volume 1: Long Papers). Association for
Computational Linguistics.
Harris, Z. S. (1954). Distributional structure. Word, 10(2-
3):146–162.
Jatowt, A. and Duh, K. (2014). A framework for analyz-
ing semantic change of words across time. In Digital
Libraries (JCDL), 2014 IEEE/ACM Joint Conference
on, pages 229–238. IEEE.
Jeffers, R. J. and Lehiste, I. (1979). Principles and methods
for historical linguistics. MIT press.
Kim, Y., Chiu, Y.-I., Hanaki, K., Hegde, D., and Petrov, S.
(2014). Temporal analysis of language through neural
language models. arXiv preprint arXiv:1405.3515.
Kulkarni, V., Al-Rfou, R., Perozzi, B., and Skiena, S.
(2015). Statistically significant detection of linguistic
change. In Proceedings of the 24th International Con-
ference on World Wide Web, pages 625–635. Interna-
tional World Wide Web Conferences Steering Com-
mittee.
Kulkarni, V., Perozzi, B., and Skiena, S. (2016). Fresh-
man or fresher? quantifying the geographic variation
of language in online social media. In ICWSM, pages
615–618.
Lau, J. H., Cook, P., McCarthy, D., Newman, D., and Bald-
win, T. (2012). Word sense induction for novel sense
detection. In Proceedings of the 13th Conference of
the European Chapter of the Association for Compu-
tational Linguistics, pages 591–601. Association for
Computational Linguistics.
Lehmann, W. P. (2013). Historical linguistics: an introduc-
tion. Routledge.
Levy, O., Goldberg, Y., and Dagan, I. (2015). Improv-
ing distributional similarity with lessons learned from
word embeddings. Transactions of the Association for
Computational Linguistics, 3:211–225.
Liao, X. and Cheng, G. (2016). Analysing the semantic
change based on word embedding. In International
Conference on Computer Processing of Oriental Lan-
guages, pages 213–223. Springer.
Lin, Y., Michel, J.-B., Aiden, E. L., Orwant, J., Brockman,
W., and Petrov, S. (2012). Syntactic annotations for
the google books ngram corpus. In Proceedings of
the ACL 2012 system demonstrations, pages 169–174.
Association for Computational Linguistics.
Manning, C. D., Raghavan, P., and Sch
¨
utze, H. (2008).
Evaluation in information retrieval, page 139–161.
Cambridge University Press.
Mark, D. (2010). The corpus of historical amer-
ican english: 400 million words, 1810–2009.
http://corpus.byu.edu/coha.
Mihalcea, R. and Nastase, V. (2012). Word epoch disam-
biguation: Finding how words change over time. In
Proceedings of the 50th Annual Meeting of the Asso-
ciation for Computational Linguistics: Short Papers-
Volume 2, pages 259–263. Association for Computa-
tional Linguistics.
Mikolov, T., Chen, K., Corrado, G., and Dean, J. (2013).
Efficient estimation of word representations in vector
space. arXiv preprint arXiv:1301.3781.
Ritter, A., Etzioni, O., et al. (2010). A latent dirichlet allo-
cation method for selectional preferences. In Proceed-
ings of the 48th Annual Meeting of the Association for
Computational Linguistics, pages 424–434. Associa-
tion for Computational Linguistics.
Sagi, E., Kaufmann, S., and Clark, B. (2011). Tracing se-
mantic change with latent semantic analysis. Current
methods in historical semantics, pages 161–183.
Sang, E. T. K. (2016). Finding rising and falling words.
LT4DH 2016, page 2.
S
´
eaghdha, D. O. (2010). Latent variable models of se-
lectional preference. In Proceedings of the 48th An-
nual Meeting of the Association for Computational
Linguistics, pages 435–444. Association for Compu-
tational Linguistics.
Simpson, J. A. and Weiner, E. S. C. (1989). The Oxford En-
glish dictionary, volume 2. Clarendon Press Oxford.
Spearman, C. (1904). The proof and measurement of asso-
ciation between two things. The American journal of
psychology, 15(1):72–101.
Takamura, H., Nagata, R., and Kawasaki, Y. (2017). An-
alyzing semantic change in japanese loanwords. In
Proceedings of the 15th Conference of the European
Chapter of the Association for Computational Lin-
guistics: Volume 1, Long Papers, volume 1, pages
1195–1204.
Taylor, W. A. (2000). Change-point analysis: a powerful
new tool for detecting changes.
Tissier, J., Gravier, C., and Habrard, A. (2017). Dict2vec:
Learning word embeddings using lexical dictionaries.
In Conference on Empirical Methods in Natural Lan-
guage Processing (EMNLP 2017), pages 254–263.
Wang, X. and McCallum, A. (2006). Topics over time: a
non-markov continuous-time model of topical trends.
In Proceedings of the 12th ACM SIGKDD interna-
tional conference on Knowledge discovery and data
mining, pages 424–433. ACM.
Wijaya, D. T. and Yeniterzi, R. (2011). Understanding se-
mantic change of words over centuries. In Proceed-
ings of the 2011 international workshop on DETecting
and Exploiting Cultural diversiTy on the social web,
pages 35–40. ACM.
Xu, Y. and Kemp, C. (2015). A computational evaluation
of two laws of semantic change. In CogSci.
Using Google Books Ngram in Detecting Linguistic Shifts over Time
339