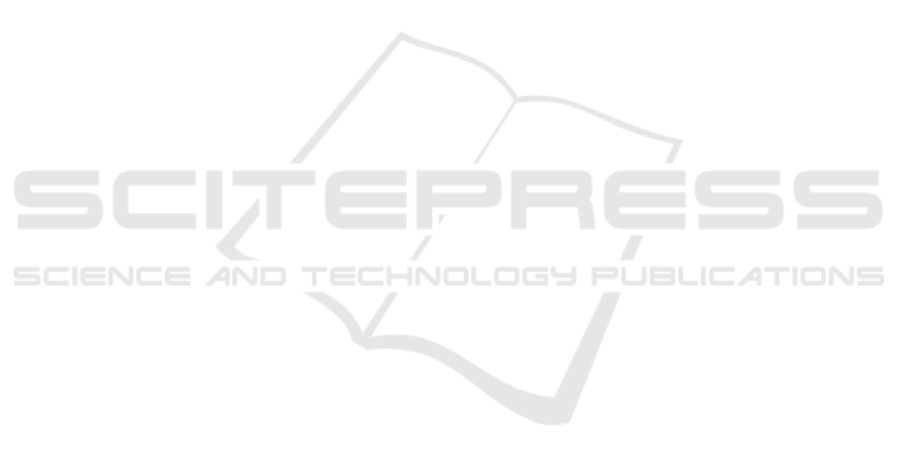
K.-S., Noy, N. F., Allemang, D., Lee, K.-I., Nixon,
L. J. B., Golbeck, J., Mika, P., Maynard, D., Mizogu-
chi, R., Schreiber, G., and Cudr-Mauroux, P., editors,
The Semantic Web, volume 4825 of Lecture Notes in
Computer Science, pages 58–71. Springer.
Bloem, P., Wibisono, A., and de Vries, G. (2014). Simpli-
fying RDF data for graph-based machine learning. In
KNOW@LOD, volume 1243 of CEUR Workshop Pro-
ceedings. CEUR-WS.org.
Colucci, S., Giannini, S., Donini, F. M., and Sciascio, E. D.
(2014). A deductive approach to the identification
and description of clusters in linked open data. In
Proceedings of the Twenty-first European Conference
on Artificial Intelligence, ECAI’14, pages 987–988,
Amsterdam, The Netherlands, The Netherlands. IOS
Press.
de Vries, G. K. (2013). A fast approximation of the
weisfeiler-lehman graph kernel for rdf data. In Pro-
ceedings of the European Conference on Machine Le-
arning and Knowledge Discovery in Databases - Vo-
lume 8188, ECML PKDD 2013, pages 606–621, New
York, NY, USA. Springer-Verlag New York, Inc.
de Vries, G. K. D. and de Rooij, S. (2015). Substructure
counting graph kernels for machine learning from rdf
data. Web Semant., 35(P2):71–84.
Grimnes, G. A., Edwards, P., and Preece, A. (2008). In-
stance based clustering of semantic web resources.
In Bechhofer, S., Hauswirth, M., Hoffmann, J., and
Koubarakis, M., editors, The Semantic Web: Research
and Applications, pages 303–317, Berlin, Heidelberg.
Springer Berlin Heidelberg.
Le, Q. and Mikolov, T. (2014). Distributed representations
of sentences and documents. In Proceedings of the
31st International Conference on International Con-
ference on Machine Learning - Volume 32, ICML’14,
pages II–1188–II–1196. JMLR.org.
L
¨
osch, U., Bloehdorn, S., and Rettinger, A. (2012). Graph
kernels for rdf data. In Simperl, E., Cimiano, P., Pol-
leres, A., Corcho, O., and Presutti, V., editors, The Se-
mantic Web: Research and Applications, pages 134–
148, Berlin, Heidelberg. Springer Berlin Heidelberg.
Manola, F. and Miller, E. (2004). RDF primer. World Wide
Web Consortium, Recommendation REC-rdf-primer-
20040210.
Mikolov, T., Yih, S. W.-t., and Zweig, G. (2013). Linguis-
tic regularities in continuous space word representa-
tions. In Proceedings of the 2013 Conference of the
North American Chapter of the Association for Com-
putational Linguistics: Human Language Technolo-
gies (NAACL-HLT-2013). Association for Computati-
onal Linguistics.
Ristoski, P. and Paulheim, H. (2016). Rdf2vec: Rdf graph
embeddings for data mining. In Groth, P., Simperl, E.,
Gray, A., Sabou, M., Kr
¨
otzsch, M., Lecue, F., Fl
¨
ock,
F., and Gil, Y., editors, The Semantic Web – ISWC
2016, pages 498–514, Cham. Springer International
Publishing.
Steinbach, M., Karypis, G., and Kumar, V. (2000). A com-
parison of document clustering techniques. In In KDD
Workshop on Text Mining.
Tran, T., Wang, H., and Haase, P. (2009). Hermes: Data web
search on a pay-as-you-go integration infrastructure.
Web Semant., 7(3):189–203.
Zhao, Y. and Karypis, G. (2002). Evaluation of hierarchical
clustering algorithms for document datasets. In Pro-
ceedings of the Eleventh International Conference on
Information and Knowledge Management, CIKM ’02,
pages 515–524, New York, NY, USA. ACM.
RDF Data Clustering based on Resource and Predicate Embeddings
373