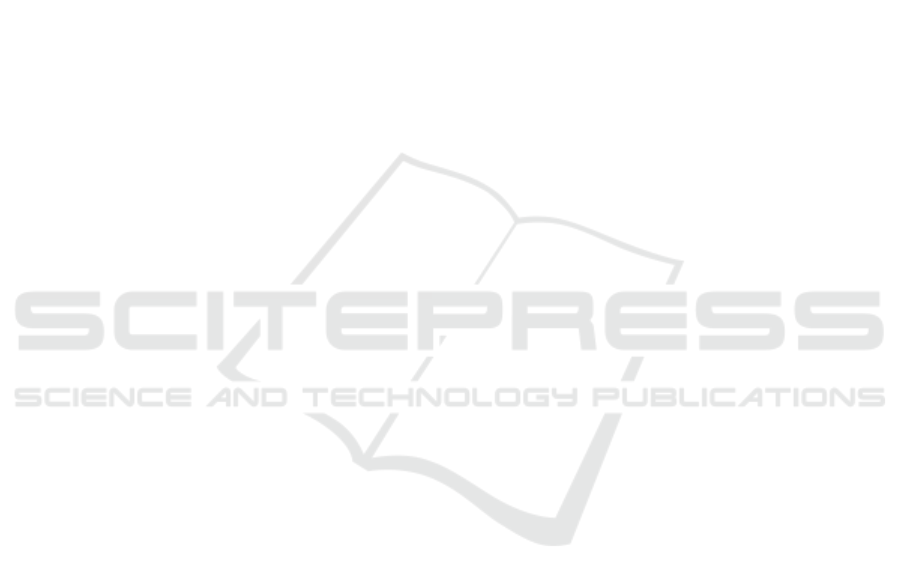
Statistical test aims to see whether there is a link
between the data TWI and API. The result of this
research is Kendall's Tau statistic test where the
researcher compares the category or class of Malaria
risk based on Topographic Wetness Index with
category or class of Annual Parasites Incidence and
0,146, sig = 0,010.
This result shows that there is correlation between
TWI and API.
4 DISCUSSION
The use of TWI data in predicting the risk of malaria
should be considered as an additional tool in the
program of malaria elimination in South Central
Timor District. This is in line to research conducted
by Cohen et al (2010) in which TWI data is better at
predicting malaria risk than land cover data and land
use data. The use of TWI data is also supported by
the technological development and the increasing
availability of free satellite imagery data by
providers. The availability of a free version of
software and application, has beneficial for
researchers to obtain spatial data (Fisher et al.,
2018).
Topography is a major factor in which moist or
wet areas are identified to have high vector densities.
Wet areas in the area is inundated inviting vector
Anopheles mosquito to breed. It will be even worse
if the area is a malaria endemic (Mwakalinga et al.,
2018). Malaria is strongly influenced by
environmental ecology. Furthermore, rapid people
movement increase the transmission of malaria.
The use of the TWI to predict malaria risk areas
is motivated by a shift in malaria cases that
previously occurred in many coastal areas, but now
the annual data of Parasites Incidence are also high
in mountainous areas. The large number of malaria
cases in mountainous areas, has challenged the
prediction of malaria risk areas that uses elevation
data of a region.
Mapping the malaria risk areas is very important
in the process of eliminating malaria. This mapping
is a basis for elimination activities in order to be
right on determining target. If we do not know about
malaria risk areas it will be difficult to carry out the
elimination activities, with this analysis, malaria
program managers in the district or provincial level
will be able to predict malaria risk areas and conduct
the activities in order to eliminate malaria
appropriately.
5 CONCLUSION
The result of this study shows that there are
correlation between TWI and API. The use of TWI
analysis results is very good for predicting areas at
risk of malaria. This is in line with the results of the
analysis that the TWI category is linked to the 2017
data API in 278 villages / sub-districts in South
Central Timor District. It needs to be done in-depth
analysis to see more other variables especially
location of malaria patient on risky area. The result
of this analysis can be a guide for Puskesmas and
Health Department to conduct a program of
elimination of malaria disease in South Central
Timor District.
REFERENCE
Central Berau of Statistic TTS District (2017) South
Central Timor in Number 2017. SoE.
Cohen, J. M. et al.
indices predict household malaria risk better than
land-use and land-cover in the western Kenya
Malaria Journal, 9(1), p. 328. doi:
10.1186/1475-2875-9-328.
Fisher, R. P. et al. satellite imagery and
digital elevation model analyses enabling natural
resource management in the developing world:
Singapore
Journal of Tropical Geography, 39(1), pp. 4561.
doi: 10.1111/sjtg.12210.
Haas, J. (2010)
(March), p. 103. Available at: http://www.diva-
portal.org/smash/record.jsf?pid=diva2:460111.
Health Department Republic Indonesia (1999) Modul
Epidemiologi Malaria. Edited by D. of C. A. S.
Disease.
Health Ministry Republic of Indonesia (2013) Basic
Health Research 2013. Jakarta.
Health Ministry Republic of Indonesia (2014) Malaria
guidelines management. Jakarta.
Mwakalinga, V. M. et al.
the interfaces between human and aquatic
mosquito habitats to enable barrier targeting of
Royal
Society Open Science, 5(5), p. 161055. doi:
10.1098/rsos.161055.
Nmor, J. C. et al.
predicting malaria vector breeding habitats:
Parasites and Vectors. Parasites & Vectors, 6(1),
p. 1. doi: 10.1186/1756-3305-6-14.
hillslope flow paths for distributed hydrological
Hydrological Processes, 5(1), pp. 5979. doi:
10.1002/hyp.3360050106.
Spatial Analysis of the correlation between Topographic Wetness Index with Annual Parasites Incidence Malaria in South Central Timor
District 2017 â
˘
A¸S Indonesia
379