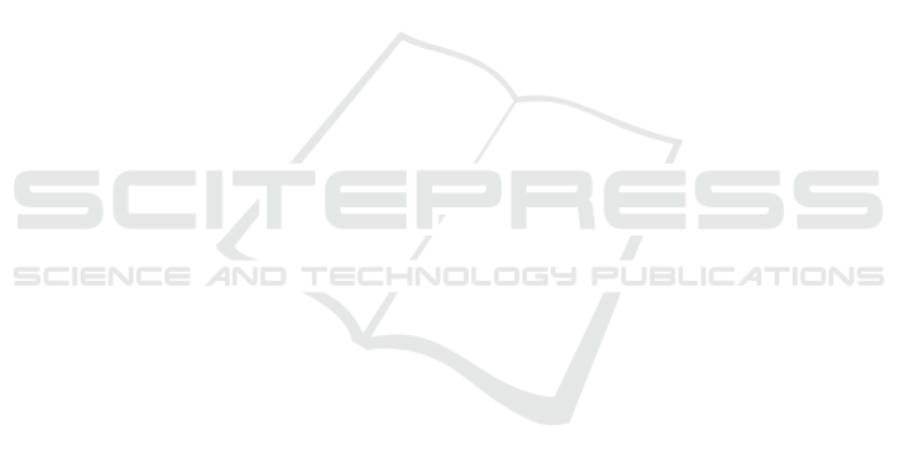
REFERENCES
Badrinarayanan, V., Kendall, A., and Cipolla, R. (2015).
Segnet: A deep convolutional encoder-decoder ar-
chitecture for image segmentation. arXiv preprint
arXiv:1511.00561.
Cordts, M., Omran, M., Ramos, S., Rehfeld, T., Enzwei-
ler, M., Benenson, R., Franke, U., Roth, S., and
Schiele, B. (2016). The cityscapes dataset for se-
mantic urban scene understanding. arXiv preprint
arXiv:1604.01685.
Drayer, B. and Brox, T. (2016). Object detection, tracking,
and motion segmentation for object-level video seg-
mentation. arXiv preprint arXiv:1608.03066.
Farabet, C., Couprie, C., Najman, L., and LeCun, Y.
(2013). Learning hierarchical features for scene labe-
ling. IEEE transactions on pattern analysis and ma-
chine intelligence, 35(8):1915–1929.
Fragkiadaki, K., Arbelaez, P., Felsen, P., and Malik, J.
(2015). Learning to segment moving objects in vi-
deos. In Proceedings of the IEEE Conference on Com-
puter Vision and Pattern Recognition, pages 4083–
4090.
Gadde, R., Jampani, V., and Gehler, P. V. (2017). Seman-
tic video cnns through representation warping. CoRR,
abs/1708.03088, 8:9.
Gaidon, A., Wang, Q., Cabon, Y., and Vig, E. (2016). Vir-
tual worlds as proxy for multi-object tracking analy-
sis. In CVPR.
Grangier, D., Bottou, L., and Collobert, R. (2009). Deep
convolutional networks for scene parsing. In ICML
2009 Deep Learning Workshop, volume 3. Citeseer.
Hazirbas, C., Ma, L., Domokos, C., and Cremers, D.
(2016). Fusenet: Incorporating depth into semantic
segmentation via fusion-based cnn architecture. In
Asian Conference on Computer Vision, pages 213–
228. Springer.
Horgan, J., Hughes, C., McDonald, J., and Yogamani, S.
(2015). Vision-based driver assistance systems: Sur-
vey, taxonomy and advances. In Intelligent Transpor-
tation Systems (ITSC), 2015 IEEE 18th International
Conference on, pages 2032–2039. IEEE.
Hur, J. and Roth, S. (2016). Joint optical flow and tem-
porally consistent semantic segmentation. In Euro-
pean Conference on Computer Vision, pages 163–177.
Springer.
Ilg, E., Mayer, N., Saikia, T., Keuper, M., Dosovitskiy, A.,
and Brox, T. (2016). Flownet 2.0: Evolution of optical
flow estimation with deep networks. arXiv preprint
arXiv:1612.01925.
Ilg, E., Mayer, N., Saikia, T., Keuper, M., Dosovitskiy, A.,
and Brox, T. (2017). Flownet 2.0: Evolution of op-
tical flow estimation with deep networks. In IEEE
conference on computer vision and pattern recogni-
tion (CVPR), volume 2, page 6.
Jain, S. D., Xiong, B., and Grauman, K. (2017). Fusion-
seg: Learning to combine motion and appearance for
fully automatic segmention of generic objects in vi-
deos. arXiv preprint arXiv:1701.05384.
Long, J., Shelhamer, E., and Darrell, T. (2015). Fully con-
volutional networks for semantic segmentation. In
Proceedings of the IEEE Conference on Computer Vi-
sion and Pattern Recognition, pages 3431–3440.
Menze, M. and Geiger, A. (2015). Object scene flow for au-
tonomous vehicles. In Proceedings of the IEEE Con-
ference on Computer Vision and Pattern Recognition,
pages 3061–3070.
Nilsson, D. and Sminchisescu, C. (2016). Semantic vi-
deo segmentation by gated recurrent flow propagation.
arXiv preprint arXiv:1612.08871, 2.
Noh, H., Hong, S., and Han, B. (2015). Learning deconvo-
lution network for semantic segmentation. In Procee-
dings of the IEEE International Conference on Com-
puter Vision, pages 1520–1528.
Ochs, P., Malik, J., and Brox, T. (2014). Segmentation of
moving objects by long term video analysis. IEEE
transactions on pattern analysis and machine intelli-
gence, 36(6):1187–1200.
Papazoglou, A. and Ferrari, V. (2013). Fast object segmen-
tation in unconstrained video. In Proceedings of the
IEEE International Conference on Computer Vision,
pages 1777–1784.
Sevilla-Lara, L., Sun, D., Jampani, V., and Black, M. J.
(2016). Optical flow with semantic segmentation and
localized layers. In Proceedings of the IEEE Confe-
rence on Computer Vision and Pattern Recognition,
pages 3889–3898.
Siam, M., Elkerdawy, S., Jagersand, M., and Yogamani, S.
(2017a). Deep semantic segmentation for automated
driving: Taxonomy, roadmap and challenges. arXiv
preprint arXiv:1707.02432.
Siam, M., Mahgoub, H., Zahran, M., Yogamani, S., Ja-
gersand, M., and El-Sallab, A. (2017b). Modnet:
Moving object detection network with motion and
appearance for autonomous driving. arXiv preprint
arXiv:1709.04821.
Simonyan, K. and Zisserman, A. (2014). Two-stream con-
volutional networks for action recognition in videos.
In Advances in neural information processing sys-
tems, pages 568–576.
Tokmakov, P., Alahari, K., and Schmid, C. (2016). Le-
arning motion patterns in videos. arXiv preprint
arXiv:1612.07217.
Torr, P. H. (1998). Geometric motion segmentation and mo-
del selection. Philosophical Transactions of the Royal
Society of London A: Mathematical, Physical and En-
gineering Sciences, 356(1740):1321–1340.
Vijayanarasimhan, S., Ricco, S., Schmid, C., Sukthankar,
R., and Fragkiadaki, K. (2017). Sfm-net: Learning
of structure and motion from video. arXiv preprint
arXiv:1704.07804.
Wehrwein, S. and Szeliski, R. (2017). Video segmentation
with background motion models. In BMVC.
VISAPP 2019 - 14th International Conference on Computer Vision Theory and Applications
172