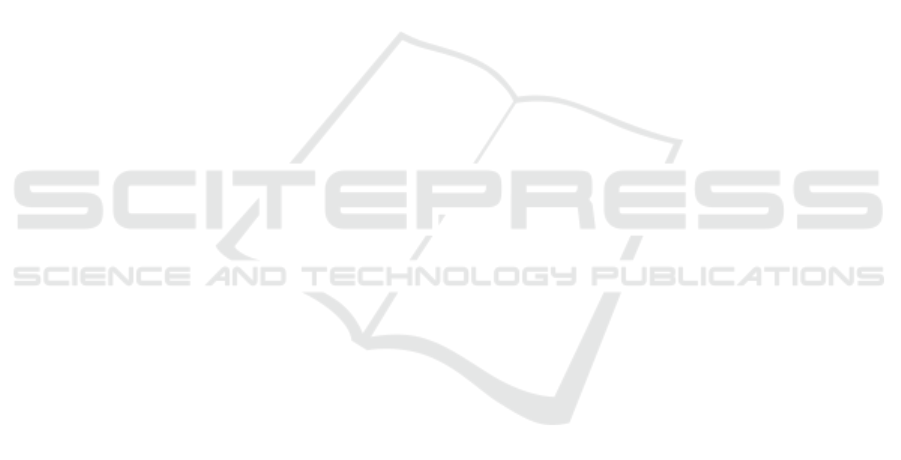
Li, L., Zheng, W., Zhang, Z., Huang, Y., and Wang, L.
(2018). Skeleton-based relational modeling for action
recognition. CoRR, abs/1805.02556.
Li, X., Adali, T., and Anderson, M. (2011). Noncircu-
lar principal component analysis and its application
to model selection. IEEE Transactions on Signal Pro-
cessing, 59(10):4516–4528.
Lokannavar, S., Lahane, P., Gangurde, A., and Chidre, P.
(2015a). Emotion recognition using eeg signals. Inter-
national Journal of Advanced Research in Computer
and Communication Engineering, 4(5):54–56.
Lokannavar, S., Lahane, P., Gangurde, A., and Chidre, P.
(2015b). Emotion recognition using eeg signals. Emo-
tion, 4(5):54–56.
Lucey, P., Cohn, J., Kanade, T., Saragih, J., Ambadar, Z.,
and Matthews, I. (2010). The extended cohn-kanade
dataset (ck+): A complete dataset for action unit and
emotion-specified expression. In Computer Vision and
Pattern Recognition Workshops (CVPRW), 2010 IEEE
Computer Society Conference on, pages 94–101.
McKeown, G., Valstar, M., Cowie, R., Pantic, M., and
Schroder, M. (2012). The semaine database: Anno-
tated multimodal records of emotionally colored con-
versations between a person and a limited agent. IEEE
Transactions on Affective Computing, 3(1):5–17.
Michel, P. and Kaliouby, R. E. (2003). Real time facial
expression recognition in video using support vector
machines. In Proceedings of the 5th international con-
ference on Multimodal interfaces, pages 258–264.
Montenegro, J., Gkelias, A., and Argyriou, V. (2016). Emo-
tion understanding using multimodal information ba-
sed on autobiographical memories for alzheimers pa-
tients. ACCVW, pages 252–268.
Montenegro, J. M. F. and Argyriou, V. (2017). Cognitive
evaluation for the diagnosis of alzheimer’s disease ba-
sed on turing test and virtual environments. Physio-
logy and Behavior, 173:42 – 51.
Ngo, A. L., Oh, Y., Phan, R., and See, J. (2016). Eulerian
emotion magnification for subtle expression recogni-
tion. ICASSP, pages 1243–1247.
Nicolaou, M. A., Gunes, H., and Pantic, M. (2011). Conti-
nuous prediction of spontaneous affect from multiple
cues and modalities in valence-arousal space. IEEE
Transactions on Affective Computing, 2(2):92–105.
Nicolle, J., Rapp, V., Bailly, K., Prevost, L., and Chetouani,
M. (2012). Robust continuous prediction of human
emotions using multiscale dynamic cues. 14th ACM
conf on Multimodal interaction, pages 501–508.
Pantic, M., Valstar, M., Rademaker, R., and Maat, L.
(2005). Web-based database for facial expression ana-
lysis. IEEE international conference on multimedia
and Expo, pages 317–321.
Park, S., Lee, S., and Ro, Y. (2015). Subtle facial expression
recognition using adaptive magnification of discrimi-
native facial motion. 23rd ACM international confe-
rence on Multimedia, pages 911–914.
Sariyanidi, E., Gunes, H., Gkmen, M., and Cavallaro, A.
(2013). Local zernike moment representation for fa-
cial affect recognition. British Machine Vision Conf.
Soleymani, M., Asghari-Esfeden, S., Fu, Y., and Pantic, M.
(2016). Analysis of eeg signals and facial expressions
for continuous emotion detection. IEEE Transactions
on Affective Computing, 7(1):17–28.
Soleymani, M., Lichtenauer, J., Pun, T., and Pantic, M.
(2012). A multimodal database for affect recognition
and implicit tagging. IEEE Transactions on Affective
Computing, 3(1):42–55.
Song, Y., McDuff, D., Vasisht, D., and Kapoor, A. (2015).
Exploiting sparsity and co-occurrence structure for
action unit recognition. In Automatic Face and Ge-
sture Recognition (FG), 2015 11th IEEE International
Conference and Workshops on, 1:1–8.
Valstar, M., Snchez-Lozano, E., Cohn, J., Jeni, L., Girard,
J., Zhang, Z., Yin, L., and Pantic, M. (2017). Fera
2017: Addressing head pose in the third facial expres-
sion recognition and analysis challenge. arXiv pre-
print arXiv:1702.04174, 19(1-12):888–896.
Wadhwa, N., Wu, H., Davis, A., Rubinstein, M., Shih, E.,
Mysore, G., Chen, J., Buyukozturk, O., Guttag, J.,
Freeman, W., and Durand, F. (2016). Eulerian video
magnification and analysis. Communications of the
ACM, 60(1):87–95.
Weninger, F., Wllmer, M., and Schuller, B. (2015). Emo-
tion recognition in naturalistic speech and language
survey. Emotion Recognition: A Pattern Analysis Ap-
proach, pages 237–267.
Wu, H., Rubinstein, M., Shih, E., Guttag, J., Durand, F.,
and Freeman, W. (2012). Eulerian video magnifica-
tion for revealing subtle changes in the world. ACM
Transactions on Graphics, 31:1–8.
Yan, H. (2017). Collaborative discriminative multi-metric
learning for facial expression recognition in video.
Pattern Recognition.
Yan, S., Xiong, Y., and Lin, D. (2018). Spatial tempo-
ral graph convolutional networks for skeleton-based
action recognition. CoRR, abs/1801.07455.
Yan, W. J., Li, X., Wang, S. J., Zhao, G., Liu, Y. J., Chen,
Y. H., and Fu, X. (2014). Casme ii: An improved
spontaneous micro-expression database and the base-
line evaluation. PloS one, 9(1):e86041.
Yi, J., Mao, X., Xue, Y., and Compare, A. (2013). Facial ex-
pression recognition based on t-sne and adaboostm2.
GreenCom, pages 1744–1749.
Zeng, Z., Pantic, M., Roisman, G. I., and Huang, T. S.
(2009). A survey of affect recognition methods: Au-
dio, visual, and spontaneous expressions. PAMI,
31(1):39–58.
Zhao, G. and Pietikinen, M. (2009). Boosted multi-
resolution spatiotemporal descriptors for facial ex-
pression recognition. Pattern recognition letters,
30(12):1117–1127.
VISAPP 2019 - 14th International Conference on Computer Vision Theory and Applications
302