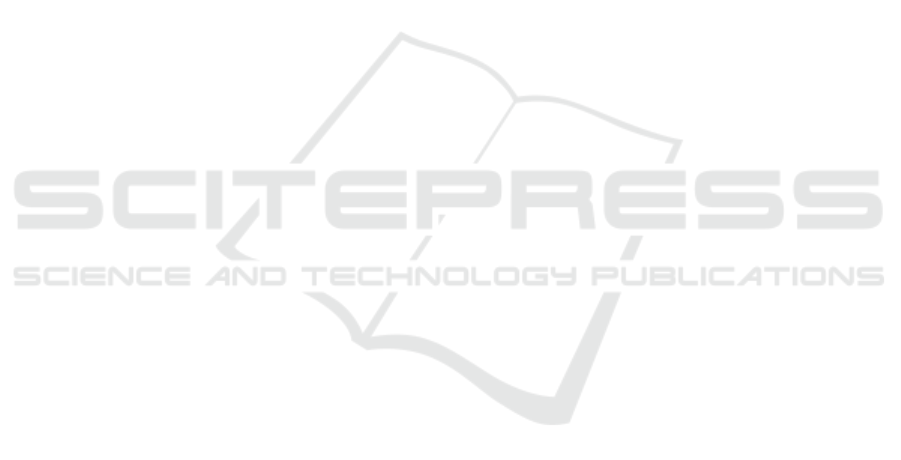
REFERENCES
Bay, H., Tuytelaars, T., and Van Gool, L. (2006). Surf:
Speeded up robust features. In European conference
on computer vision, pages 404–417. Springer.
Fei-Fei, L. and Perona, P. (2005). A bayesian hierarchical
model for learning natural scene categories. In Com-
puter Vision and Pattern Recognition, 2005. CVPR
2005. IEEE Computer Society Conference on, vo-
lume 2, pages 524–531. IEEE.
Gan, C., Wang, N., Yang, Y., Yeung, D.-Y., and Hauptmann,
A. G. (2015). Devnet: A deep event network for mul-
timedia event detection and evidence recounting. In
Proc. of the IEEE Conference on Computer Vision and
Pattern Recognition, pages 2568–2577.
Gao, Z., Chen, M.-Y., Hauptmann, A. G., and Cai, A.
(2010). Comparing evaluation protocols on the kth
dataset. In International Workshop on Human Beha-
vior Understanding, pages 88–100. Springer.
Hou, Y., Li, Z., Wang, P., and Li, W. (2018). Skeleton op-
tical spectra-based action recognition using convoluti-
onal neural networks. IEEE Transactions on Circuits
and Systems for Video Technology, 28(3):807–811.
Ji, S., Xu, W., Yang, M., and Yu, K. (2013). 3d convolu-
tional neural networks for human action recognition.
IEEE Transactions on PAMI, 35(1):221–231.
Klaser, A., Marszałek, M., and Schmid, C. (2008). A spatio-
temporal descriptor based on 3d-gradients. In BMVC
2008-19th British Machine Vision Conference, pages
275–1. British Machine Vision Association.
Koppula, H. S., Gupta, R., and Saxena, A. (2013). Learning
human activities and object affordances from rgb-d vi-
deos. The International Journal of Robotics Research,
32(8):951–970.
Krizhevsky A, Sutskever I, H. G. (2012). Imagenet classifi-
cation with deep convolutional neural networks. pages
1097–1105. In Advances in neural information pro-
cessing systems.
Kuehne, H., Jhuang, H., Garrote, E., Poggio, T., and Serre,
T. (2011). Hmdb: a large video database for human
motion recognition. In Computer Vision (ICCV), 2011
IEEE International Conference on, pages 2556–2563.
Laptev, I. (2005). On space-time interest points. Internati-
onal journal of computer vision, 64(2-3):107–123.
Laptev, I., Marszalek, M., Schmid, C., and Rozenfeld, B.
(2008). Learning realistic human actions from mo-
vies. In Computer Vision and Pattern Recognition,
2008. CVPR 2008. IEEE Conference on, pages 1–8.
Le, Q. V., Zou, W. Y., Yeung, S. Y., and Ng, A. Y. (2011).
Learning hierarchical invariant spatio-temporal featu-
res for action recognition with independent subspace
analysis. In Computer Vision and Pattern Recogni-
tion (CVPR), 2011 IEEE Conference on, pages 3361–
3368. IEEE.
LeCun, Y., Bottou, L., Bengio, Y., and Haffner, P. (1998).
Gradient-based learning applied to document recogni-
tion. Proceedings of the IEEE, 86(11):2278–2324.
Ni, B., Pei, Y., Liang, Z., Lin, L., and Moulin, P. (2013).
Integrating multi-stage depth-induced contextual in-
formation for human action recognition and locali-
zation. In Automatic Face and Gesture Recognition
(FG), 2013 10th IEEE International Conference and
Workshops on, pages 1–8. IEEE.
Niebles, J. C., Chen, C.-W., and Fei-Fei, L. (2010). Mo-
deling temporal structure of decomposable motion
segments for activity classification. In European con-
ference on computer vision, pages 392–405. Springer.
Rahmani, H., Mian, A., and Shah, M. (2018). Learning
a deep model for human action recognition from no-
vel viewpoints. IEEE transactions on pattern analysis
and machine intelligence, 40(3):667–681.
Scovanner, P., Ali, S., and Shah, M. (2007). A 3-
dimensional sift descriptor and its application to
action recognition. In Proceedings of the 15th ACM
international conference on Multimedia, pages 357–
360. ACM.
Simonyan, K. and Zisserman, A. (2014). Two-stream
convolutional networks for action recognition in vi-
deos. In Ghahramani, Z., Welling, M., Cortes, C.,
Lawrence, N. D., and Weinberger, K. Q., editors, Ad-
vances in Neural Information Processing Systems 27,
pages 568–576. Curran Associates, Inc.
Soomro, K., Zamir, A. R., and Shah, M. (2012). Ucf101:
A dataset of 101 human actions classes from videos in
the wild. arXiv preprint arXiv:1212.0402.
Sung, J., Ponce, C., Selman, B., and Saxena, A. (2012a).
Unstructured human activity detection from rgbd ima-
ges. In Robotics and Automation (ICRA), 2012 IEEE
International Conference on, pages 842–849. IEEE.
Sung, J., Ponce, C., Selman, B., and Saxena, A. (2012b).
Unstructured human activity detection from rgbd ima-
ges. In 2012 IEEE International Conference on Robo-
tics and Automation, pages 842–849.
Wang, H., Kl
¨
aser, A., Schmid, C., and Liu, C.-L. (2013).
Dense trajectories and motion boundary descriptors
for action recognition. International journal of com-
puter vision, 103(1):60–79.
Wang, H. and Schmid, C. (2013). Action recognition with
improved trajectories. In IEEE international confe-
rence on computer vision, pages 3551–3558.
Wang, K., Wang, X., Lin, L., Wang, M., and Zuo, W.
(2014). 3d human activity recognition with reconfigu-
rable convolutional neural networks. In Proceedings
of the 22Nd ACM International Conference on Multi-
media, MM ’14, pages 97–106, New York, NY, USA.
Wang, L., Qiao, Y., and Tang, X. (2015). Action recog-
nition with trajectory-pooled deep-convolutional des-
criptors. In IEEE Conference on computer vision and
pattern recognition, pages 4305–4314.
Wang, L., Xiong, Y., Wang, Z., Qiao, Y., Lin, D., Tang, X.,
and Van Gool, L. (2016). Temporal segment networks:
Towards good practices for deep action recognition.
In Leibe, B., Matas, J., Sebe, N., and Welling, M.,
editors, Computer Vision – ECCV 2016, pages 20–36,
Cham. Springer International Publishing.
Wu, P., Hoi, S. C., Xia, H., Zhao, P., Wang, D., and Miao,
C. (2013). Online multimodal deep similarity learning
with application to image retrieval. In Proceedings of
the 21st ACM international conference on Multime-
dia, pages 153–162.
VISAPP 2019 - 14th International Conference on Computer Vision Theory and Applications
198