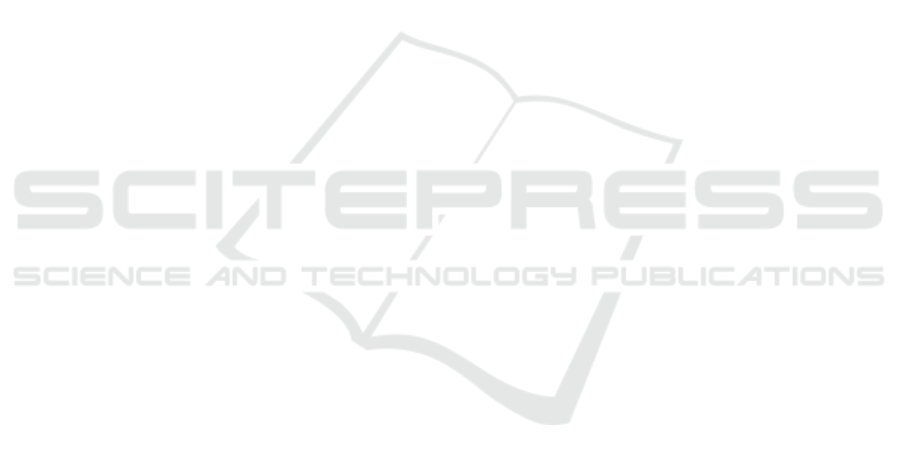
IEEE Conference on Computer Vision and Pattern Re-
cognition, volume 1, pages 886–893. IEEE.
Dell’Acqua, F. and Gamba, P. (2012). Remote sensing
and earthquake damage assessment: Experiences, li-
mits, and perspectives. Proceedings of the IEEE,
100(10):2876–2890.
Fujita, A., Sakurada, K., Imaizumi, T., Ito, R., Hikosaka,
S., and Nakamura, R. (2017). Damage detection from
aerial images via convolutional neural networks. In
Machine Vision Applications (MVA), 2017 Fifteenth
IAPR International Conference on, pages 5–8. IEEE.
Gamba, P., Dell’Acqua, F., and Odasso, L. (2007). Object-
oriented building damage analysis in VHR optical sa-
tellite images of the 2004 tsunami over Kalutara, Sri
Lanka. In Urban Remote Sensing Joint Event, 2007,
pages 1–5. IEEE.
Genuer, R., Poggi, J.-M., and Tuleau-Malot, C. (2010). Va-
riable selection using random forests. Pattern Recog-
nition Letters, 31(14):2225–2236.
Gerke, M. and Kerle, N. (2011). Automatic structu-
ral seismic damage assessment with airborne oblique
pictometry
c
imagery. Photogrammetric Engineering
& Remote Sensing, 77(9):885–898.
Geusebroek, J.-M., Van den Boomgaard, R., Smeulders, A.
W. M., and Geerts, H. (2001). Color invariance. IEEE
Transactions on Pattern Analysis and Machine Intel-
ligence, 23(12):1338–1350.
Gueguen, L. and Hamid, R. (2015). Large-scale damage
detection using satellite imagery. In Proceedings of
the IEEE Conference on Computer Vision and Pattern
Recognition, pages 1321–1328.
Huyck, C. K., Adams, B. J., Cho, S., Chung, H.-C., and
Eguchi, R. T. (2005). Towards rapid citywide damage
mapping using neighborhood edge dissimilarities in
very high-resolution optical satellite imagery Appli-
cation to the 2003 Bam, Iran, earthquake. Earthquake
Spectra, 21(S1):255–266.
Im, J., Jensen, J., and Tullis, J. (2008). Object-based change
detection using correlation image analysis and image
segmentation. International Journal of Remote Sen-
sing, 29(2):399–423.
Jiang, L., Lu, H., My, V. D., Koch, A., and Zell, A.
(2015). Superpixel segmentation based gradient maps
on RGB-D dataset. In IEEE International Conference
on Robotics and Biomimetics (ROBIO), pages 1359–
1364. IEEE.
Lagarias, J. C., Reeds, J. A., Wright, M. H., and Wright,
P. E. (1998). Convergence properties of the Nelder–
Mead Simplex method in low dimensions. SIAM Jour-
nal on Optimization, 9(1):112–147.
Ma, J. and Qin, S. (2012). Automatic depicting algorithm
of earthquake collapsed buildings with airborne high
resolution image. In Geoscience and Remote Sensing
Symposium (IGARSS), 2012 IEEE International, pa-
ges 939–942. IEEE.
Maggiori, E., Tarabalka, Y., Charpiat, G., and Alliez, P.
(2016). Fully convolutional neural networks for re-
mote sensing image classification. In Geoscience and
Remote Sensing Symposium (IGARSS), 2016 IEEE In-
ternational, pages 5071–5074. IEEE.
Pesaresi, M., Gerhardinger, A., and Haag, F. (2007). Rapid
damage assessment of built-up structures using VHR
satellite data in tsunami-affected areas. International
Journal of Remote Sensing, 28(13-14):3013–3036.
Pohl, M., Meidow, J., and Bulatov, D. (2017). Simplifica-
tion of polygonal chains by enforcing few distinctive
edge directions. Lecture Notes in Computer Science
(including subseries Lecture Notes in Artificial Intel-
ligence and Lecture Notes in Bioinformatics), 10270
LNCS:3–14.
Rasika, A., Kerle, N., and Heuel, S. (2006). Multi-scale
texture and color segmentation of oblique airborne vi-
deo data for damage classification. In Proceedings
of ISPRS Midterm Symposium 2006 Remote Sensing:
From Pixels to Processes, pages 08–11.
Rathje, E. M., Woo, K.-S., Crawford, M., and Neuen-
schwander, A. (2005). Earthquake damage identifica-
tion using multi-temporal high-resolution optical sa-
tellite imagery. In Proceedings of the IEEE on Ge-
oscience and Remote Sensing Symposium, volume 7,
pages 5045–5048. IEEE.
Sirmacek, B. and Unsalan, C. (2009). Damaged building
detection in aerial images using shadow information.
In 4th International Conference on Recent Advances
in Space Technologies, pages 249–252. IEEE.
Thomas, J., Kareem, A., and Bowyer, K. W. (2014). Au-
tomated poststorm damage classification of low-rise
building roofing systems using high-resolution aerial
imagery. IEEE Transactions on Geoscience and Re-
mote Sensing, 52(7):3851–3861.
Tomowski, D., Klonus, S., Ehlers, M., Michel, U., and
Reinartz, P. (2010). Change visualization through a
texture-based analysis approach for disaster applicati-
ons. In ISPRS Proceedings on Advanced Remote Sen-
sing Science, volume XXXVIII, pages 263–269.
Tu, J., Li, D., Feng, W., Han, Q., and Sui, H. (2017). De-
tecting damaged building regions based on semantic
scene change from multi-temporal high-resolution re-
mote sensing images. ISPRS International Journal of
Geo-Information, 6(5):131.
Varma, M. and Zisserman, A. (2005). A statistical approach
to texture classification from single images. Internati-
onal Journal of Computer Vision, 62(1-2):61–81.
Veksler, O., Boykov, Y., and Mehrani, P. (2010). Super-
pixels and supervoxels in an energy optimization fra-
mework. Proceeding on European Conference on
Computer Vision, pages 211–224.
Vetrivel, A., Gerke, M., Kerle, N., Nex, F., and Vosselman,
G. (2017). Disaster damage detection through syner-
gistic use of deep learning and 3D point cloud features
derived from very high resolution oblique aerial ima-
ges, and multiple-kernel-learning. ISPRS Journal of
Photogrammetry and Remote Sensing.
Warnke, S. and Bulatov, D. (2017). Variable selection
for road segmentation in aerial images. International
Archives of the Photogrammetry, Remote Sensing &
Spatial Information Sciences, 42.
Zhang, J.-F., Xie, L.-L., and Tao, X.-X. (2002). Change
detection of remote sensing image for earthquake-
damaged buildings and its application in seismic di-
saster assessment. Journal of Natural Disasters,
11(2):59–64.
VISAPP 2019 - 14th International Conference on Computer Vision Theory and Applications
220