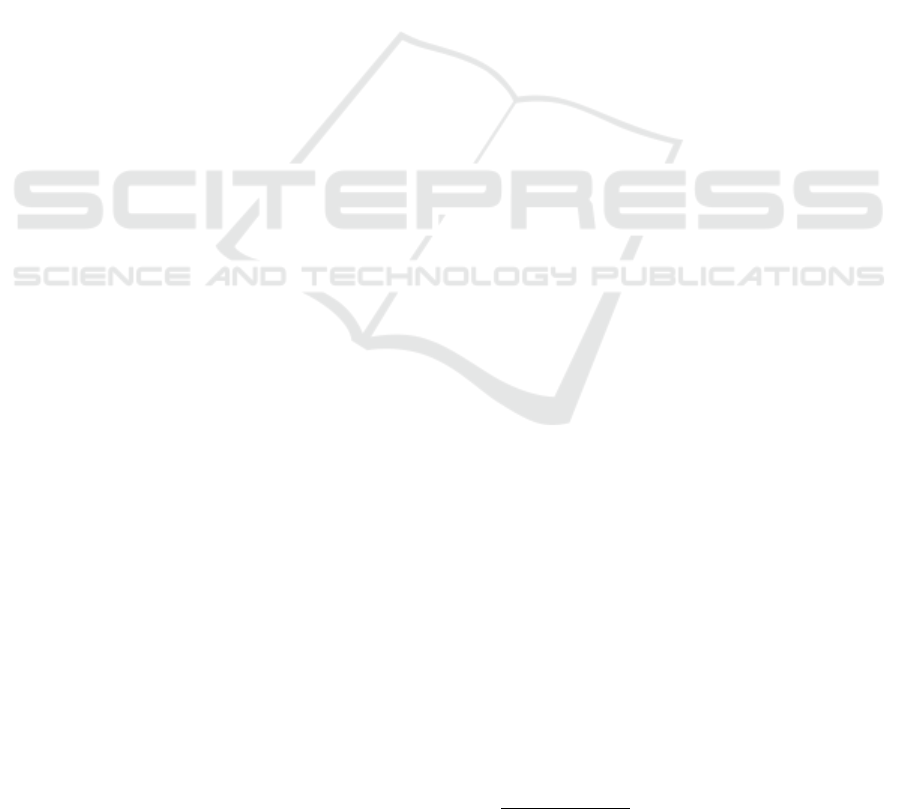
results which point out new visualization subjects. For
example, more visualization studies are required
focusing on printer usages and mail servers.
Further quantitative analysis results provide
information which requires to be deeply examined to
improve existing security visualization designs and to
form novel design. For example, sets of infrastructure
items which may be examined in groups in security
visualization solutions, the clusters of threats and
associated data sources and data attributes may point to
new metrics for particular threats.
Majority of the results helped in distinguishing
items among alternatives, or helped to understand new
issues. A few of the results did not allow sharp
distinctions among alternatives. During the scaling,
multiplier sets(coefficients) (one to five) were used as
mentioned before. Using a different multiplier set
would end up with having more clear boundaries.
The authors think that doing this kind of a survey
may result in user-centric solutions with better designs.
In this way, the designers can find out novel ideas
which may contribute to creating holistic approaches
for the enterprise security. These results should be
reflected to the security visualization domain by novel
designs which are not restricted to known data sources
and known use-cases.
This survey may also be suitable for carrying out
internally in the organizations. It may also be adapted
for non-technical people. This effort may lead to other
interesting results, such as the identification of new
security sources, and new visualization use-cases.
One major limitation of this study was the limited
number of attendees. They were all informed about the
content of the survey prior to their participation,
especially on the type of the questions, and the length
of the survey. Some participants hesitated to contribute
due to the length of the survey and some others
hesitated due to the specific subject of the survey. A
shorter survey involving similar concepts can be
prepared as a future work, and new ways of survey
distribution can be considered in order to get the
maximum benefit. Another limitation is, as explained
before although literature learnings influenced the
survey design, the length limitations did not allow to
explicitly demonstrate all these influences. Using an
ordered coefficient set corresponding to Likert scale
results during the scaling of some data elements may
be considered as some type of limitation, cause another
set may slightly effect the results as mentioned earlier.
This paper has shown that users are not familiar
with the majority of security visualization solutions or
have problems in using existing security visualization
solutions. More effort should be given to designing
user-focused security visualization designs.
The results were recorded to be converted to
functional and non-functional requirements as a future
work. The requirements should also be elicited
accordingly and should be combined with the latest
technological instruments to form an enterprise
security visualization system design as a further future
work.
REFERENCES
Agarwal, Rakesh, and Ramakrishnan Srikant. 1994. “Fast
algorithms for mining association rules.” Proceedings
of the 20th Very Large Data Bases Conference.
Burlington, MA, USA: Morgan Kaufmann. 487-499.
Frincke, Deborah A., Carrie E. Gates, and John R. Goodall.
2009. “Message from the Workshop Chairs.” 6th
International Workshop on Visualization for Cyber
Security. Atlanta, GA, USA: IEEE. iv-v.
Agarwal, R. and Srikant, R., 1994. Fast algorithms for
mining association rules. Burlington, MA, USA,
Morgan Kaufmann, pp. 487-499.
Frincke, D. A., Gates, C. E. and Goodall, J. R., 2009.
Message from the Workshop Chairs. Atlanta, GA,
USA, IEEE, pp. iv-v.
Fry, B., 2007. Visualizing data: exploring and explaining
data with the Processing environment. s.l.:O'Reilly
Media, Inc.
Halleen, G. and Kellogg, G., 2007. Security monitoring
with cisco security mars. Boston, MA,USA: Pearson
Education.
Hall, M. et al., 2009. The WEKA data mining software: an
update. 11(1), pp. 10-18.
Josephsen, D., 2007. Building a monitoring infrastructure
with Nagios. Upper Saddle River, NJ, USA: Prentice
Hall.
Koike, H. and Ohno, K., 2004. SnortView: visualization
system of snort logs.. s.l., ACM, pp. 143-147.
Liao, Q., Blaich, A., Striegel, A. and Thain, D., 2008.
ENAVis: Enterprise Network Activities Visualization.
s.l., s.n., pp. 59-74.
Özdemir Sönmez, F. and Günel, B., 2018. Security
Visualization Extended Review Issues, Classifications,
Validation Methods, Trends, Extensions. In: Security
and Privacy Management, Techniques, and Protocols.
s.l.:IGI Global, pp. 152-197.
Sec Viz, 2018. SecViz Security Visualization. [Online]
Available at: https://secviz.org/[Accessed 8 9 2018].
Shiravi, H., Shiravi, A. and Ghorbani, A. A., 2012. A
Survey of Visualization Systems for Network Security.
IEEE Transactions on Visualization and Computer
Graphics, 18(8), pp. 1313 - 1329.
Staheli, D. et al., 2014. Visualization evaluation for cyber
security: trends and future directions. Paris, France,
ACM, pp. 49-56.
Vis Sec, 2018. IEEE Symposium on Visualization for Cyber
Security. [Online] Available at:
https://vizsec.org/[Accessed 10 9 2018].
IVAPP 2019 - 10th International Conference on Information Visualization Theory and Applications
182