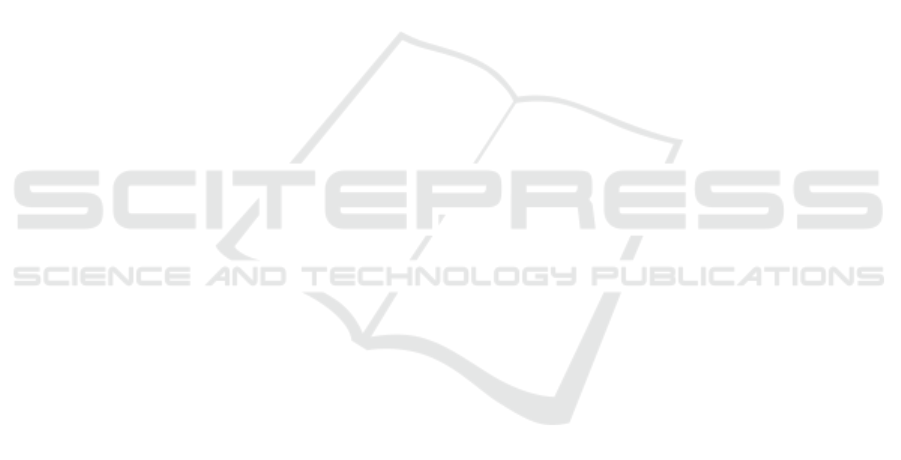
REFERENCES
Afifi, M. and Abdelhamed, A. (2017). Afif4: Deep gender
classification based on adaboost-based fusion of iso-
lated facial features and foggy faces. arXiv preprint
arXiv:1706.04277.
Agustsson, E., Timofte, R., Escalera, S., Baro, X., Guyon,
I., and Rothe, R. (2017). Apparent and real age esti-
mation in still images with deep residual regressors on
appa-real database. In Automatic Face & Gesture Re-
cognition (FG 2017), 2017 12th IEEE International
Conference on, pages 87–94. IEEE.
Antipov, G., Baccouche, M., Berrani, S.-A., and Dugelay,
J.-L. (2016). Apparent age estimation from face ima-
ges combining general and children-specialized deep
learning models. In Proceedings of the IEEE con-
ference on computer vision and pattern recognition
workshops, pages 96–104.
Biemann, C. (2006). Chinese whispers: an efficient graph
clustering algorithm and its application to natural lan-
guage processing problems. In Proceedings of the first
workshop on graph based methods for natural lan-
guage processing, pages 73–80. Association for Com-
putational Linguistics.
Bulat, A. and Tzimiropoulos, G. (2017). How far are we
from solving the 2d & 3d face alignment problem?
(and a dataset of 230,000 3d facial landmarks). In
International Conference on Computer Vision.
Cao, Q., Shen, L., Xie, W., Parkhi, O. M., and Zisserman,
A. (2018). Vggface2: A dataset for recognising faces
across pose and age. In Automatic Face & Gesture
Recognition (FG 2018), 2018 13th IEEE International
Conference on, pages 67–74. IEEE.
Chen, B.-C., Chen, C.-S., and Hsu, W. H. (2014). Cross-
age reference coding for age-invariant face recogni-
tion and retrieval. In European conference on compu-
ter vision, pages 768–783. Springer.
Cheng, Y. (1995). Mean shift, mode seeking, and clustering.
IEEE transactions on pattern analysis and machine
intelligence, 17(8):790–799.
Frey, B. J. and Dueck, D. (2007). Clustering by
passing messages between data points. science,
315(5814):972–976.
Golomb, B. A., Lawrence, D. T., and Sejnowski, T. J.
(1990). Sexnet: A neural network identifies sex from
human faces. In NIPS, volume 1, page 2.
Han, H., Otto, C., Jain, A. K., et al. (2013). Age estimation
from face images: Human vs. machine performance.
ICB, 13:1–8.
He, K., Zhang, X., Ren, S., and Sun, J. (2015). Delving
deep into rectifiers: Surpassing human-level perfor-
mance on imagenet classification. In Proceedings of
the IEEE international conference on computer vi-
sion, pages 1026–1034.
He, K., Zhang, X., Ren, S., and Sun, J. (2016). Deep resi-
dual learning for image recognition. In Proceedings of
the IEEE conference on computer vision and pattern
recognition, pages 770–778.
Hu, Z., Wen, Y., Wang, J., Wang, M., Hong, R., and Yan,
S. (2017). Facial age estimation with age difference.
IEEE Transactions on Image Processing, 26(7):3087–
3097.
Huang, G., Mattar, M., Lee, H., and Learned-Miller, E. G.
(2012). Learning to align from scratch. In Advances
in neural information processing systems, pages 764–
772.
Huang, G. B., Mattar, M., Berg, T., and Learned-Miller, E.
(2008). Labeled faces in the wild: A database forstu-
dying face recognition in unconstrained environments.
In Workshop on faces in’Real-Life’Images: detection,
alignment, and recognition.
Jia, S. and Cristianini, N. (2015). Learning to classify gen-
der from four million images. Pattern recognition let-
ters, 58:35–41.
Ng, H.-W. and Winkler, S. (2014). A data-driven approach
to cleaning large face datasets. In Image Processing
(ICIP), 2014 IEEE International Conference on, pa-
ges 343–347. IEEE.
Ni, B., Song, Z., and Yan, S. (2009). Web image mining
towards universal age estimator. In Proceedings of
the 17th ACM international conference on Multime-
dia, pages 85–94. ACM.
Panis, G. and Lanitis, A. (2014). An overview of rese-
arch activities in facial age estimation using the fg-net
aging database. In European Conference on Computer
Vision, pages 737–750. Springer.
Parkhi, O. M., Vedaldi, A., Zisserman, A., et al. (2015).
Deep face recognition. In BMVC, volume 1, page 6.
Ricanek, K. and Tesafaye, T. (2006). Morph: A longitudinal
image database of normal adult age-progression. In
Automatic Face and Gesture Recognition, 2006. FGR
2006. 7th International Conference on, pages 341–
345. IEEE.
Rothe, R., Timofte, R., and Van Gool, L. (2016). Deep ex-
pectation of real and apparent age from a single image
without facial landmarks. International Journal of
Computer Vision, pages 1–14.
Sun, Y., Wang, X., and Tang, X. (2015). Deeply learned
face representations are sparse, selective, and robust.
In Proceedings of the IEEE conference on computer
vision and pattern recognition, pages 2892–2900.
Zeiler, M. D. (2012). Adadelta: an adaptive learning rate
method. arXiv preprint arXiv:1212.5701.
Unsupervised Facial Biometric Data Filtering for Age and Gender Estimation
217