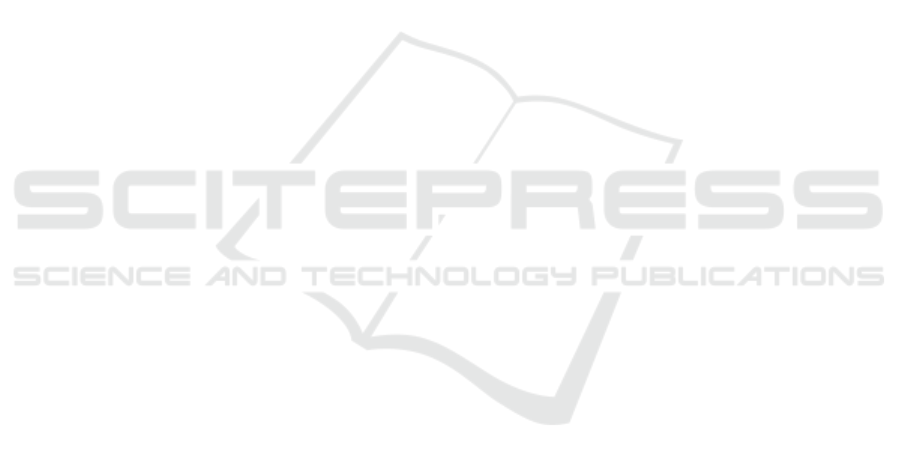
The methods used in this paper are not limited to
predicting the outcomes of the malaria vaccine—they
can also be used to predict the outcomes for any vac-
cine for which the gene expression data are available
in the HG-U133 Plus2.0 format. The feature selection
scheme (Section 2.2) can be used to find the gene ex-
pression attributes that are better correlated with the
target variable for any particular vaccine for which
predictions are to be made. Along with feature se-
lection schemes, non-linear models such as MLP can
be used where appropriate, to capture complex rela-
tionships (in case of some other diseases) between the
gene expression data and the vaccine outcomes.
REFERENCES
Bucasas, K., M Franco, L., Shaw, C., Bray, M., Wells, J.,
Ni
˜
no, D., Arden, N., Quarles, J., Couch, R., and Bel-
mont, J. (2011). Early patterns of gene expression cor-
relate with the humoral immune response to influenza
vaccination in humans. The Journal of Infectious Dis-
eases, 203:921–9.
Dash, M. and Liu, H. (1997). Feature selection for classifi-
cation. Intelligent Data Analysis, 1(3):131–156.
Gardner, M. W. and Dorling, S. (1998). Artificial neural
networks (the multilayer perceptron)—a review of ap-
plications in the atmospheric sciences. Atmospheric
Environment, 32(14-15):2627–2636.
Guyon, I. and Elisseeff, A. (2003). An introduction to vari-
able and feature selection. Journal of Machine Learn-
ing Research, 3(Mar):1157–1182.
Hanley, J. A. and McNeil, B. J. (1982). The meaning and
use of the area under a receiver operating characteris-
tic (roc) curve. Radiology, 143(1):29–36.
Hornik, K., Stinchcombe, M., and White, H. (1989). Multi-
layer feedforward networks are universal approxima-
tors. Neural Networks, 2(5):359–366.
Hsu, C.-W., Chang, C., and Lin, C.-J. (2016). A practical
guide to support vector classification. https://www.
csie.ntu.edu.tw/ cjlin/papers/guide/guide.pdf.
Jolliffe, I. (2011). Principal component analysis. In In-
ternational Encyclopedia of Statistical Science, pages
1094–1096. Springer.
Karlik, B. and Olgac, A. V. (2011). Performance analysis of
various activation functions in generalized mlp archi-
tectures of neural networks. International Journal of
Artificial Intelligence and Expert Systems, 1(4):111–
122.
Malaria Vaccine Initiative (2017). RTS, S. https://www.
malariavaccine.org/malaria-and-vaccines/first-
generation-vaccine/rtss.
Sanford, J. P., Philipose, N. M., Mitchell, V. S., et al. (1993).
The Children’s Vaccine Initiative: Achieving the Vi-
sion. National Academies Press.
Simon, D. and Boring, J. R. (1990). The History, Physi-
cal, and Laboratory Examinations. 3rd edition. But-
terworths.
ThermoFisher (2001). Genechip
TM
human genome U133
plus 2.0 array. https://www.thermofisher.com/order/
catalog/product/900468.
Touati, R., Messaoudi, I., ElloumiOueslati, A., and Lachiri,
Z. (2018). Classification of Helitron’s Types in the
C.elegans Genome based on Features Extracted from
Wavelet Transform and SVM Methods. In Proceed-
ings of the 11th International Joint Conference on
Biomedical Engineering Systems and Technologies -
Volume 4: BIOINFORMATICS,, pages 127–134. IN-
STICC, SciTePress.
Trtica-Majnaric, L., Zekic-Susac, M., Sarlija, N., and Vi-
tale, B. (2010). Prediction of influenza vaccination
outcome by neural networks and logistic regression.
Journal of Biomedical Informatics, 43(5):774–781.
US Food and Drug Administration (2017). 22 Case
Studies Where Phase 2 and Phase 3 Trials had Diver-
gent Results. https://www.fda.gov/downloads/
aboutfda/reportsmanual
sforms/reports/ucm535780.pdf.
Vahey, M. T., Wang, Z., Kester, K. E., Cummings, J., Hep-
pner Jr, D. G., Nau, M. E., Ofori-Anyinam, O., Co-
hen, J., Coche, T., Ballou, W. R., et al. (2010). Ex-
pression of genes associated with immunoproteasome
processing of major histocompatibility complex pep-
tides is indicative of protection with adjuvanted RTS,
S malaria vaccine. The Journal of Infectious Diseases,
201(4):580–589.
Van Dyk, D. A. and Meng, X.-L. (2001). The art of data
augmentation. Journal of Computational and Graph-
ical Statistics, 10(1):1–50.
Wilson, C. S., Davidson, G. S., Martin, S. B., Andries, E.,
Potter, J., Harvey, R., Ar, K., Xu, Y., Kopecky, K. J.,
Ankerst, D. P., et al. (2006). Gene expression profil-
ing of adult acute myeloid leukemia identifies novel
biologic clusters for risk classification and outcome
prediction. Blood, 108(2):685–696.
Zhang, C. and Woodland, P. C. (2015). Parameterised
sigmoid and relu hidden activation functions for dnn
acoustic modelling. In Sixteenth Annual Conference
of the International Speech Communication Associa-
tion.
Zhu, X. and Wu, X. (2004). Class noise vs. attribute noise:
A quantitative study. Artificial Intelligence Review,
22(3):177–210.
BIOINFORMATICS 2019 - 10th International Conference on Bioinformatics Models, Methods and Algorithms
162