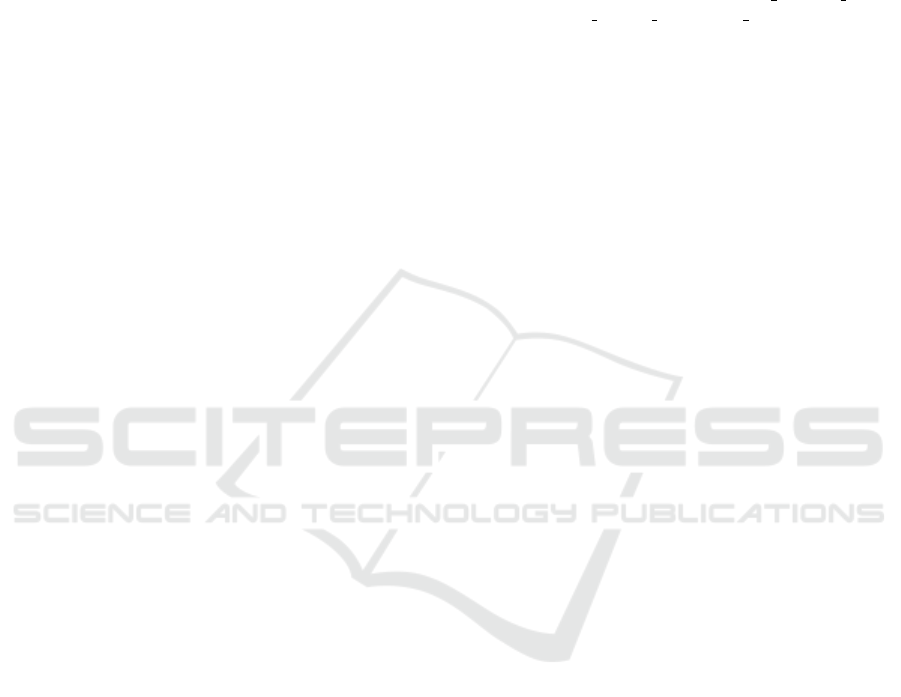
5 CONCLUSION AND
DISCUSSION
In this paper we have presented a new method for
predicting and classifying pair-activities of vehicles
using a new deep learning framework. Our method
uses the QTC representation, and we have constructed
corresponding image textures for each QTC trajec-
tory using one-hot vector. Our trajectory represen-
tation, successfully encodes different types of vehi-
cles activities, and is used as an input for Tra jNet.
Tra jNet offers a compact network for classifying
pair-wise vehicle interactions. We also demonstrate
how we efficiently used limited amount of dataset to
train Tra jNet, and achieved high accuracy classifica-
tion rates across different and challenging datasets.
We have conducted direct comparisons against the
state-of-the-art qualitative (AlZoubi et al., 2017) and
quantitative (Lin et al., 2013) methods, which have
itself been shown to outperform other recent met-
hods. We have shown that our classification met-
hod outperforms that developed by (Lin et al., 2013)
and (AlZoubi et al., 2017); for the classification of
traffic data, we achieved 1.16% error rate, compared
to 3.44%, 4.58%, 16.98%, 27.24%, and 16.48% of
(AlZoubi et al., 2017), (Lin et al., 2013), (Zhou et al.,
2008), (Ni et al., 2009) and (Lin et al., 2010), respecti-
vely.
We have also presented our vehicle-obstacle in-
teraction dataset for complete and incomplete scena-
rios, which provides a detailed and useful resource
for researchers studying vehicle-obstacle behaviors,
and is publicly available for download. We evaluated
our classification method on this dataset, we achieved
0.0% and 0.3% for both complete and predicted sce-
narios datasets, respectively. This again demonstrates
the effectiveness of our activity recognition method.
To predict a full scenario from partial-observed one
we have presented a FFNN. We evaluated our trajec-
tory prediction method on the same vehicle-obstacle
dataset and we achieved average error of 0.4m.
Encouraged by our results, we plan to extend our
work by integrating our vehicles activity recognition
method with our ongoing project of autonomous vehi-
cle system to provide valuable information about the
type of the scenario the vehicle is in (or about to enter)
to increase the safety and to help in decision making
processes.
REFERENCES
Ahmed, S. A., Dogra, D. P., Kar, S., and Roy, P. P.
(2018). Trajectory-based surveillance analysis: A sur-
vey. IEEE Transactions on Circuits and Systems for
Video Technology.
AlZoubi, A., Al-Diri, B., Pike, T., Kleinhappel, T., and
Dickinson, P. (2017). Pair-activity analysis from video
using qualitative trajectory calculus. IEEE Transacti-
ons on Circuits and Systems for Video Technology.
AlZoubi, A. and Nam, D. (2018). Vehicle Ob-
stacle Interaction Dataset (VOIDataset).
https://figshare.com/articles/Vehicle Obstacle
Interaction Dataset VOIDataset /6270233.
Chavoshi, S. H., De Baets, B., Neutens, T., Delafontaine,
M., De Tr
´
e, G., and de Weghe, N. V. (2015). Mo-
vement pattern analysis based on sequence signatu-
res. ISPRS International Journal of Geo-Information,
4(3):1605–1626.
Deng, J., Dong, W., Socher, R., Li, L.-J., Li, K., and Fei-Fei,
L. (2009). Imagenet: A large-scale hierarchical image
database. In Computer Vision and Pattern Recogni-
tion, 2009. CVPR 2009. IEEE Conference on, pages
248–255. IEEE.
Dodge, S., Laube, P., and Weibel, R. (2012). Movement si-
milarity assessment using symbolic representation of
trajectories. International Journal of Geographical
Information Science, 26(9):1563–1588.
Dubuisson, M.-P. and Jain, A. K. (1994). A modified haus-
dorff distance for object matching. In Proceedings of
12th international conference on pattern recognition,
pages 566–568. IEEE.
Hanheide, M., Peters, A., and Bellotto, N. (2012). Analy-
sis of human-robot spatial behaviour applying a qua-
litative trajectory calculus. In RO-MAN, 2012 IEEE,
pages 689–694. IEEE.
Khosroshahi, A., Ohn-Bar, E., and Trivedi, M. M. (2016).
Surround vehicles trajectory analysis with recurrent
neural networks. In Intelligent Transportation Systems
(ITSC), 2016 IEEE 19th International Conference on,
pages 2267–2272. IEEE.
Krizhevsky, A., Sutskever, I., and Hinton, G. E. (2012).
Imagenet classification with deep convolutional neu-
ral networks. In Advances in neural information pro-
cessing systems, pages 1097–1105.
Lin, W., Chu, H., Wu, J., Sheng, B., and Chen, Z. (2013). A
heat-map-based algorithm for recognizing group acti-
vities in videos. IEEE Transactions on Circuits and
Systems for Video Technology, 23(11):1980–1992.
Lin, W., Sun, M.-T., Poovendran, R., and Zhang, Z. (2010).
Group event detection with a varying number of group
members for video surveillance. IEEE Transacti-
ons on Circuits and Systems for Video Technology,
20(8):1057–1067.
Liu, W., Wang, Z., Liu, X., Zeng, N., Liu, Y., and Alsaadi,
F. E. (2017). A survey of deep neural network ar-
chitectures and their applications. Neurocomputing,
234:11–26.
Ni, B., Yan, S., and Kassim, A. (2009). Recognizing human
group activities with localized causalities. In Compu-
ter Vision and Pattern Recognition, 2009. CVPR 2009.
IEEE Conference on, pages 1470–1477. IEEE.
Ohn-Bar, E. and Trivedi, M. M. (2016). Looking at hu-
mans in the age of self-driving and highly automated
Vehicle Activity Recognition using Mapped QTC Trajectories
37