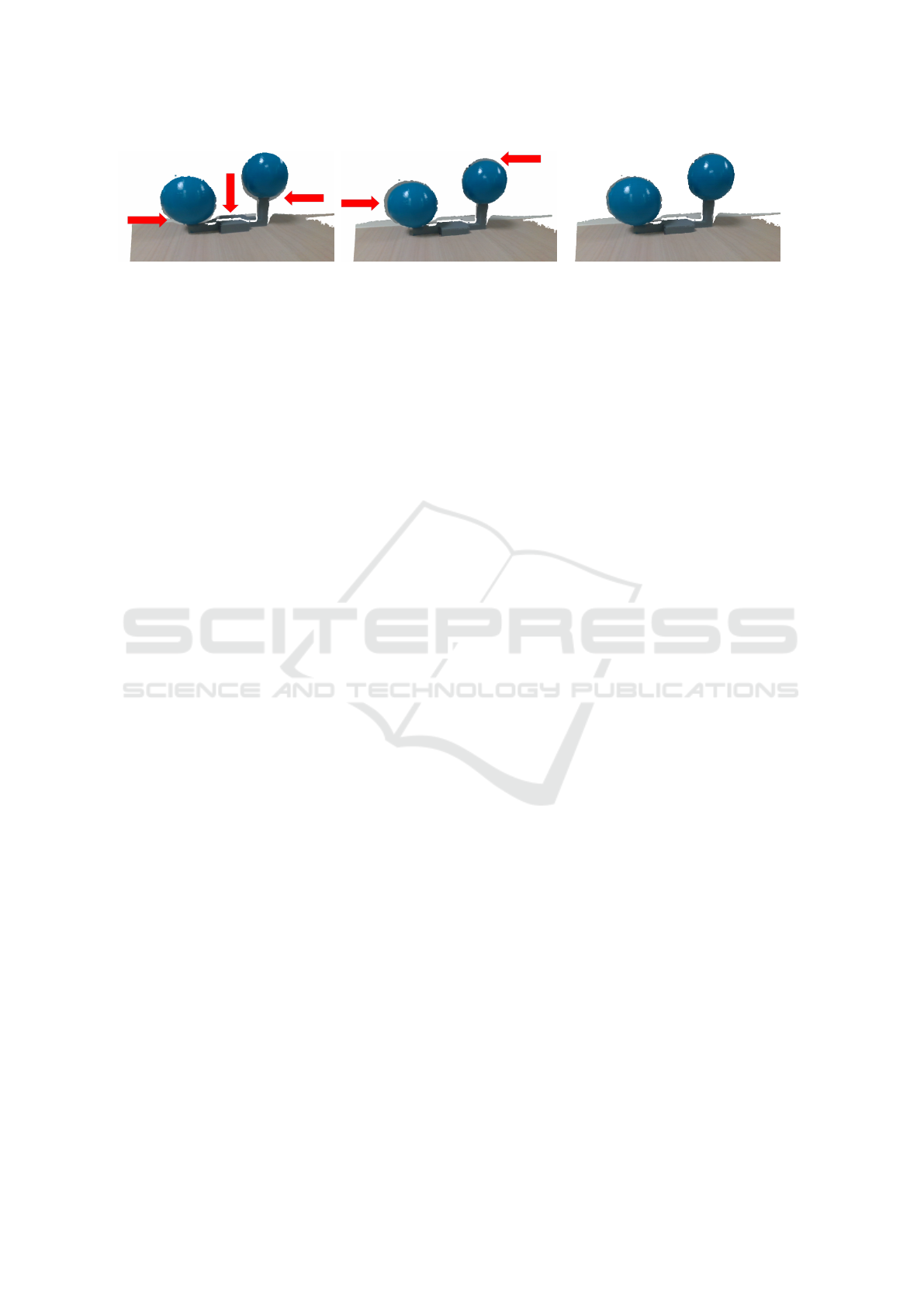
(a) Manufacturer calibration. (b) Initialization calibration. (c) Proposed minimization. Staranowicz
et al. minimization alignment is similar.
Figure 7: Alignment results on the Double dataset. Misalignments are highlighted in red.
pically observed by Depth cameras. These methods
are restricted to low range RGB-D calibration, as no
modelization of the Depth noise is proposed. In future
work, we seek to deal with the noise increasing with
the distance observed by Depth camera, and improve
the ellipse back projection accuracy.
6 CONCLUSION
This work demonstrates the sensitivity of the Starano-
wicz et al. method (Staranowicz et al., 2014) to noisy
measurements. We proposed a new minimization ob-
jective function to better constrain the relative transla-
tion and the intrinsic parameters of the Depth camera.
Multiple simulations with both synthetic data that re-
produces real conditions and real data were perfor-
med to determine and quantify the evolution of cali-
bration parameters. We showed that using the 3D cen-
ters of spheres, instead of their 2D projection allows
to improve the calibration parameters estimation, es-
pecially with high accuracy cameras and noisy Depth
data.
REFERENCES
Basso, F., Menegatti, E., and Pretto, A. (2018). Robust In-
trinsic and Extrinsic Calibration of RGB-D Cameras.
IEEE Transactions on Robotics, pages 01–18.
Boas, D. J. T., Ramel, J.-Y., Slimane, M., and Poltaretskyii,
S. (2018). Rgb-d sphere-based calibration dataset.
http://www.rfai.li.univ-tours.fr/tools-and-demos/.
Darwish, W., Tang, S., Li, W., and Chen, W. (2017). A New
Calibration Method for Commercial RGB-D Sensors.
Sensors, 17(6):1204.
Guan, J., Deboeverie, F., Slembrouck, M., van Haeren-
borgh, D., van Cauwelaert, D., Veelaert, P., and Phi-
lips, W. (2015). Extrinsic Calibration of Camera Net-
works Using a Sphere. Sensors, 15(8):18985–19005.
Hartley, R. and Zisserman, A. (2004). Multiple View Ge-
ometry in Computer Vision. Cambridge University
Press, ISBN: 0521540518, second edition.
Herrera C., D., Kannala, J., and Heikkila, J. (2012). Joint
Depth and Color Camera Calibration with Distortion
Correction. IEEE Transactions on Pattern Analysis
and Machine Intelligence, 34(10):2058–2064.
Liu, Z., Wu, Q., Wu, S., and Pan, X. (2017). Flexible and
accurate camera calibration using grid spherical ima-
ges. Optics Express, 25(13):15269–15285.
Mikhelson, I. V., Lee, P. G., Sahakian, A. V., Wu, Y., and
Katsaggelos, A. K. (2014). Automatic, fast, online
calibration between depth and color cameras. Journal
of Visual Communication and Image Representation,
25(1):218–226.
Minghao Ruan and Huber, D. (2014). Calibration of 3D
Sensors Using a Spherical Target. Proceedings of the
International Conference on 3D Vision, 01:187–193.
Schnieders, D. and Wong, K.-Y. K. (2013). Camera and
light calibration from reflections on a sphere. Compu-
ter Vision and Image Understanding, 117:1536–1547.
Shen, J., Xu, W., Luo, Y., Su, P.-C., and Cheung, S.-c. S.
(2014). Extrinsic calibration for wide-baseline RGB-
D camera network. IEEE 16th International Workshop
on Multimedia Signal Processing .
Staranowicz, A., Brown, G. R., Morbidi, F., and Mariottini,
G. L. (2014). Easy-to-Use and Accurate Calibration of
RGB-D Cameras from Spheres. In Image and Video
Technology, volume 8333, pages 265–278. Springer
Berlin Heidelberg.
Su, P.-C., Shen, J., Xu, W., Cheung, S.-C., and Luo, Y.
(2018). A Fast and Robust Extrinsic Calibration for
RGB-D Camera Networks. Sensors, 18(1):235.
Sun, J., He, H., and Zeng, D. (2016). Global Calibration of
Multiple Cameras Based on Sphere Targets. Sensors,
16(1):77.
Wong, K.-Y. K., Zhang, G., and Chen, Z. (2011). A Strati-
fied Approach for Camera Calibration Using Spheres.
IEEE Transactions on Image Processing, 20(2):305–
316.
Zhang, C. and Zhang, Z. (2014). Calibration Between
Depth and Color Sensors for Commodity Depth Ca-
meras. In Computer Vision and Machine Learning
with RGB-D Sensors, pages 47–64. Springer Interna-
tional Publishing.
VISAPP 2019 - 14th International Conference on Computer Vision Theory and Applications
98