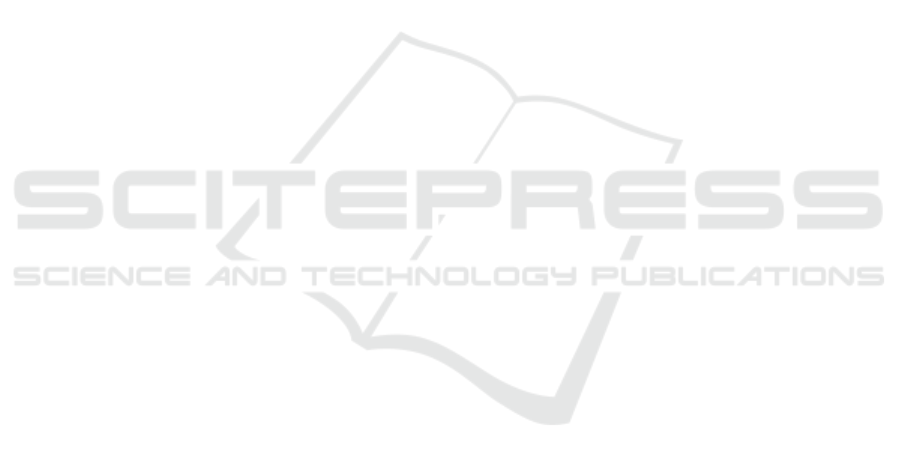
ongoing in implementation of the final phase includ-
ing a large number of participants and a wider variety
of food types to realise the objective of providing scal-
able cost effective remote therapy management and
early warning support dysphagia conditions.
ACKNOWLEDGEMENTS
This work was conducted within the eSwallHome
(ANR research Programme) and was also funded
within the framework of eBioMed chair of BMBI lab-
oratory. This work is also a part of the STIC AMSUD-
EMONITOR project. We would also like to thank
Open Health Institute for their support for our re-
search study.
REFERENCES
Aboofazeli, M. and Moussavi, Z. (2004). Automated clas-
sification of swallowing and breath sounds. IEEE, Vol
3:7803–8439.
Amft, O. and Troster, G. (2006). Methods for detection
and classification of normal swallowing from muscle
activation and sound. In 2006 Pervasive Health Con-
ference and Workshops, pages 1–10.
Amft, O. and Tr
¨
oster, G. (2008). Recognition of dietary
activity events using on-body sensors. Artificial In-
telligence in Medicine, 42(2):121 – 136. Wearable
Computing and Artificial Intelligence for Healthcare
Applications.
Baptista, M., Silva, R., Jardim, H., and Quintal, A. (2018).
Perioperative electronic system - a new approach for
perioperative nursing performance. pages 505–509.
Boyer, C., Dolamic, L., Ruch, P., and Falquet, G. (2016).
Effect of the named entity recognition and sliding
window on the honcode automated detection of hon-
code criteria for mass health online content. In In
Proceedings of the 9th International Joint Conference
on Biomedical Engineering Systems and Technologies
(BIOSTEC 2016), volume 5, pages 151–158.
Brune, T., Brune, B., Eschborn, S., and Brinker, K. (2017).
Automatic visual detection of incorrect endoscope
adaptions in chemical disinfection devices. In In Pro-
ceedings of the 10th International Joint Conference
on Biomedical Engineering Systems and Technologies
(BIOSTEC 2017), pages 305–312.
Dodds, W. J., Logemann, J. A., and Stewart, E. T. (1990).
Radiologic assessment of abnormal oral and pharyn-
geal phases of swallowing. American Roentgen Ray
Society, Vol 154:965–974.
Dong, B. and Biswas, S. (2012). Swallow monitoring
through apnea detection in breathing signal. In 2012
Annual International Conference of the IEEE Engi-
neering in Medicine and Biology Society, pages 6341–
6344.
Hsiao, T. Y., Wang, C. L., Chen, C. N., Hsieh, F. J., and
Shau, Y. W. (2001). Noninvasive assessment of la-
ryngeal phonation function using colour doppler ul-
trasound imaging. Ultrasound in Med. & Bioll, Vol
27:1035–1040.
Huckabee, M.-L., Butler, S. G., Barclay, M., and Jit, S.
(2005). Submental surface electromyographic mea-
surement and pharyngeal pressures during normal and
effortful swallowing. Arch Phys Med Rehabil, Vol
86:2144–2149.
Imtiaz, U., Yamamura, K., Kong, W., Sessa, S., Lin, Z.,
Bartolomeo, L., Ishii, H., Zecca, M., Yamada, Y., and
Takanishi, A. (2014). Application of wireless iner-
tial measurement units and emg sensors for studying
deglutition preliminary results. IEEE, Vol 978:4244–
7929.
INED (February 12, 2013). ”journ
´
ees d’information sur
les troubles de la d
´
eglutition”. https://etablissements.
fhf.fr/annuaire/hopital-actualite.php?id=998.
Jones, B. (2012). Normal and abnormal swallowing: Imag-
ing In Diagnosis And Therapyl. Springer, 2nd edition.
Kawashima, K., Motohashi, P. Y., and Fujishima, P. I.
(2004). Prevalence of dysphagia among community-
dwelling elderly individuals as estimated using a
questionnaire for dysphagia screening. Dysphagia,
19:266–271.
Lazareck, L. J. and Moussavi, Z. (2002). Automated algo-
rithm for swallowing sound detection. In Canadian
Med. and Biol. Eng. Conf.
Lazareck, L. J. and Moussavi, Z. (2004a). Classification of
normal and dysphagic swallows by acoustical means.
IEEE Transactions on Biomedical Engineering, Vol
51:0018–9294.
Lazareck, L. J. and Moussavi, Z. (2004b). Swallowing
sound characteristics in healthy and dysphagic indi-
viduals. IEEE, Vol 3:7803–8439.
Logemann, J. A. (1999). Behavioral management for
oropharyngeal dysphagia. Folia Phoniatr Logop, Vol
51:199–212.
Martin-Harris, B. and Jones, B. (2008). The videofluoro-
graphic swallowing study. Phys Med Rehabil Clin N
Am, Vol 19:769–785.
Miller, J. L. and Watkin, K. L. (1997). Lateral pharyngeal
wall motion during swallowing using real time ultra-
sound. Dysphagia, Vol 12:125–132.
Moussavi, Z. (2005). Assessment of swallowing sounds
stages with hidden markov model. IEEE, Vol 6:7803–
8740.
Penman, J. and Thomson, M. (1998). A review of the tex-
tured diets developed for the management of dyspha-
gia. J Hum Nutr Diet, Vol 11:51–60.
Reynolds, D. and Rose, R. (1995). Robust text-independent
speaker identification using gaussian mixture speaker
models. Speech Commun, 17:121 – 136. Wearable
Computing and Artificial Intelligence for Healthcare
Applications.
Shawker, T. H., Sonies, B., Stone, M., and Baum,
B. J. (1983). Realtime ultrasound visualization of
tongue movement during swallowing. Dysphagia, Vol
11:485–490.
HEALTHINF 2019 - 12th International Conference on Health Informatics
228