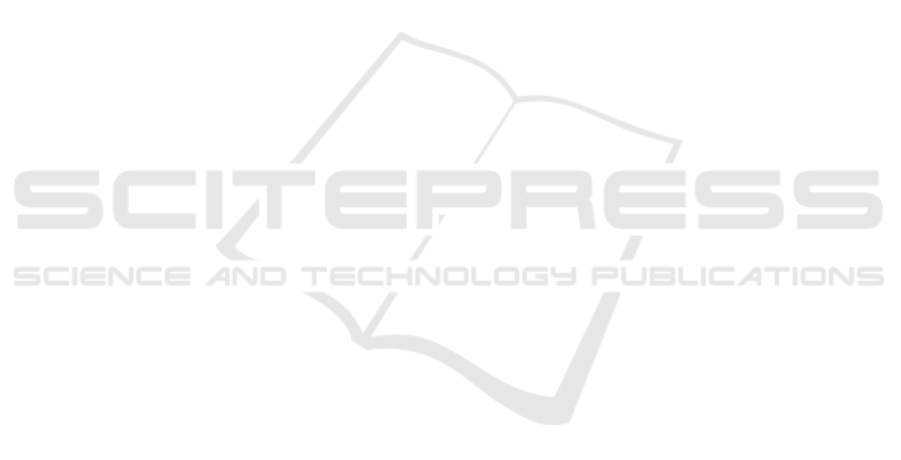
sonal feelings and opinion, which we believe is bene-
ficial for tasks such as estimating the attention level of
a user, to incorporate the subjective nature of attention
itself. We further set up baseline results of attention
level estimation, using our annotations and different
deep learning fusion models. Our best achieved accu-
racy was 80.02% on attention level estimation. As a
future work, we consider labeling the dataset for other
applications in the field related to attention, such as
the VFOA (looking at the TV or not) of each person,
frame-by-frame. Shift of attention labels can also be
added based on either a new labeling session or either
by using the information available in the current at-
tention level labels, e.g annotating the frames where
the attention shifts from low to mid, mid to high and
high to low.
REFERENCES
Asteriadis, S., Karpouzis, K., and Kollias, S. (2011). The
Importance of Eye Gaze and Head Pose to Estimating
Levels of Attention. 2011 Third International Confe-
rence on Games and Virtual Worlds for Serious Appli-
cations.
Borghi, G., Venturelli, M., Vezzani, R., and Cucchiara, R.
(2017). POSEidon: Face-from-Depth for Driver Pose
Estimation. IEEE Conference on Computer Vision and
Pattern Recognition.
Boston, U. (2018). Boston university common data set
2017-2018.
Chen, H., Wang, G., Xue, J.-H., and He, L. (2016). A novel
hierarchical framework for human action recognition.
Pattern Recognition, 55:148–159.
Gomez-Uribe, C. A. and Hunt, N. (2016). The Netflix Re-
commender System: Algorithms, Business Value, and
Innovation. ACM Transactions on Management Infor-
mation Systems, 6(4):Article No. 13.
Hidalgo, G., Cao, Z., Simon, T., Wei, S.-E., and Joo, H.
(2018). OpenPose: Real-time multi-person keypoint
detection library for body, face, and hands estimation.
original-date: 2017-04-24T14:06:31Z.
Jariwala, K., Dalal, U., and Vincent, A. (2016). A robust eye
gaze estimation using geometric eye features. Third
International Conference on Digital Information Pro-
cessing, Data Mining, and Wireless Communications.
Kar, A. and Corcoran, P. (2017). A Review and Analy-
sis of Eye-Gaze Estimation Systems, Algorithms and
Performance Evaluation Methods in Consumer Plat-
forms. IEEE Access, 5:16495–16519.
Kuang, H., Chan, L. L. H., Liu, C., and Yan, H. (2016).
Fruit classification based on weighted score-level fea-
ture fusion. Journal of Electronic Imaging, 25(1).
Li, Z. and Jarvis, R. (2009). Real time Hand Gesture Re-
cognition using a Range Camera. Australasian Con-
ference on Robotics and Automation (ACRA).
Mancas, M. and Ferrera, V. (2016). How to Measure At-
tention? Mancas M., Ferrera V., Riche N., Taylor J.
(eds) From Human Attention to Computational Atten-
tion, 10.
Mass
´
e, B., Ba, S., and Horaud, R. (2017). Tracking Gaze
and Visual Focus of Attention of People Involved in
Social Interaction. Computing Research Repository,
Computer Science(Computer Vision and Pattern Re-
cognition).
Molina, J., Pajuelo, J. A., and Mart
´
ınez, J. M. (2015). Real-
time Motion-based Hand Gestures Recognition from
Time-of-Flight Video. Springer Science+Business
Media New York 2015.
Murthy G.N., K. and Khan, Z. A. (2014). Cognitive at-
tention behaviour detection systems using Electroen-
cephalograph (EEG) signals. Research Journal of
Pharmacy and Technology, 7(2):238–247.
Nararajasivan, D. and Govindarajan, M. (2016). Location
Based Context Aware user Interface Recommendation
System. Proceedings of the International Conference
on Informatics and Analytics, page Article No. 78.
Steil, J., M
¨
uller, P., Sugano, Y., and Bulling, A. (2018).
Forecasting user attention during everyday mobile in-
teractions using device-integrated and wearable sen-
sors. Proceedings of the 20th International Confe-
rence on Human-Computer Interaction with Mobile
Devices and Services.
Tamdee, P. and Prasad, R. (2018). Context-Aware Com-
munication and Computing: Applications for Smart
Environment. Springer Series in Wireless Technology.
Tseng, C.-H. and Cheng, Y.-H. (2017). A camera-based
attention level assessment tool designed for classroom
usage. The Journal of Supercomputing, pages 1–14.
Yun, K., Honorio, J., Chattopadhyay, D., Berg, T. L., and
Samaras, D. (2012). Two-person interaction detection
using body-pose features and multiple instance lear-
ning. IEEE Computer Society Conference on Compu-
ter Vision and Pattern Recognition Workshops.
Zaletelj, J. and Ko
ˇ
sir, A. (2017). Predicting students’ at-
tention in the classroom from Kinect facial and body
features. EURASIP Journal on Image and Video Pro-
cessing, 2017(80).
Zhang, S., Yang, Y., Xiao, J., Liu, X., Yang, Y., Xie, D.,
and Zhuang, Y. (2018). Fusing Geometric Features for
Skeleton Based Action Recognition using Multilayer
LSTM Networks. IEEE Transactions on Multimedia,
20(9).
VISAPP 2019 - 14th International Conference on Computer Vision Theory and Applications
256