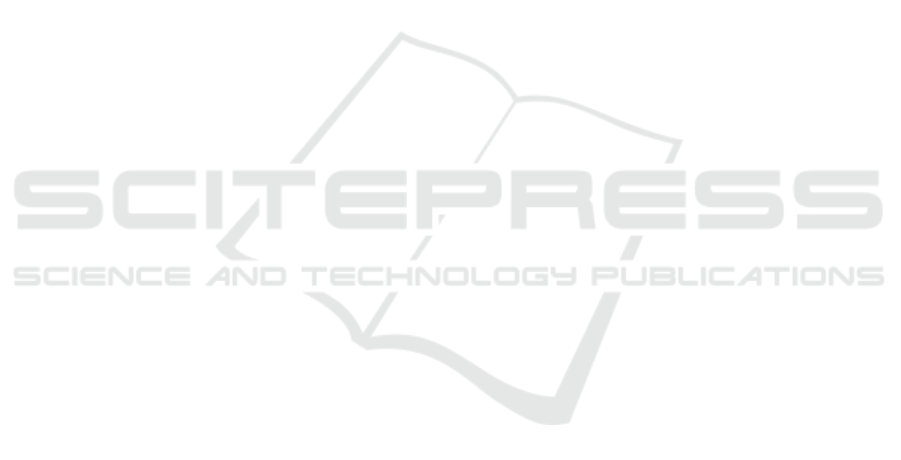
mation. In Proceedings of the European Conference
on Computer Vision (ECCV), pages 614–630.
Janai, J., Guney, F., Ranjan, A., Black, M., and Geiger, A.
(2018). Unsupervised learning of multi-frame optical
flow with occlusions. In Proceedings of the European
Conference on Computer Vision (ECCV), pages 690–
706.
Jason, J. Y., Harley, A. W., and Derpanis, K. G. (2016).
Back to basics: Unsupervised learning of optical flow
via brightness constancy and motion smoothness. In
European Conference on Computer Vision, pages 3–
10. Springer.
Long, G., Kneip, L., Alvarez, J. M., Li, H., Zhang, X., and
Yu, Q. (2016). Learning image matching by simply
watching video. In European Conference on Com-
puter Vision, pages 434–450. Springer.
Makansi, O., Ilg, E., and Brox, T. (2018). Fusionnet
and augmentedflownet: Selective proxy ground truth
for training on unlabeled images. arXiv preprint
arXiv:1808.06389.
Mayer, N., Ilg, E., Hausser, P., Fischer, P., Cremers, D.,
Dosovitskiy, A., and Brox, T. (2016). A large dataset
to train convolutional networks for disparity, optical
flow, and scene flow estimation. In Proceedings of
the IEEE Conference on Computer Vision and Pattern
Recognition, pages 4040–4048.
Menze, M. and Geiger, A. (2015). Object scene flow for
autonomous vehicles. In CVPR.
Papazoglou, A. and Ferrari, V. (2013). Fast object segmen-
tation in unconstrained video. In Proceedings of the
IEEE International Conference on Computer Vision,
pages 1777–1784.
Ranjan, A. and Black, M. J. (2017). Optical flow estimation
using a spatial pyramid network. In IEEE Conference
on Computer Vision and Pattern Recognition (CVPR),
volume 2, page 2. IEEE.
Ren, Z., Yan, J., Ni, B., Liu, B., Yang, X., and Zha, H.
(2017). Unsupervised deep learning for optical flow
estimation. In AAAI, volume 3, page 7.
Sun, D., Yang, X., Liu, M.-Y., and Kautz, J. (2018). Pwc-
net: Cnns for optical flow using pyramid, warping,
and cost volume. In Proceedings of the IEEE Con-
ference on Computer Vision and Pattern Recognition,
pages 8934–8943.
Wang, H., Kl
¨
aser, A., Schmid, C., and Liu, C.-L. (2013).
Dense trajectories and motion boundary descriptors
for action recognition. International journal of com-
puter vision, 103(1):60–79.
Wang, Y., Yang, Y., Yang, Z., Zhao, L., and Xu, W.
(2018). Occlusion aware unsupervised learning of op-
tical flow. In Proceedings of the IEEE Conference
on Computer Vision and Pattern Recognition, pages
4884–4893.
Wannenwetsch, A. S., Keuper, M., and Roth, S. (2017).
Probflow: Joint optical flow and uncertainty estima-
tion. In Computer Vision (ICCV), 2017 IEEE Interna-
tional Conference on, pages 1182–1191. IEEE.
Weinzaepfel, P., Revaud, J., Harchaoui, Z., and Schmid, C.
(2015). Learning to detect motion boundaries. In
The IEEE Conference on Computer Vision and Pat-
tern Recognition (CVPR).
Wulff, J., Butler, D. J., Stanley, G. B., and Black, M. J.
(2012). Lessons and insights from creating a synthetic
optical flow benchmark. In A. Fusiello et al. (Eds.),
editor, ECCV Workshop on Unsolved Problems in Op-
tical Flow and Stereo Estimation, Part II, LNCS 7584,
pages 168–177. Springer-Verlag.
Zhu, Y., Lan, Z., Newsam, S., and Hauptmann, A. G.
(2017). Guided optical flow learning. arXiv preprint
arXiv:1702.02295.
Unsupervised Fine-tuning of Optical Flow for Better Motion Boundary Estimation
783