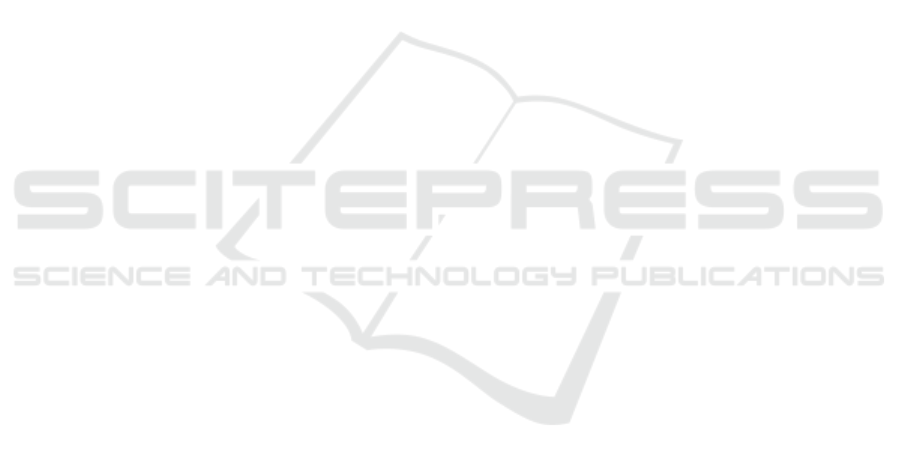
7 SUMMARY
In this paper, we described exploroBOT, a novel sy-
stem developed to support rapid exploration using a
combination of automatic chart generation and intui-
tive navigation.
expoloroBOT enables quick data exploration
using automatic charts and a user-driven decision path
based on the “browsing exploration metaphor” sup-
ported by a novel visual guidance framework. This
allows a fast retrieval of the sought type of chart with
minimal effort in chart design.
Through an evaluation experiment, we found that
exploroBOT enabled swift and accurate data explo-
ration. Considering these results, we reflect on our
approach and suggested several directions for future
work. A video demonstration of exploroBOT is avai-
lable on YouTube: https://youtu.be/C4iPvRvwUEA.
ACKNOWLEDGEMENTS
This work is funded by the Centre for Applied Data
Analytics Research (CeADAR) and co-funded by En-
terprise Ireland (EI) and the International Develop-
ment Agency (IDA) in Ireland.
REFERENCES
Amar, R., Eagan, J., and Stasko, J. (2005). Low-level com-
ponents of analytic activity in information visualiza-
tion. In Proceedings - IEEE Symposium on Informa-
tion Visualization, INFO VIS, pages 111–117.
Bouali, F., Guettala, A., and Venturini, G. (2016). VizAs-
sist: an interactive user assistant for visual data mi-
ning. Visual Computer, 32(11):1447–1463.
Gotz, D., When, Z., Lu, J., Kissa, P., Cao, N., Qian, W. H.,
Liu, S. X., and Zhou, M. X. (2010). HARVEST: An
Intelligent Visual Analytic Tool for the Masses. In
Proceedings of the first international workshop on In-
telligent visual interfaces for text analysis, pages 1–4.
Graham, W. and Wilkinson, L. (2010). Autovis: automatic
visualization. Information Visualization, 9(1):47–69.
Kim, Y., Wongsuphasawat, K., Hullman, J., and Heer, J.
(2017). GraphScape : A Model for Automated Reaso-
ning about Visualization Similarity and Sequencing.
In CHI 2017 Conference on Human Factors in Com-
puting Systems, pages 2628–2638, Denver, CO, USA.
ACM.
Levene, H. (1960). Robust tests for equality of variances. In
Ingram Olkin; Harold Hotelling; et, editor, Contribu-
tions to Probability and Statistics: Essays in Honor of
Harold Hotelling, chapter Robust tes, pages 278–292.
Stanford University Press.
Neuh
¨
auser, M. (2011). Wilcoxon-mann-whitney test. In In-
ternational encyclopedia of statistical science, pages
1656–1658. Springer Berlin Heidelberg.
Pak Chung, W., Cowley, W., Foote, H., Jurrus, E., and Tho-
mas, J. (2000). Visualizing sequential patterns for text
mining. In Information Visualization, 2000. InfoVis
2000. IEEE Symposium on, pages 105–111. IEEE.
Perry, D., Howe, B., Key, A. M. F., and Aragon, C. (2013).
VizDeck: Streamlining Exploratory Visual Analytics
of Scientific Data. In iConference 2013, pages 338–
350, Washington DC, USA.
Saket, B., Kim, H., Brown, E. T., and Endert, A. (2017).
Visualization by Demonstration: An Interaction Para-
digm for Visual Data Exploration. IEEE Transactions
on Visualization and Computer Graphics, 23(1):331–
340.
Salkind, N. J. (2007). Kruskal-Wallis Test. In Encyclopedia
of measurement and statistics. Thousand Oaks, CA:
SAGE Publications, vol.1 edition.
Scheibel, W., Trapp, M., and D
¨
ollner, J. (2016). Interactive
Revision Exploration using Small Multiples of Soft-
ware Maps. In 11th Joint Conference on Computer
Vision, Imaging and Computer Graphics Theory and
Applications IVAPP, volume 2, pages 131–138.
Shapiro, S. S. and Wilk, M. B. (1965). An analysis of va-
riance test for normality (complete samples). Biome-
trika, 52(3/4):591–611.
Shneiderman, B. (1996). The Eyes Have It : A Task by
Data Type Taxonomy for Information Visualizations.
In Visual Languages, 1996. Proceedings., IEEE Sym-
posium on, pages 336–343.
Stolper, C. D., Perer, A., and Gotz, D. (2014). Progres-
sive visual analytics: User-driven visual exploration
of in-progress analytics. IEEE Transactions on Visua-
lization and Computer Graphics, 20(12):1653–1662.
Unwin, A., Theus, M., and Hofmann, H. (2006). Graphics
of large datasets: visualizing a million. Springer
Science & Business Media.
Vartak, M., Huang, S., Siddiqui, T., Madden, S., and Para-
meswaran, A. (2015a). Towards Visualization Recom-
mendation Systems. In Workshop on Data Systems for
Interactive Analytics (DSIA).
Vartak, M., Madden, S., Parameswaran, A., and Polyzotis,
N. (2015b). SEEDB : Supporting Visual Analytics
with Data-Driven Recommendations. In VLDB.
Welch, B. L. (1951). On the Comparison of Several
Mean Values: An Alternative Approach. Biometrika,
38:330–336.
Wongsuphasawat, K., Moritz, D., Anand, A., Mackinlay,
J., Howe, B., and Heer, J. (2016). Voyager: Explora-
tory Analysis via Faceted Browsing of Visualization
Recommendations. In IEEE Transactions on Visuali-
zation and Computer Graphics, volume 1, pages 1–1.
IEEE.
Yalc¸n, M. A., Park, C., and Bederson, B. B. (2016). Cogni-
tive Stages in Visual Data Exploration. In BELIV ’16,
October 24 2016, Baltimore, MD, USA. ACM.
IVAPP 2019 - 10th International Conference on Information Visualization Theory and Applications
232