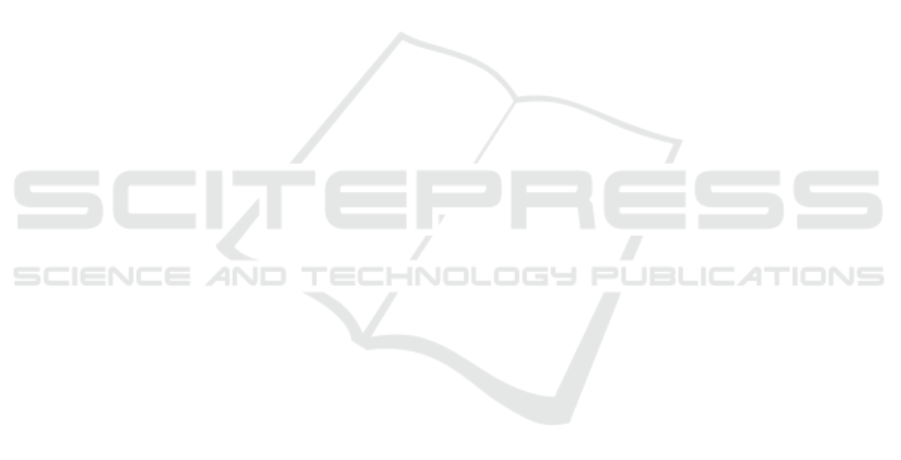
ACKNOWLEDGEMENTS
This work is supported by the Research National
Agency (ANR) in the framework of the 2017 Lab-
Com program (ANR 17-LCV2-0006-01).
REFERENCES
Bishop, C. M. (2006). Pattern Recognition and Machine Le-
arning (Information Science and Statistics). Springer-
Verlag, Berlin, Heidelberg.
Everingham, M., Eslami, S. M., Gool, L., Williams, C. K.,
Winn, J., and Zisserman, A. (2015). The pascal vi-
sual object classes challenge: A retrospective. Int. J.
Comput. Vision, 111(1):98–136.
Everingham, M., Van Gool, L., Williams, C. K. I., Winn,
J., and Zisserman, A. (2010). The pascal visual ob-
ject classes (voc) challenge. International Journal of
Computer Vision, 88(2):303–338.
H
¨
ausser, P., Mordvintsev, A., and Cremers, D. (2017). Lear-
ning by association - A versatile semi-supervised trai-
ning method for neural networks. In CVPR 2017, Ho-
nolulu, HI, USA, 2017, pages 626–635.
He, K., Gkioxari, G., Doll
´
ar, P., and Girshick, R. B. (2017).
Mask R-CNN. CoRR, abs/1703.06870.
Kumar, M. P., Packer, B., and Koller, D. (2010). Self-paced
learning for latent variable models. In Neural Infor-
mation Processing Systems - Volume 01, NIPS’10, pa-
ges 1189–1197, USA. Curran Associates Inc.
Lee, D.-H. (2013). Pseudo-label : The simple and efficient
semi-supervised learning method for deep neural net-
works.
Lin, T.-Y., Goyal, P., Girshick, R. B., He, K., and Doll
´
ar, P.
(2017). Focal loss for dense object detection. 2017
IEEE International Conference on Computer Vision
(ICCV), pages 2999–3007.
Lin, T.-Y., Maire, M., Belongie, S., Hays, J., Perona, P., Ra-
manan, D., Dollr, P., and Zitnick, C. L. (2014). Micro-
soft coco: Common objects in context. In European
Conference on Computer Vision (ECCV), Zrich. Oral.
Little, R. J. A. and Rubin, D. B. (1986). Statistical Analysis
with Missing Data. John Wiley & Sons, Inc., New
York, NY, USA.
Liu, W., Anguelov, D., Erhan, D., Szegedy, C., Reed, S.,
Fu, C.-Y., and Berg, A. C. (2016). Ssd: Single shot
multibox detector. arXiv preprint arXiv:1512.02325.
Papandreou, G., Chen, L.-C., Murphy, K. P., and Yuille,
A. L. (2015). Weakly-and semi-supervised learning
of a deep convolutional network for semantic image
segmentation. In IEEE International Conference on
Computer Vision, ICCV ’15, pages 1742–1750, Wa-
shington, DC, USA. IEEE Computer Society.
Redmon, J. and Farhadi, A. (2016). YOLO9000: Better,
Faster, Stronger.
Ren, S., He, K., Girshick, R., and Sun, J. (2015). Faster
R-CNN: Towards Real-Time Object Detection with
Region Proposal Networks. In Cortes, C., Lawrence,
N. D., Lee, D. D., Sugiyama, M., and Garnett, R., edi-
tors, Advances in Neural Information Processing Sys-
tems 28, pages 91–99. Curran Associates, Inc.
Rosenberg, C., Hebert, M., and Schneiderman, H. (2005).
Semi-supervised self-training of object detection mo-
dels. In WACV/MOTION’05 - Volume 01, pages 29–
36, Washington, DC, USA. IEEE Computer Society.
Sajjadi, M., Javanmardi, M., and Tasdizen, T. (2016). Re-
gularization with stochastic transformations and per-
turbations for deep semi-supervised learning. In Neu-
ral Information Processing Systems 2016, December
5-10, 2016, Barcelona, Spain, pages 1163–1171.
Yan, Z., Liang, J., Pan, W., Li, J., and Zhang, C.
(2017). Weakly- and semi-supervised object detection
with expectation-maximization algorithm. CoRR,
abs/1702.08740.
VISAPP 2019 - 14th International Conference on Computer Vision Theory and Applications
296