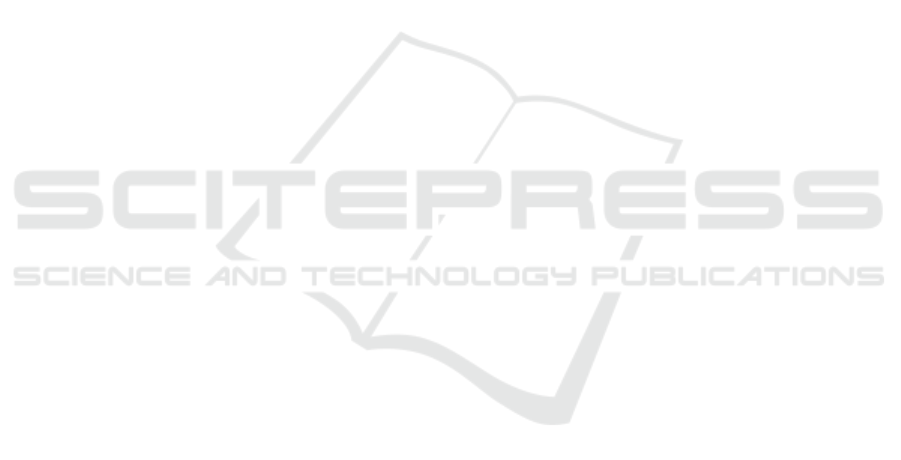
Organization, W. H. (2015). The Selection and Use of Es-
sential Medicines: Report of the WHO Expert Com-
mittee, 2015 (including the 19th WHO Model List
of Essential Medicines and the 5th WHO Model List
of Essential Medicines for Children). Number 994.
World Health Organization.
Pham, B. T., Bui, D. T., Prakash, I., and Dholakia, M.
(2017). Hybrid integration of multilayer perceptron
neural networks and machine learning ensembles for
landslide susceptibility assessment at himalayan area
(india) using gis. Catena, 149:52–63.
Ponzio, F., Macii, E., Ficarra, E., and Cataldo, S. D.
(2018). Colorectal cancer classification using deep
convolutional networks. In Proceedings of the 11th
International Joint Conference on Biomedical En-
gineering Systems and Technologies - Volume 2:
BIOIMAGING, (BIOSTEC 2018), pages 58–66. IN-
STICC, SciTePress.
Rajpurkar, P., Irvin, J., Zhu, K., Yang, B., Mehta, H., Duan,
T., Ding, D., Bagul, A., Langlotz, C., Shpanskaya, K.,
et al. (2017). Chexnet: Radiologist-level pneumonia
detection on chest x-rays with deep learning. arXiv
preprint arXiv:1711.05225.
Salamon, J. and Bello, J. P. (2017). Deep convolutional neu-
ral networks and data augmentation for environmental
sound classification. IEEE Signal Processing Letters,
24(3):279–283.
Saraiva, A., Barros, M., Nogueira, A., Fonseca Ferreira, N.,
and Valente, A. (2018a). Virtual interactive environ-
ment for low-cost treatment of mechanical strabismus
and amblyopia. Information, 9(7):175.
Saraiva, A., Ferreira, N., and Valente, A. (2018b). New
bioinspired filter of dicom images.
Saraiva, A., Melo, R., Filipe, V., Sousa, J., Ferreira, N. F.,
and Valente, A. (2018c). Mobile multirobot manipu-
lation by image recognition.
Saraiva, A., Miranda de Jesus Castro, F., Ferreira, N.,
and Valente, A. (2018d). Compression of electro-
cardiographs comparative study between the walsh
hadamard transform and discrete cosine transform.
Saraiva, A. A., , N. F. F., Salviano F.S.P. Soares and, M. J.
C. S. R., and Antonio, V. (2018e). Filtering of cardiac
signals with mathematical morphology for qrs detec-
tion. Proceedings of ICAT’18, 7th International Con-
ference on Advanced Technologies.
Saraiva, A. A., Costa, N., Sousa, J. V. M., De Araujo, T. P.,
Ferreira, N. F., and Valente, A. (2018f). Scalable task
cleanup assignment for multi-agents. In Memorias de
Congresos UTP, volume 1, pages 439–446.
Saraiva, A. A., Nogueira, A. T., Ferreira, N. F., and Valente,
A. (2018g). Application of virtual reality for the treat-
ment of strabismus and amblyopia. In 2018 IEEE 6th
International Conference on Serious Games and Ap-
plications for Health (SeGAH), pages 1–7. IEEE.
Saraiva, A. A., SANTOS, D. S., JUNIOR, F. M., SOUSA,
J. V. M., FERREIRA, N. F., and Valente, A.
(2018h). Navigation of quadruped multirobots by ges-
ture recognition using restricted boltzmann machines.
In Memorias de Congresos UTP, volume 1, pages
431–438.
Sivaramakrishnan, R., Antani, S., Xue, Z., Candemir, S.,
Jaeger, S., and Thoma, G. (2017). Visualizing abnor-
malities in chest radiographs through salient network
activations in deep learning. In Life Sciences Confer-
ence (LSC), 2017 IEEE, pages 71–74. IEEE.
Tang, J., Deng, C., and Huang, G.-B. (2016). Extreme
learning machine for multilayer perceptron. IEEE
transactions on neural networks and learning systems,
27(4):809–821.
Ustinova, E., Ganin, Y., and Lempitsky, V. (2017). Multi-
region bilinear convolutional neural networks for per-
son re-identification. In Advanced Video and Signal
Based Surveillance (AVSS), 2017 14th IEEE Interna-
tional Conference on, pages 1–6. IEEE.
Vajda, S., Karargyris, A., Jaeger, S., Santosh, K., Candemir,
S., Xue, Z., Antani, S., and Thoma, G. (2018). Fea-
ture selection for automatic tuberculosis screening in
frontal chest radiographs. Journal of medical systems,
42(8):146.
Vehtari, A., Gelman, A., and Gabry, J. (2017). Prac-
tical bayesian model evaluation using leave-one-out
cross-validation and waic. Statistics and Computing,
27(5):1413–1432.
Wang, X., Peng, Y., Lu, L., Lu, Z., Bagheri, M., and Sum-
mers, R. M. (2017). Chestx-ray8: Hospital-scale chest
x-ray database and benchmarks on weakly-supervised
classification and localization of common thorax dis-
eases. In Computer Vision and Pattern Recogni-
tion (CVPR), 2017 IEEE Conference on, pages 3462–
3471. IEEE.
Xue, Z., Antani, S., Long, L. R., and Thoma, G. R. (2018).
Using deep learning for detecting gender in adult chest
radiographs. In Medical Imaging 2018: Imaging In-
formatics for Healthcare, Research, and Applications,
volume 10579, page 105790D. International Society
for Optics and Photonics.
Yu, S., Jia, S., and Xu, C. (2017). Convolutional neural
networks for hyperspectral image classification. Neu-
rocomputing, 219:88–98.
Models of Learning to Classify X-ray Images for the Detection of Pneumonia using Neural Networks
83