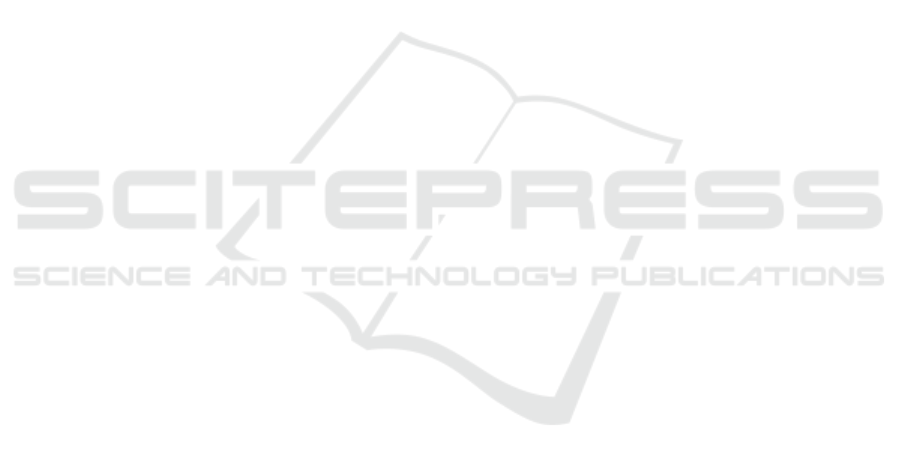
Hahnloser, R. H., Sarpeshkar, R., Mahowald, M. A., Dou-
glas, R. J., and Seung, H. S. (2000). Digital selec-
tion and analogue amplification coexist in a cortex-
inspired silicon circuit. Nature, 405(6789):947.
Hochreiter, S. and Schmidhuber, J. (1997). Long short-term
memory. Neural Computation, 9(8):1735–1780.
Hong, S., Wu, M., Zhou, Y., Wang, Q., Shang, J., Li, H., and
Xie, J. (2017). ENCASE: An ENsemble ClASsifiEr
for ECG classification using expert features and deep
neural networks. In 2017 Computing in Cardiology
(CinC), pages 1–4.
January, C. T., Wann, L. S., Alpert, J. S., Calkins, H., Cigar-
roa, J. E., Cleveland, J. C., Conti, J. B., Ellinor, P. T.,
Ezekowitz, M. D., Field, M. E., Murray, K. T., Sacco,
R. L., Stevenson, W. G., Tchou, P. J., Tracy, C. M., and
Yancy, C. W. (2014). 2014 AHA/ACC/HRS guide-
line for the management of patients with atrial fibril-
lation: a report of the American College of Cardiol-
ogy/American Heart Association task force on prac-
tice guidelines and the Heart Rhythm Society. Journal
of the American College of Cardiology, 64(21):e1–
e76.
Kannel, W. B., Wolf, P. A., Benjamin, E. J., and Levy, D.
(1998). Prevalence, incidence, prognosis, and predis-
posing conditions for atrial fibrillation: population-
based estimates. The American journal of cardiology,
82(7):2N–9N.
Kingma, D. P. and Ba, J. (2014). Adam: A
method for stochastic optimization. arXiv preprint
arXiv:1412.6980.
Krizhevsky, A., Sutskever, I., and Hinton, G. E. (2012). Im-
ageNet classification with deep convolutional neural
networks. In Advances in Neural Information Pro-
cessing Systems 25, pages 1097–1105.
LeCun, Y. A., Bottou, L., Orr, G. B., and M
¨
uller, K.-
R. (2012). Efficient backprop. In Neural networks:
Tricks of the trade, pages 9–48. Springer.
Nattel, S., Burstein, B., and Dobrev, D. (2008). Atrial re-
modeling and atrial fibrillation: mechanisms and im-
plications. Circulation: Arrhythmia and Electrophys-
iology, 1(1):62–73.
Owis, M. I., Abou-Zied, A. H., Youssef, A.-B., and Kadah,
Y. M. (2002). Study of features based on nonlinear
dynamical modeling in ECG arrhythmia detection and
classification. IEEE Transactions on Biomedical En-
gineering, 49(7):733–736.
Rajpurkar, P., Hannun, A. Y., Haghpanahi, M., Bourn, C.,
and Ng, A. Y. (2017). Cardiologist-level arrhythmia
detection with convolutional neural networks. arXiv
preprint arXiv:1707.01836.
Srivastava, N., Hinton, G., Krizhevsky, A., Sutskever, I.,
and Salakhutdinov, R. (2014). Dropout: a simple way
to prevent neural networks from overfitting. The Jour-
nal of Machine Learning Research, 15(1):1929–1958.
Teijeiro, T., Garc
´
ıa, C. A., Castro, D., and F
´
elix, P. (2017).
Arrhythmia classification from the abductive interpre-
tation of short single-lead ECG records. In 2017 Com-
puting in Cardiology (CinC), pages 1–4.
Wang, T. J., Larson, M. G., Levy, D., Vasan, R. S., Leip,
E. P., Wolf, P. A., D’Agostino, R. B., Murabito, J. M.,
Kannel, W. B., and Benjamin, E. J. (2003). Temporal
relations of atrial fibrillation and congestive heart fail-
ure and their joint influence on mortality: the Fram-
ingham heart study. Circulation, 107(23):2920–2925.
Xia, Y., Wulan, N., Wang, K., and Zhang, H. (2018). De-
tecting atrial fibrillation by deep convolutional neu-
ral networks. Computers in Biology and Medicine,
93:84–92.
Xiong, Z., Stiles, M. K., and Zhao, J. (2017). Robust ECG
signal classification for detection of atrial fibrillation
using a novel neural network. In 2017 Computing in
Cardiology (CinC), pages 1–4.
Zabihi, M., Rad, A. B., Katsaggelos, A. K., Kiranyaz, S.,
Narkilahti, S., and Gabbouj, M. (2017). Detection
of atrial fibrillation in ECG hand-held devices using
a random forest classifier. In 2017 Computing in Car-
diology (CinC), pages 1–4.
Zihlmann, M., Perekrestenko, D., and Tschannen, M.
(2017). Convolutional recurrent neural networks for
electrocardiogram classification. In 2017 Computing
in Cardiology (CinC), pages 1–4.
Classification of Cardiac Arrhythmias from Single Lead ECG with a Convolutional Recurrent Neural Network
41