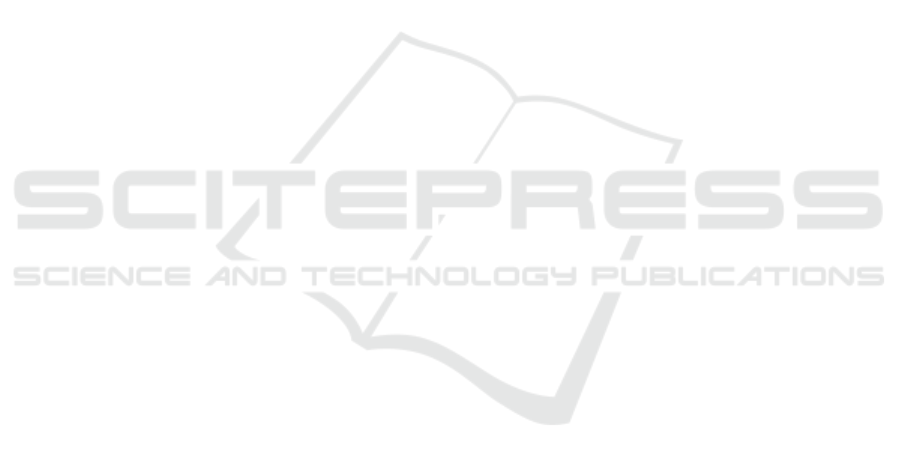
and RNA-binding proteins by deep learning. Nature
Biotechnology, 33:831–838.
Benson, D., Karsch-Mizrachi, I., Lipman, D., Ostell, J., and
Sayers, E. (2011). Genbank. Nucleic Acids Research,
39:D32–D37.
Beyer, A., Christensen, M., Walker, B., and LeStourgeon,
W. (1977). Identification and characterization of the
packaging proteins of core 40S hnRNP particles. Cell,
11:127–138.
Breiman, L. (2001). Random forests. Machine Learning,
45:5–32.
Dunn, S., Wahl, L., and Gloor, G. (2008). Mutual in-
formation without the influence of phylogeny or en-
tropy dramatically improves residue contact predic-
tion. Bioinformatics, 24:333–340.
Feng, G.-S., Chong, K., Kumar, A., and Williams, B.
(1992). Identification of double-stranded RNA-
binding domains in the interferon-induced double-
stranded RNA-activated p68 kinase. Proc. Natl. Acad.
Sci. USA, 89:5447–5451.
Finn, R. D., Coggill, P., Eberhardt, R. Y., Eddy, S. R.,
Mistry, J., Mitchell, A. L., Potter, S. C., Punta, M.,
Qureshi, M., Sangrador-Vegas, A., Salazar, G. A.,
Tate, J., and Bateman, A. (2016). The Pfam protein
families database: towards a more sustainable future.
Nucleic Acids Research, 44(D1):D279–D285.
Glisovic, T., Bachorik, J., Yong, J., and Dreyfuss, G. (2008).
RNA-binding proteins and post-transcriptional gene
regulation. FEBS Letters, 582:1977–1986.
Glorot, X., Bordes, A., and Bengio, Y. (2011). Deep sparse
rectifier neural networks. In 14th International Con-
ference on Artificial Intelligence and Statistics, pages
315–323.
Gupta, A. and Gribskov, M. (2011). The role of RNA
sequence and structure in RNA-protein interactions.
Journal of Molecular Biology, 409:574–587.
Hall, T. (2005). Multiple modes of RNA recognition by
zinc finger proteins. Current Opinion in Structural
Biology, 15:367–373.
Hayashida, M., Kamada, M., Song, J., and Akutsu, T.
(2013). Prediction of protein-RNA residue-base con-
tacts using two-dimensional conditional random field
with the lasso. BMC Systems Biology, 7(Suppl 2):S15.
Hayashida, M., Okada, N., Kamada, M., and Koyano, H.
(2018). Improving conditional random field model
for prediction of protein-RNA residue-base contacts.
Quantitative Biology, 6:155–162.
Kalvari, I., Argasinska, J., Quinones-Olvera, N., Nawrocki,
E. P., Rivas, E., Eddy, S. R., Bateman, A., Finn, R. D.,
and Petrov, A. I. (2018). Rfam 13.0: shifting to a
genome-centric resource for non-coding RNA fami-
lies. Nucleic Acids Research, 46(D1):D335–D342.
Kedersha, N., Gupta, M., Li, W., Miller, I., and Anderson,
P. (1999). RNA-binding proteins TIA-1 and TIAR
link the phosphorylation of eIF-2α to the assembly of
mammalian stress granules. Journal of Cell Biology,
147:1431–1441.
Lafferty, J., McCallum, A., and Pereira, F. (2001). Con-
ditional random fields: Probabilistic models for seg-
menting and labeling sequence data. In Proc. Int.
Conf. on Machine Learning.
LeCun, Y., Bengio, Y., and Hinton, G. (2015). Deep learn-
ing. Nature, 521:436–444.
Murphy, L., Wallqvist, A., and Levy, R. (2000). Simpli-
fied amino acid alphabets for protein fold recognition
and implications for folding. Protein Engineering,
13:149–152.
Rose, P. W., Prli
´
c, A., Altunkaya, A., Bi, C., Bradley, A. R.,
Christie, C. H., Costanzo, L. D., Duarte, J. M., Dutta,
S., Feng, Z., Green, R. K., Goodsell, D. S., Hud-
son, B., Kalro, T., Lowe, R., Peisach, E., Randle,
C., Rose, A. S., Shao, C., Tao, Y.-P., Valasatava, Y.,
Voigt, M., Westbrook, J. D., Woo, J., Yang, H., Young,
J. Y., Zardecki, C., Berman, H. M., and Burley, S. K.
(2017). The RCSB protein data bank: integrative view
of protein, gene and 3D structural information. Nu-
cleic Acids Research, 45(D1):D271–D281.
Sharan, M., F
¨
orstner, K., Eulalio, A., and Vogel, J. (2017).
APRICOT: an integrated computational pipeline for
the sequence-based identification and characterization
of RNA-binding proteins. Nucleic Acids Research,
45:e96.
Siomi, H., Matunis, M., Michael, W., and Dreyfuss, G.
(1993). The pre-mRNA binding K protein contains
a novel evolutionary conserved motif. Nucleic Acids
Research, 21:1193–1198.
Sun, M., Wang, X., Zou, C., He, Z., Liu, W., and Li, H.
(2016). Accurate prediction of RNA-binding protein
residues with two discriminative structural descrip-
tors. BMC Bioinformatics, 17(1):231.
Tang, Y., Liu, D., Wang, Z., Wen, T., and Deng, L. (2017).
A boosting approach for prediction of protein-RNA
binding residues. BMC Bioinformatics, 18(13):465.
The UniProt Consortium (2017). UniProt: the univer-
sal protein knowledgebase. Nucleic Acids Research,
45:D158–D169.
Weirauch, M. T., Cote, A., Norel, R., Annala, M., Zhao,
Y., Riley, T. R., Saez-Rodriguez, J., Cokelaer, T., Ve-
denko, A., Talukder, S., Consortium, D., Bussemaker,
H. J., Morris, Q. D., Bulyk, M. L., Stolovitzky, G., and
Hughes, T. R. (2013). Evaluation of methods for mod-
eling transcription factor sequence specificity. Nature
Biotechnology, 31:126–134.
Zeng, H., Edwards, M., Liu, G., and Gifford, D.
(2016). Convolutional neural network architectures
for predicting DNA-protein binding. Bioinformatics,
32:i121–i127.
Zhao, Y., Stormo, G., Feature, N., and Eisenstein, M.
(2011). Quantitative analysis demonstrates most tran-
scription factors require only simple models of speci-
ficity. Nature Biotechnology, 29:480–483.
Artificial Neural Network Approach to Prediction of Protein-RNA Residue-base Contacts
167