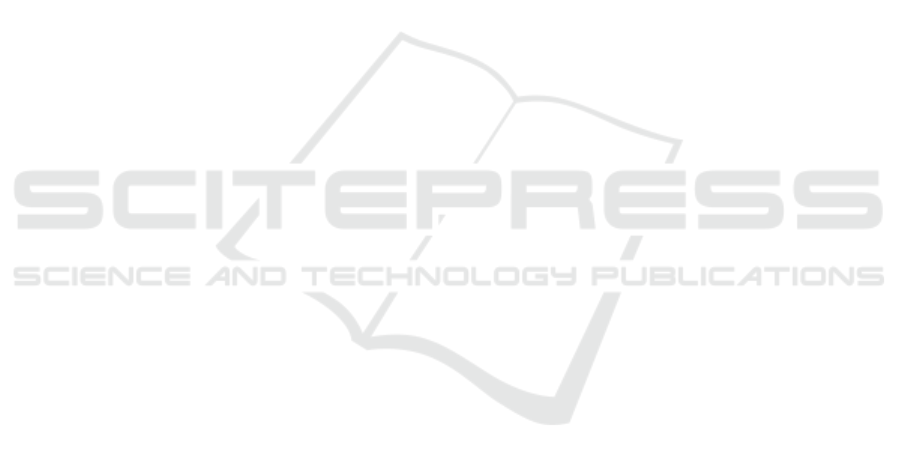
the expectations that during stress, the LF(nu) and
the LF/HF ratio increased with a small decrease in
HF(nu) power with no significance. This group re-
sponds to stress with the withdrawal of the parasym-
pathetic nervous system and the activation of the sym-
pathetic nervous system. For group 1, the results
showed significant decrease in LF(nu) and LF/HF ra-
tio during stress and significant increase in HF(nu)
power. The simultaneous increase in HF(nu) and
heart rate is more difficult to explain, although it could
be an influence of complex respiratory pattern (Vuk-
sanovi
´
c and Gal, 2007), or it could be the effect of
different co-activation humoral mechanims, caused
by compensatory sympatho-adrenal activation with
catecholamine release into the circulation (Terkelsen
et al., 2005).
In terms of EDA, both groups showed an increase
in Band 1 power, although significance was only
found in group 2 between baseline 2 and stress 1 seg-
ments. It is possible to conclude that even if there is
a distinct response to stress in terms of HRV, there is
activation of the sympathetic nervous system during
the stress situation, due to the fact that the sympa-
thetic nervous system influences the heart and sweat
through distinct hormones, respectively, epinephrine
and acetylcholine.
Finally, the classification model implemented in
section 3.3, showed that it was possible to predict the
type of response for each subject during stress, using
only their baseline features for both HRV and EDA
features, making it possible to classify the subjects
into the two different groups, with an accuracy of ap-
proximately 80% for HRV features in baseline and an
accuracy of approximately 77% for HRV and EDA
simultaneous features. This model assumes to be a
good asset for future assessment of the type of re-
sponse when the subjects are under a stress situation.
REFERENCES
Ahmed, M. W., Kadish, A. H., Parker, M. A., and Gold-
berger, J. J. (1994). Effect of physiologic and pharma-
cologic adrenergic stimulation on heart rate variabil-
ity. Journal of the American College of Cardiology,
24(4):1082–1090.
Allen, J. (2007). Photoplethysmography and its application
in clinical physiological measurement. Physiological
Measurement, 28(3).
Bansal, D., Khan, M., and Salhan, A. (2009). A Review of
Measurement and Analysis of Heart Rate Variability.
In 2009 International Conference on Computer and
Automation Engineering, pages 243–246.
Benedek, M. and Kaernbach, C. (2010). A continuous mea-
sure of phasic electrodermal activity. Journal of Neu-
roscience Methods, 190(1):80–91.
Bolanos, M., Nazeran, H., and Haltiwanger, E. (2006).
Comparison of heart rate variability signal features de-
rived from electrocardiography and photoplethysmog-
raphy in healthy individuals. In Annual International
Conference of the IEEE Engineering in Medicine and
Biology - Proceedings, pages 4289–4294.
Boucsein, W. (2012). Electodermal Activity. Second edi
edition.
Breiman, L. (2001). Random forests. Machine Learning,
45(1):5–32.
Brennan, M., Palaniswami, M., and Kamen, P. (2001).
Do existing measures of Poincare
´
e plot geome-
try reflect nonlinear features of heart rate variabil-
ity? IEEE Transactions on Biomedical Engineering,
48(11):1342–1347.
Bussmann, B. (1998). Differentiation of autonomic nervous
activity in different stages of coma displayed by power
spectrum analysis of heart rate variability. pages 46–
52.
Cochran, W. G. (2013). The χ2 Test of Goodness of Fit. The
Annals of Mathematical Statistics, 23(3):315–345.
Donges, N. (2018). The Random Forest Algorithm.
Gamboa, H. and Fred, A. (2008). Electrodermal Activity
Model. Psychophysiology, (April):30.
Guidelines (1996). Guidelines Heart rate variability. Euro-
pean Heart Journal, 17:354–381.
Guyton, A. C. and Hall, J. E. (2011). Textbook of Medical
Physiology.
Hamilton, J. L. and Alloy, L. B. (2016). Atypical reactivity
of heart rate variability to stress and depression across
development: Systematic review of the literature and
directions for future research.
Hjortskov, N., Riss
´
en, D., Blangsted, A. K., Fallentin, N.,
Lundberg, U., and Søgaard, K. (2004). The effect of
mental stress on heart rate variability and blood pres-
sure during computer work. European Journal of Ap-
plied Physiology, 92(1-2):84–89.
Hsu, C. H., Tsai, M. Y., Huang, G. S., Lin, T. C., Chen,
K. P., Ho, S. T., Shyu, L. Y., and Li, C. Y. (2012).
Poincar
´
e plot indexes of heart rate variability detect
dynamic autonomic modulation during general anes-
thesia induction. Acta Anaesthesiologica Taiwanica,
50(1):12–18.
Jang, D.-G., Park, S., Hahn, M., and Park, S.-H. (2014).
A Real-Time Pulse Peak Detection Algorithm for the
Photoplethysmogram. International Journal of Elec-
tronics and Electrical Engineering, 2(1):45–49.
Kleckner, I. R., Jones, R. M., Wilder-Smith, O., Worm-
wood, J. B., Akcakaya, M., Quigley, K. S., Lord, C.,
and Goodwin, M. S. (2017). Simple, Transparent, and
Flexible Automated Quality Assessment Procedures
for Ambulatory Electrodermal Activity Data.
Kuntamalla, S., Ram, L., and Reddy, G. (2014). An Ef-
ficient and Automatic Systolic Peak Detection Algo-
rithm for Photoplethysmographic Signals. Interna-
tional Journal of Computer Applications, 97(19):975–
8887.
Langewitz, W. and Ruddel, H. (1989). Spectral analysis of
heart rate variability under mental stress. J Hypertens
Suppl, 7(6):S32—-3.
BIOSIGNALS 2019 - 12th International Conference on Bio-inspired Systems and Signal Processing
50