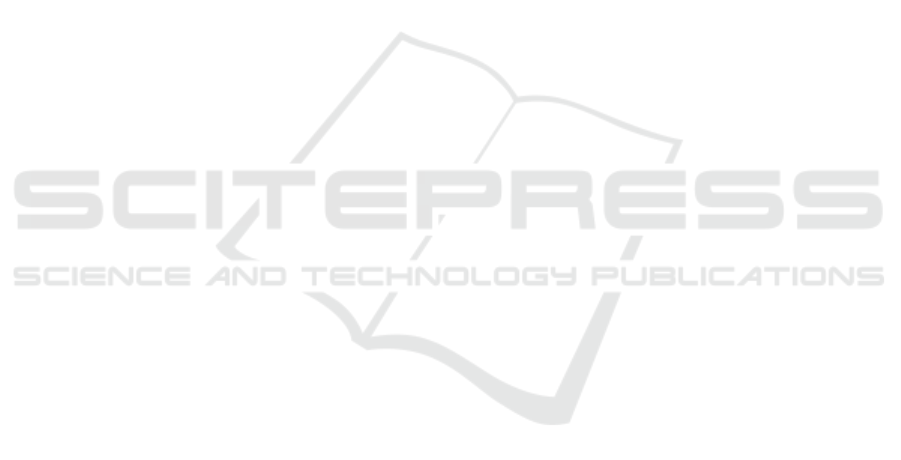
making use of these materials as “medium-level”
data to intermediately fine-tune deep neural
networks, we managed to make the deep neutral
networks acquire pathological knowledge step by
step following the way of pathologist’s perception.
By this mean, the initial task and the final target task
are expected to be bridged in a reasonable way. In
the experiments, our proposed scheme exerted
adequate efficacy for boosting the classification
performance and revealed high applicability for
different CNN architectures. Taking the proposed
scheme as seed, it is promising to promote such kind
of stepwise training scheme to more medical image
recognition tasks.
REFERENCES
Ferlay J, Soerjomataram I, Ervik M et al., 2013.
GLOBOCAN 2012 v1.0, Cancer Incidence and
Mortality Worldwide: IARC CancerBase, No. 11.
M. Fukayama et al., 2015. The Japanese Society of
Pathology Guideline 2015, The Japanese Society of
Pathology, pp. 6.
Toby C. Cornish, Global In-sourcing Using A Pathology
Teleconsultation Network Platform,
https://digitalpathologyassociation.org/_data/files/201
4_Pathology_Visions/PV14_Presentations/19C_In-
Sourcing_Workshop_Cornish.pdf, available on 13
Dec. 2018.
Abdelrahim N. Esgiar, R. Naguib and Bayan S. Sharif et
al., 1998, Microscopic Image Analysis for Quantitative
Measurement and Feature Identification of Normal and
Cancerous Colonic Mucosa, IEEE Transactions on
Information Technology in Biomedicine, vol. 2, no. 3,
pp. 197–203.
J. Diamond, N. H. Anderson, P. H. Bartels et al., 2004,
The Use of Morphological Characteristics and Texture
Analysis in the Identification of Tissue Composition in
Prostatic Neoplasia, Human Pathology, vol. 35, no. 9,
pp. 1121-1131.
K. Masood and N. Rajpoot, 2009, Texture Based
Classification of Hyperspectral Colon Biopsy Samples
Using CLBP, International Symposium on Biomedical
Imaging: From Nano to Macro, pp. 1011-1014.
Dinggang Shen, Guorong Wu and Heung-Il Suk, 2017,
Deep Learning in Medical Image Analysis, Annual
Review of Biomedical Engineering, no. 19, pp. 221–
248.
Raphaël Marée, 2017, The Need for Careful Data
Collection for Pattern Recognition in Digital
Pathology, Journal of Pathology Informatics. vol. 8,
no. 19.
Hao Chen, Xiaojuan Qi and Lequan Yu, 2016, DCAN:
Deep Contour-Aware Networks for Accurate Gland
Segmentation, IEEE Conference on Computer Vision
and Pattern Recognition, pp. 2487-2496.
B. Bejnordi, G. Litjens, N. Timofeeva et al., 2016, Stain
Specific Standardization of Whole-Slide
Histopathological Images, IEEE Transactions on
Medical Imaging, vol. 35, issue 2, pp. 404-415.
A. Krizhevsky, I. Sutskever and G. Hinton, 2012,
ImageNet Classification with Deep Convolutional
Neural Networks, 25th International Conference on
Neural Information Processing Systems, vol. 1, pp.
1097-1105.
Andrew Janowczyk and Anant Madabhushi, 2016, Deep
Learning for Digital Pathology Image Analysis: A
Comprehensive Tutorial With Selected Use Cases,
Journal of Pathology Informatics, vol. 7, no. 29.
Yan Xu, Zhipeng Jia, LiangBo Wang et al., 2017, Large
Scale Tissue Histopathology Image Classification,
Segmentation, and Visualization via Deep
Convolutional Activation Features, BMC
Bioinformatics, 18:281.
L. Hou, D. Samaras and TM. Kurc, 2016, Patch-based
Convolutional Neural Network for Whole Slide Tissue
Image Classification, IEEE Computer Society
Conference on Computer Vision and Pattern
Recognition, pp. 2424-2433.
Xu J, Luo X, Wang G et al., 2016, A Deep Convolutional
Neural Network for Segmenting and Classifying
Epithelial and Stromal Regions in Histopathological
Images, Neurocomputing, vol. 191, pp. 214-223.
F. Ciompi, O. Gessinnk, B. E. Bejnordi et al., 2017, The
Importance of Stain Normalization in Colorectal
Tissue Classification with Convolutional Networks,
IEEE International Symposium in Biomedical Imaging.
Manan Shah, Christopher Rubadue, David Suster et al.,
2016, Deep Learning Assessment of Tumor
Proliferation in Breast Cancer Histological Images,
arXiv:1610.03467.
H. Chen, Q. Dou, D. Ni et al., 2015, Automatic Fetal
Ultrasound Standard Plane Detectionusing Knowledge
Transferred Recurrent Neural Networks, International
Conference on Medical Image Computing and
Computer-Assisted Intervention, pp. 507-514.
Hoo Chang Shin, Holger R. Roth, Mingchen Gao et al.,
2016, Deep Convolutional Neural Networks for
Computer-Aided Detection: CNN Architectures,
Dataset Characteristics and Transfer Learning, IEEE
Transactions on Medical Imaging, vol. 35, issue 5, pp.
1285-1298.
J. Yosinski, J. Clune, Y. Bengio et al., 2014, How
Transferable Are Features in Deep Neural Networks?
Annual Conference on Neural Information Processing
Systems, pp. 3320–3328.
Anselm Brachmann, Erhardt Barth and Christoph Redies,
2017, Using CNN Features to Better Understand What
Makes Visual Artworks Special, Frontiers in
Psychology, 2017; 8: 830.
Aiga Suzuki, Satoshi Suzuki, Shoji Kido et al., 2017, A 2-
staged Transfer Learning Method with Deep
Convolutional Neural Network for Diffuse Lung
Disease Analysis, Proc. of the 2017 Intl. Forum on
Medical Imaging in Asia, pp. 160-163.
BIOIMAGING 2019 - 6th International Conference on Bioimaging
98