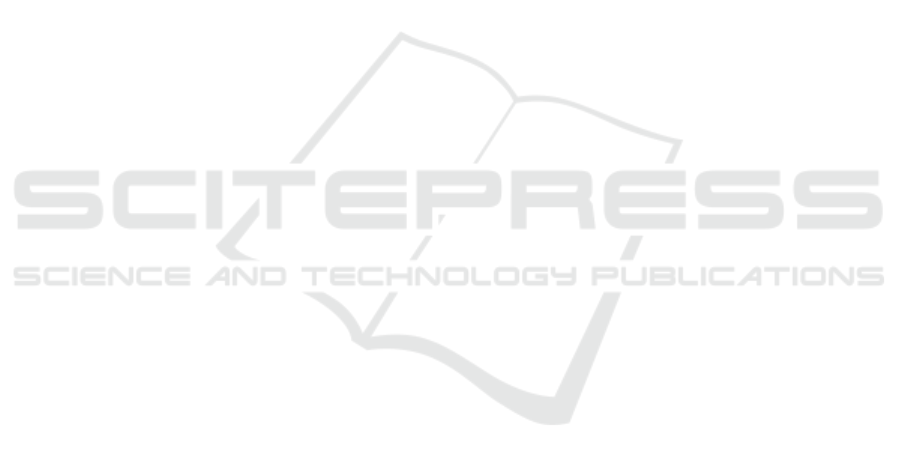
ble scale with that of more affluent studios. Wide-
baseline FVV systems are likely to always be more
susceptible to inherent noise in the form of occlusi-
ons and photogrammetry errors. While this noise pre-
sents a difficult obstacle we have shown that it is of-
ten temporally incoherent and so it can be corrected
by enforcing spatio-temporal constraints.
By leveraging the permanence of temporally co-
herent geometry, our system is able to effectively filter
noise while retaining pertinent geometric data which
has been lost on a frame to frame basis. By enforcing
this spatio-temporal consistency we demonstrate the
improvements that our system will have for modern
and future FVV systems alike.
We have shown that our system is suited to filte-
ring point clouds from both studio setups and hand-
held ”dynamic camera” outdoor scenes. Although the
effects are most appreciable for dynamic outdoor sce-
nes in which there tends to be much more noise, the
advantage of more accurate flow information demon-
strates visible improvements for indoor, studio-based
sequences also. Some inherent limitations exist in the
amount of noise which can be filtered whilst retaining
important geometry, as is typical of many signal-to-
noise filtering systems. This is particularly evident in
the case of fast moving objects but our system allevia-
tes this problem by using a dense optical flow method
with demonstrably good sensitivity to large displace-
ment as well as our proposed dynamic object tracking
constraint.
In comparison to temporally-naive geometric ups-
ampling approaches we can see that supplying spatio-
temporal information leads to more accurate results
and provides tighter framework for seeding geometric
upsampling processes. This is confirmed by the re-
sults obtained from the synthetic dataset test whereby
the most accurate approach was achieved by spatio-
temporal filtering of an edge-aware upsampled point
cloud.
ACKNOWLEDGMENTS
This publication has emanated from research con-
ducted with the financial support of Science Founda-
tion Ireland (SFI) under the Grant No. 15/RP/2776.
REFERENCES
Bao, L., Yang, Q., and Jin, H. (2014). Fast edge-preserving
patchmatch for large displacement optical flow. In
Proceedings of the IEEE Conference on Computer Vi-
sion and Pattern Recognition, pages 3534–3541.
Basha, T., Moses, Y., and Kiryati, N. (2013). Multi-view
scene flow estimation: A view centered variational
approach. International journal of computer vision,
101(1):6–21.
Berj
´
on, D., Pag
´
es, R., and Mor
´
an, F. (2016). Fast fe-
ature matching for detailed point cloud generation.
In Image Processing Theory Tools and Applications
(IPTA), 2016 6th International Conference on, pages
1–6. IEEE.
Bouguet, J.-Y. (2001). Pyramidal implementation of the af-
fine lucas-kanade feature tracker. Intel Corporation.
Collet, A., Chuang, M., Sweeney, P., Gillett, D., Evseev,
D., Calabrese, D., Hoppe, H., Kirk, A., and Sullivan,
S. (2015). High-quality streamable free-viewpoint vi-
deo. ACM Transactions on Graphics (ToG), 34(4):69.
Doll
´
ar, P. and Zitnick, C. L. (2013). Structured forests for
fast edge detection. In Computer Vision (ICCV), 2013
IEEE International Conference on, pages 1841–1848.
IEEE.
Farneb
¨
ack, G. (2003). Two-frame motion estimation based
on polynomial expansion. In Scandinavian conference
on Image analysis, pages 363–370. Springer.
Furukawa, Y. and Ponce, J. (2010). Accurate, dense, and ro-
bust multiview stereopsis. IEEE transactions on pat-
tern analysis and machine intelligence, 32(8):1362–
1376.
Gastal, E. S. and Oliveira, M. M. (2011). Domain transform
for edge-aware image and video processing. In ACM
Transactions on Graphics (ToG), volume 30, page 69.
ACM.
Hartley, R. I. and Zisserman, A. (2004). Multiple View Ge-
ometry in Computer Vision. Cambridge University
Press.
Hu, Y., Song, R., and Li, Y. (2016). Efficient coarse-to-
fine patchmatch for large displacement optical flow.
In Proceedings of the IEEE Conference on Computer
Vision and Pattern Recognition, pages 5704–5712.
Huang, C.-H., Boyer, E., Navab, N., and Ilic, S. (2014).
Human shape and pose tracking using keyframes. In
Proceedings of the IEEE Conference on Computer Vi-
sion and Pattern Recognition, pages 3446–3453.
Huang, H., Wu, S., Gong, M., Cohen-Or, D., Ascher, U.,
and Zhang, H. (2013). Edge-aware point set resam-
pling. ACM Transactions on Graphics, 32:9:1–9:12.
Kazhdan, M. and Hoppe, H. (2013). Screened poisson sur-
face reconstruction. ACM Transactions on Graphics
(ToG), 32(3):29.
Klaudiny, M., Budd, C., and Hilton, A. (2012). Towards
optimal non-rigid surface tracking. In European Con-
ference on Computer Vision, pages 743–756.
Lang, M., Wang, O., Aydin, T. O., Smolic, A., and
Gross, M. H. (2012). Practical temporal consistency
for image-based graphics applications. ACM Trans.
Graph., 31(4):34–1.
Li, H., Adams, B., Guibas, L. J., and Pauly, M. (2009). Ro-
bust single-view geometry and motion reconstruction.
In ACM Transactions on Graphics (ToG), volume 28,
page 175. ACM.
Liu, Y., Dai, Q., and Xu, W. (2010). A point-cloud-
based multiview stereo algorithm for free-viewpoint
Spatio-temporal Upsampling for Free Viewpoint Video Point Clouds
691