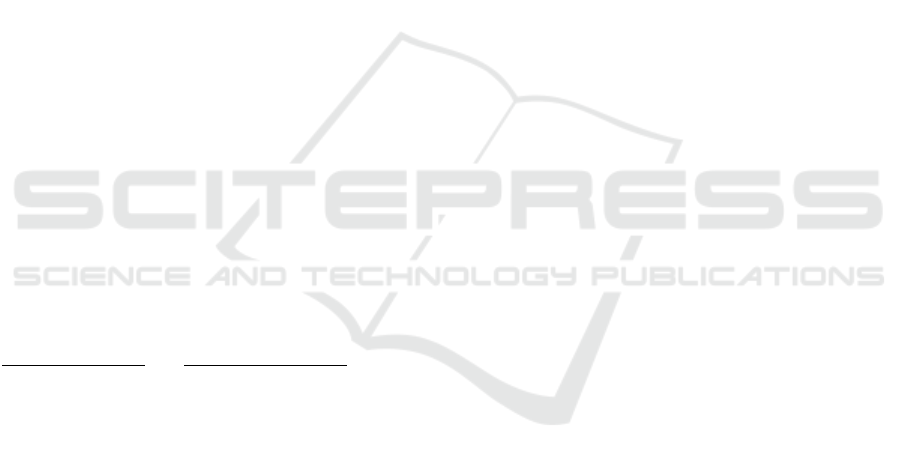
5 DISCUSSION
The increased accuracy of the predictions by using
data with more experimental evidence suggest that
the ncRNA-ncRNA interaction data with scarce
experimental support is not reliable enough to avoid
misclassifications. Concluding, therefore, in spite of
the overall good performances of our classification
approach, supplementing the ncRNA-ncRNA
interaction data with more experimental evidence will
aid in increasing the accuracy of the classification
workflow.
6 CONCLUSIONS
In this study we have tested different datasets to
study different types of non-coding RNA interactions
and the differences between those interactions. In our
experiments we have tested two main kind of
features, k-mers features and duplex features.
Interestingly we have discovered that using the k-
mers features is sufficient to distinguish between
different types of noncoding RNA interactions. We
didn’t observe any positive contribution of the duplex
features.
7 AVAILABILITY OF DATA AND
MATERIALS
All of the ncRNA data was obtained from
www.mirbase.org and starbase.sysu.edu.cn.
8 FUNDING
The work was supported by Zefat Academic College
to MY.
ACKNOWLEDGEMENTS
The work was supported by Zefat Academic College
to MY.
REFERENCES
Berthold, M. R. et al. (2008) ‘KNIME: The Konstanz
Information Miner’, in SIGKDD Explorations, pp. 319–
326. doi: 10.1007/978-3-540-78246-9_38.
Cakir, M. V. and Allmer, J. (2010) ‘Systematic
computational analysis of potential RNAi regulation in
Toxoplasma gondii’, in Health Informatics and
Bioinformatics (HIBIT), 2010 5th International
Symposium on. Ankara, Turkey: IEEE, pp. 31–38. doi:
10.1109/HIBIT.2010.5478909.
Childs L, Nikoloski Z, May P, Walther D. Identification
and classification of ncRNA molecules using graph
properties. Nucleic Acids Res. 2009;37(9):66.
Chiu HS, Somvanshi S, Patel E, Chen TW, Singh VP,
Zorman B, Patil SL, Pan Y, Chatterjee SS; Cancer
Genome Atlas Research Network, Sood AK, Gunaratne
PH, Sumazin P. Pan-Cancer Analysis of lncRNA
Regulation Supports Their Targeting of Cancer Genes
in Each Tumor Context. Cell Rep. 2018 Apr
3;23(1):297-312.e12. doi:
10.1016/j.celrep.2018.03.064.
Chou, C.-H. et al. (2018) ‘miRTarBase update 2018: a
resource for experimentally validated microRNA-target
interactions.’, Nucleic acids research, 46(D1), pp.
D296–D302. doi: 10.1093/nar/gkx1067.
Furió-Tarí P, Tarazona S, Gabaldón T, Enright AJ, Conesa
A. spongeScan: A web for detecting microRNA
binding elements in lncRNA sequences. Nucleic Acids
Res. 2016 Jul 8;44(W1):W176-80.
Griffiths-Jones, S. (2010) ‘miRBase: microRNA sequences
and annotation.’, Current protocols in bioinformatics /
editoral board, Andreas D. Baxevanis ... [et al.],
Chapter 12, p. Unit 12.9.1-10. doi:
10.1002/0471250953.bi1209s29.
Li, J. H. et al. (2014) ‘StarBase v2.0: Decoding miRNA-
ceRNA, miRNA-ncRNA and protein-RNA interaction
networks from large-scale CLIP-Seq data’, Nucleic
Acids Research. doi: 10.1093/nar/gkt1248.
Malik Yousef, Dalit Levy, Jens Allmer: Species
Categorization via MicroRNAs - Based on 3'UTR
Target Sites using Sequence Features.
BIOINFORMATICS 2018: 112-118
Matthews, B. W. (1975) ‘Comparison of the predicted and
observed secondary structure of T4 phage lysozyme’,
BBA - Protein Structure, 405(2), pp. 442–451. doi:
10.1016/0005-2795(75)90109-9.
Panwar B, Arora A, Raghava GP. Prediction and
classification of ncRNAs using structural information.
BMC Genomics. 2014;15(1):127.
Pertea M, Shumate A, Pertea G, Varabyou A, Chang Y,
Madugundu AK, Pandey A, Salzberg S (2018)
‘Thousands of large-scale RNA sequencing
experiments yield a comprehensive new human gene
list and reveal extensive transcriptional noise‘. bioRxiv
‘Sacar MD, Allmer J. Data mining for microrna gene
prediction: On the impact of class imbalance and
feature number for microrna gene prediction. 2013 8th
Int. Symp. Heal. Informatics Bioinforma.IEEE; 2013 p.
1--6.’ (no date).
Shaltout, N. A. N. et al. (2014) ‘Information gain as a
feature selection method for the efficient classification
of Influenza-A based on viral hosts’, in Proceedings of
the World Congress on Engineering. Newswood
Limited, pp. 625–631.
BIOINFORMATICS 2019 - 10th International Conference on Bioinformatics Models, Methods and Algorithms
172