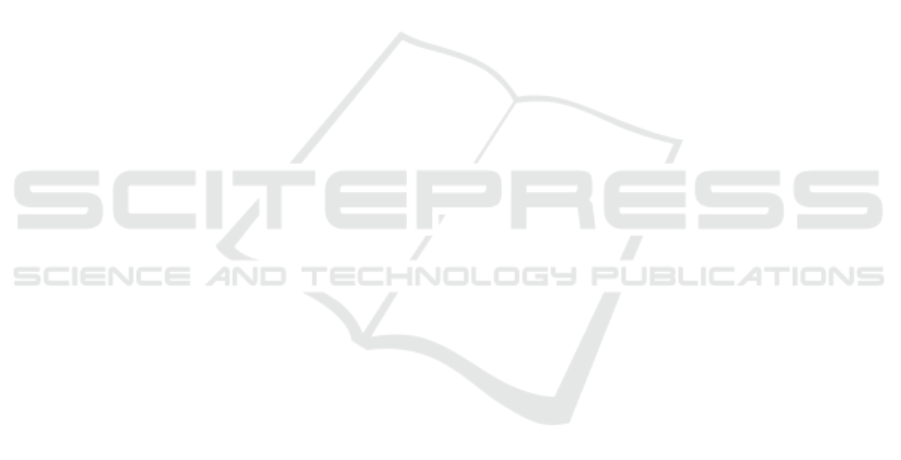
4.3.3 Overall Performances
We can observe that the Late Statistics combined with
the reduced hue histograms H30 outperform prior
works on the AFF, Trunk12 and BarkTex datasets.
LS-LCoLBP/H30 is in averaged 6.3% more accurate
than the methods compared in (Boudra et al., 2018).
Moreover, it is about 100 times smaller than SMBP*.
On NewBarkTex, Late Statistics combined with hue
histograms and a SVM classifier achieve similar re-
sults to Sandid16* with an accuracy of 82.0%. We
observe that our method (LS − LCoLBP/H30) obtai-
ned slightly lower results than the most accurate algo-
rithms from the literature on this dataset, but it does
have a significantly smaller feature vector which is
about 30 times smaller than Sandid16* and 100 times
smaller than Porebski18*. On Bark-101, we can ob-
serve the lowest accuracy rates for all compared met-
hods over all the datasets. These results are explained
by the higher number of classes in Bark-101 compa-
red to existing datasets. It also demonstrates the chal-
lenge proposed by Bark-101. However, most methods
including GWs/H30 achieved a top-1 recognition rate
about 30%, which is far above the random guess of
0.9%.
5 CONCLUSION
In this study, we compared recent state-of-the-art des-
criptors in the context of tree bark recognition in the
wild. We proposed two novel algorithms to reduce
the dimensionality of texture and color features vec-
tors. We showed that the proposed algorithms out-
perform state-of-the-art methods on four bark datasets
with a considerable gain in space complexity. We be-
lieve that these methods can be generalized on other
histogram-like feature vectors. Furthermore, we rele-
ased a new dataset made of 101 bark classes of seg-
mented images with high intra-class variability. We
demonstrated that the proposed dataset is particularly
challenging for existing methods, enforcing the need
for future prospects on bark recognition. Future work
will investigate the proposed methods as a lightweight
representation with multiple color spaces. We will
also evaluate the proposed algorithms on mobile plat-
forms, such as smartphones, to assess their perfor-
mances on real-world settings.
ACKNOWLEDGEMENT
This work is part of ReVeRIES project (Reconnais-
sance de V
´
eg
´
etaux R
´
ecr
´
eative, Int
´
eractive et Educa-
tive sur Smartphone) supported by the French Nati-
onal Agency for Research with the reference ANR-
15-CE38-004-01, and part of the French Environment
and Energy Management Agency, Grant TEZ17-42.
REFERENCES
Alice Porebski, Vinh Truong Hoang, N. V. D. H. (2018).
Multi-color space local binary pattern-based feature
selection for texture classification. Journal of Electro-
nic Imaging, 27:27 – 27 – 15.
Baki
´
c, V., Mouine, S., Ouertani-Litayem, S., Verroust-
Blondet, A., Yahiaoui, I., Go
¨
eau, H., and Joly, A.
(2013). Inria’s participation at imageclef 2013 plant
identification task. In CLEF Working Notes.
Bertrand, S., Ameur, R. B., Cerutti, G., Coquin, D., Valet,
L., and Tougne, L. (2018). Bark and leaf fusion sy-
stems to improve automatic tree species recognition.
Ecological Informatics.
Bertrand, S., Cerutti, G., and Tougne, L. (2017). Bark re-
cognition to improve leaf-based classification in di-
dactic tree species identification. In VISAPP.
Boudra, S., Yahiaoui, I., and Behloul, A. (2018). Plant iden-
tification from bark: A texture description based on
statistical macro binary pattern. In ICPR.
Cerutti, G., Tougne, L., Mille, J., Vacavant, A., and Coquin,
D. (2013). Understanding leaves in natural images - a
model-based approach for tree species identification.
Computer Vision and Image Understanding.
Go
¨
eau, H., Joly, A., Bonnet, P., Selmi, S., Molino, J.-
F., Barth
´
el
´
emy, D., and Boujemaa, N. (2014). Li-
feclef plant identification task 2014. In CLEF2014
Working Notes. Working Notes for CLEF 2014 Con-
ference, Sheffield, UK, September 15-18, 2014, pages
598–615. CEUR-WS.
Guo, Z., Zhang, L., and Zhang, D. (2010). A Completed
Modeling of Local Binary Pattern Operator for Tex-
ture Classification. IEEE TIP, 19(6).
Haralick, R. M., Shanmugam, K., and Dinstein, I. (1973).
Textural Features for Image Classification. IEEE
Transactions on Systems, Man, and Cybernetics.
Huang, Z.-K., Huang, D.-S., Du, J.-X., Quan, Z.-H., and
Guo, S.-B. (2006a). Bark classification based on gabor
filter features using rbpnn neural network. In Interna-
tional conference on neural information processing,
pages 80–87. Springer.
Huang, Z.-K., Zheng, C.-H., Du, J.-X., and Wan, Y.-y.
(2006b). Bark classification based on textural featu-
res using artificial neural networks. In International
Symposium on Neural Networks. Springer.
Junmin Wang, Yangyu Fan, N. L. (2017). Combining fine
texture and coarse color features for color texture clas-
sification. Journal of Electronic Imaging.
Lakmann, R. (1998). Barktex benchmark database of color
textured images. Koblenz-Landau University.
Ojala, T., Pietik
¨
ainen, M., and M
¨
aenp
¨
a
¨
a, T. (2001). A Gene-
ralized Local Binary Pattern Operator for Multiresolu-
tion Gray Scale and Rotation Invariant Texture Classi-
Efficient Bark Recognition in the Wild
247