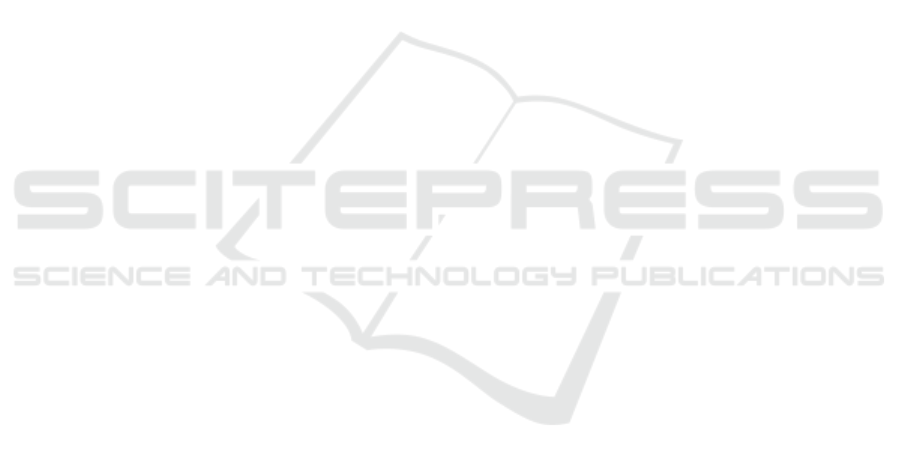
Armitage, A. & Keeble-Allen, D. Undertaking a structured
literature review or structuring a literature review: Tales
from the field. Proceedings of the 7th European
Conference on Research Methodology for Business and
Management Studies: ECRM2008, Regent's College,
London, 2008. 35.
Ash, J. 1997. Organizational Factors that Influence
Information Technology Diffusion in Academic Health
Sciences Centers. Journal of the American Medical
Informatics Association, 4, 102-111.
Bagozzi, R. P. 2007. The legacy of the technology
acceptance model and a proposal for a paradigm shift.
Journal of the association for information systems, 8, 3.
Baker, D., Bridges, D., Hunter, R., Johnson, G., Krupa, J.,
Murphy, J. & Sorenson, K. 2002. Guidebook to
decision-making methods. Department of Energy, USA.
Carroll, N., Travers, M. & Richardson, I. Connecting
Multistakeholder Analysis Across Connected Health
Solutions. International Joint Conference on
Biomedical Engineering Systems and Technologies,
2016. Springer, 319-339.
Chen, L.-D. & Tan, J. 2004. Technology Adaptation in E-
commerce:: Key Determinants of Virtual Stores
Acceptance. European Management Journal, 22, 74-86.
Davis, F. D. 1989. Perceived usefulness, perceived ease of
use, and user acceptance of information technology.
MIS Quarterly: Management Information Systems, 13,
319-339.
Dedrick, J. & West, J. Why firms adopt open source
platforms: a grounded theory of innovation and
standards adoption. Proceedings of the workshop on
standard making: A critical research frontier for
information systems, 2003. 236-257.
Fishbein, M. & Ajzen, I. 1975. Belief, attitude, intention and
behavior: An introduction to theory and research.
Gelijns, A. C. 1992. Technology and health care in an era of
limits, National Academies Press.
Goodhue, D. L. 1998. Development and measurement
validity of a task‐ technology fit instrument for user
evaluations of information system. Decision sciences,
29, 105-138.
Green, L. W. & Kreuter, M. W. 1999. Health promotion
planning: An educational and ecological approach,
Mayfield publishing company Mountain View, CA.
Hsiao, S.-J., Li, Y.-C., Chen, Y.-L. & Ko, H.-C. 2009.
Critical factors for the adoption of mobile nursing
information systems in Taiwan: the nursing department
administrators’ perspective. Journal of medical systems,
33, 369.
Hsu, P.-F., Kraemer, K. L. & Dunkle, D. 2006.
Determinants of e-business use in US firms.
International Journal of Electronic Commerce, 10, 9-45.
Kim, S., Lee, K., Hwang, H. & Yoo, S. 2016. Analysis of
the factors influencing healthcare professionals'
adoption of mobile electronic medical record (EMR)
using the unified theory of acceptance and use of
technology (UTAUT) in a tertiary hospital. BMC
medical informatics and decision making, 16, 12-12.
Kuziemsky, C., Abbas, R. M. & Carroll, N. Toward a
Connected Health Delivery Framework. 2018
IEEE/ACM International Workshop on Software
Engineering in Healthcare Systems (SEHS), 2018.
IEEE, 46-49.
Liberati, A., Altman, D. G., Tetzlaff, J., Mulrow, C.,
Gøtzsche, P. C., Ioannidis, J. P., Clarke, M., Devereaux,
P. J., Kleijnen, J. & Moher, D. 2009. The PRISMA
statement for reporting systematic reviews and meta-
analyses of studies that evaluate health care
interventions: explanation and elaboration. PLoS
medicine, 6, e1000100.
Lippeveld, T., Sauerborn, R. & Bodart, C. 2000. Design and
implementation of health information systems, Citeseer.
Maillet, É., Mathieu, L. & Sicotte, C. 2015. Modeling
factors explaining the acceptance, actual use and
satisfaction of nurses using an Electronic Patient Record
in acute care settings: An extension of the UTAUT.
International journal of medical informatics, 84, 36-47.
Menachemi, N., Burke, D. E. & Ayers, D. J. 2004. Factors
affecting the adoption of telemedicine—a multiple
adopter perspective. Journal of medical systems, 28,
617-632.
Menachemi, N., Matthews, M., Ford, E. W., Hikmet, N. &
Brooks, R. G. 2009. The relationship between local
hospital IT capabilities and physician EMR adoption.
Journal of medical systems, 33, 329.
Pearlson, K. & Saunders, C. 2006. Managing and Using
Information Systems: A Strategic Approach.
Petticrew, M. 2001. Systematic reviews from astronomy to
zoology: myths and misconceptions. Bmj, 322, 98-101.
Rippen, H. E., Pan, E. C., Russell, C., Byrne, C. M. & Swift,
E. K. 2013. Organizational framework for health
information technology. International Journal of
Medical Informatics, 82, e1-e13.
Sulaiman, H. & Wickramasinghe, N. 2014. Assimilating
Healthcare Information Systems in a Malaysian
Hospital. CAIS, 34, 77.
Teplensky, J. D., Pauly, M. V., Kimberly, J. R., Hillman, A.
L. & Schwartz, J. S. 1995. Hospital adoption of medical
technology: an empirical test of alternative models.
Health services research, 30, 437.
Tornatzky, L. & Fleischer, M. 1990. The Processes of
Technological Innovation AK Chakrabarti, ed.
Lexington Books. Available at: http://books. google.
com. hk/books.
Venkatesh, V. & Bala, H. 2008. Technology acceptance
model 3 and a research agenda on interventions.
Decision sciences, 39, 273-315.
Venkatesh, V., Morris, M. G., Davis, G. B. & Davis, F. D.
2003. User acceptance of information technology:
Toward a unified view. MIS quarterly, 425-478.
Wang, Y.-M., Wang, Y.-S. & Yang, Y.-F. 2010.
Understanding the determinants of RFID adoption in the
manufacturing industry. Technological forecasting and
social change, 77, 803-815.
Yang, Z., Kankanhalli, A., Ng, B.-Y. & Lim, J. T. Y. 2013.
Analyzing the enabling factors for the organizational
decision to adopt healthcare information systems.
Decision Support Systems, 55, 764-776.
Yusof, M. M., Kuljis, J., Papazafeiropoulou, A. &
Stergioulas, L. K. 2008. An evaluation framework for
HEALTHINF 2019 - 12th International Conference on Health Informatics
246