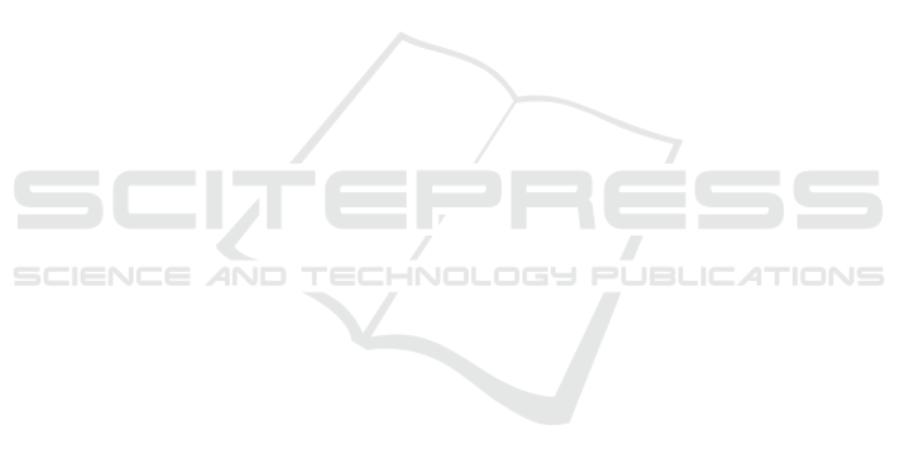
ANS (Hoyer et al., 2005) respectively. As a matter of
fact, the sympathetic branch intervenes on a slower
time scale, but its effect last longer in the target
system, whereas the parasympathetic has a punctual
yet rapidly vanishing action. Given these
considerations, it is possible to speculate that that the
vagal system is possibly more implicated in the
directionality from RESP to RR while the
sympathetic in the opposite directionality. The latter
assumption is based on the behavior of TE
RR→RESP
showing higher TE values for intermediate lags up to
the point of reaching almost comparable values to the
maximum information transfer for TE
RESP→RR
.
In sum, we have provided a noninvasive
characterization of the sleep state effects on
cardiorespiratory regulation in newborns, by
employing a measure of TE capable of quantifying
both linear and nonlinear aspect of the
interrelationship between the cardiac and respiratory
system. The novel approach of lagged TE was
capable of highlighting interactions occurring on
different time scales and possibly related to the
activation of the two autonomic branches. The
proposed interpretation of TE related findings is also
consistent with previous works, modelling the
interrelationship between the two branches of ANS
and cardiorespiratory regulation (Ataee et al., 2012).
In the specific context of this work, information flow
between HR and respiration is increased in QS with
respect to AS, indicating that in such sleep state more
information is exchanged between the cardiac and the
respiratory systems on average. This approach to the
quantification of cardiorespiratory interactions
affords the potential for early assessment of infant
development of bidirectional control between
physiological systems providing a more
comprehensive framework of investigation rather
than studying the two signals on their own.
Lastly, the proposed approach could facilitate
early risk assessment for neurophysiological
disorders such as Sudden Infant Death Syndrome
(SIDS) by tracking adverse profile of information
exchange between the two systems, thus exposing
infants to greater risk for a variety of pathology
involving the malfunction of cardiorespiratory
controlling mechanism.
ACKNOWLEDGEMENTS
The writing of this manuscript was supported by the
Sackler Institute of Developmental Psychobiology at
Columbia University and by National Institute of
Health grants NIH Grants R37 HD32774 (WPF) and
T32 MH018264 (NB). This publication was also
supported by the National Center for Advancing
Translational Sciences, National Institutes of Health,
through Grant Number UL1TR001873. The content
is solely the responsibility of the authors and does not
necessarily represent the official views of the NIH.
REFERENCES
Ataee, P., Belingard, L., Dumont, G.A., Noubari, H.A.,
Boyce, W.T., 2012. Autonomic-cardiorespiratory
regulation: A physiology-based mathematical model.
Proc. Annu. Int. Conf. IEEE Eng. Med. Biol. Soc. EMBS
3805–3808.
Berntson, G.G., Cacioppo, J.T., Quigley, K.S., 1993.
Respiratory sinus arrhythmia: Autonomic origins,
physiological mechanisms, and psychophysiological
implications. Psychophysiology 30, 183–196.
Caţaron, A., Andonie, R., 2018. Transfer Information
Energy: A Quantitative Indicator of Information
Transfer between Time Series. Entropy 20, 323.
Faes, L., Marinazzo, D., Montalto, A., Nollo, G., 2014.
Lag-specific transfer entropy as a tool to assess
cardiovascular and cardiorespiratory information
transfer. IEEE Trans. Biomed. Eng. 61, 2556–2568.
Faes, L., Montalto, A., Nollo, G., Marinazzo, D., 2013a.
Information decomposition of short-term
cardiovascular and cardiorespiratory variability.
Comput. Cardiol. (2010). 40, 113–116.
Faes, L., Nollo, G., Porta, A., 2011. Information domain
approach to the investigation of cardio-vascular, cardio-
pulmonary, and vasculo-pulmonary causal couplings.
Front. Physiol. 2.
Faes, L., Nollo, G., Porta, A., 2012. Non-uniform
multivariate embedding to assess the information
transfer in cardiovascular and cardiorespiratory
variability series. Comput. Biol. Med. 42, 290–297.
Faes, L., Nollo, G., Porta, A., 2013b. Compensated transfer
entropy as a tool for reliably estimating information
transfer in physiological time series. Entropy 15, 198–
219.
Farrell, T.G., Bashir, Y., Cripps, T., Malik, M., Poloniecki,
J., Bennett, E.D., Ward, D.E., Camm, A.J., 1991. Risk
stratification for arrhythmic events in postinfarction
patients based on heart rate variability, ambulatory
electrocardiographic variables and the signal-averaged
electrocardiogram. J. Am. Coll. Cardiol. 18, 687–697.
Frasch, M.G., Zwiener, U., Hoyer, D., Eiselt, M., 2007.
Autonomic organization of respirocardial function in
healthy human neonates in quiet and active sleep. Early
Hum. Dev. 83, 269–277.
Galland, B.C., Hayman, R.M., Taylor, B.J., Bolton, D.P.G.,
Sayers, R.M., Williams, S.M., 2000. Factors Affecting
Heart Rate Variability and Heart Rate Responses to
Tilting in Infants Aged 1 and 3 Months. Pediatr. Res.
48, 360–368.
Harper, R.M., Hoppenbrouwers, T., Sterman, M.B.,
McGinty, D.J., Hodgman, J., 1976. Polygraphic studies
Lagged Transfer Entropy Analysis to Investigate Cardiorespiratory Regulation in Newborns during Sleep
145