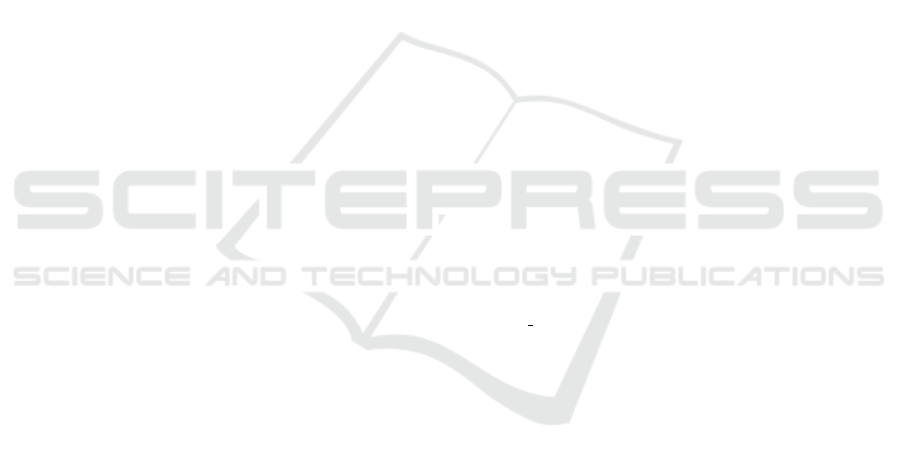
Dai, W., Doyle, J., Liang, X., Zhang, H., Dong, N., Li, Y.,
and Xing, E. P. (2017). SCAN: structure correcting
adversarial network for chest x-rays organ segmenta-
tion. CoRR, abs/1703.08770.
Dar, S. U. H., Yurt, M., Karacan, L., Erdem, A., Erdem,
E., and C¸ ukur, T. (2018). Image synthesis in multi-
contrast MRI with conditional generative adversarial
networks. CoRR, abs/1802.01221.
Frangi, A. F., Tsaftaris, S. A., and Prince, J. L. (2018).
Simulation and synthesis in medical imaging. IEEE
Transactions on Medical Imaging, 37(3):673–679.
Frid-Adar, M., Diamant, I., Klang, E., Amitai, M., Gold-
berger, J., and Greenspan, H. (2018). Gan-based
synthetic medical image augmentation for increased
CNN performance in liver lesion classification. CoRR,
abs/1803.01229.
Goodfellow, I., Pouget-Abadie, J., Mirza, M., Xu, B.,
Warde-Farley, D., Ozair, S., Courville, A., and Ben-
gio, Y. (2014). Generative adversarial nets. In Advan-
ces in neural information processing systems, pages
2672–2680.
Gulrajani, I., Ahmed, F., Arjovsky, M., Dumoulin, V., and
Courville, A. C. (2017). Improved training of wasser-
stein gans. CoRR, abs/1704.00028.
He, K., Zhang, X., Ren, S., and Sun, J. (2016). Deep resi-
dual learning for image recognition. In Proceedings of
the IEEE conference on computer vision and pattern
recognition, pages 770–778.
Hussain, Z., Gimenez, F., Yi, D., and Rubin, D. (2017).
Differential data augmentation techniques for medical
imaging classification tasks. In AMIA Annual Sympo-
sium Proceedings, volume 2017, page 979. American
Medical Informatics Association.
Jack Jr, C. R., Bernstein, M. A., Fox, N. C., Thompson,
P., Alexander, G., Harvey, D., Borowski, B., Britson,
P. J., L. Whitwell, J., Ward, C., et al. (2008). The alz-
heimer’s disease neuroimaging initiative (adni): Mri
methods. Journal of Magnetic Resonance Imaging:
An Official Journal of the International Society for
Magnetic Resonance in Medicine, 27(4):685–691.
Karras, T., Aila, T., Laine, S., and Lehtinen, J. (2017). Pro-
gressive growing of gans for improved quality, stabi-
lity, and variation. CoRR, abs/1710.10196.
Kingma, D. P. and Ba, J. (2014). Adam: A method for
stochastic optimization. CoRR, abs/1412.6980.
Kuka
ˇ
cka, J., Golkov, V., and Cremers, D. (2018). Regulari-
zation for deep learning: A taxonomy.
LeCun, Y. A., Bottou, L., Orr, G. B., and M
¨
uller, K.-
R. (1998). Efficient backprop. In Neural networks:
Tricks of the trade, pages 9–48. Springer.
Neff, T. (2018). Data augmentation in deep learning using
generative adversarial networks. Master’s thesis, Graz
University of Technology, Graz, Austria,.
Odena, A., Olah, C., and Shlens, J. (2016). Conditional
image synthesis with auxiliary classifier gans. arXiv
preprint arXiv:1610.09585.
Perez, L. and Wang, J. (2017). The effectiveness of data
augmentation in image classification using deep lear-
ning. CoRR, abs/1712.04621.
Petersen, R. C., Aisen, P., Beckett, L., Donohue, M., Gamst,
A., Harvey, D., Jack, C., Jagust, W., Shaw, L., Toga,
A., et al. (2010). Alzheimer’s disease neuroimaging
initiative (adni) clinical characterization. Neurology,
74(3):201–209.
Russakovsky, O., Deng, J., Su, H., Krause, J., Satheesh,
S., Ma, S., Huang, Z., Karpathy, A., Khosla, A.,
Bernstein, M., Berg, A. C., and Fei-Fei, L. (2015).
ImageNet Large Scale Visual Recognition Challenge.
International Journal of Computer Vision (IJCV),
115(3):211–252.
Shaban, M. T., Baur, C., Navab, N., and Albarqouni, S.
(2018). Staingan: Stain style transfer for digital histo-
logical images. CoRR, abs/1804.01601.
Shin, H.-C., Tenenholtz, N. A., Rogers, J. K., Schwarz,
C. G., Senjem, M. L., Gunter, J. L., Andriole, K., and
Michalski, M. (2018). Medical Image Synthesis for
Data Augmentation and Anonymization using Gene-
rative Adversarial Networks. ArXiv e-prints.
Srivastava, N., Hinton, G., Krizhevsky, A., Sutskever, I.,
and Salakhutdinov, R. (2014). Dropout: a simple way
to prevent neural networks from overfitting. The Jour-
nal of Machine Learning Research, 15(1):1929–1958.
Vasconcelos, C. N. and Vasconcelos, B. N. (2017). Incre-
asing deep learning melanoma classification by clas-
sical and expert knowledge based image transforms.
CoRR, abs/1702.07025.
Wang, Y., Girshick, R. B., Hebert, M., and Hariharan,
B. (2018). Low-shot learning from imaginary data.
CoRR, abs/1801.05401.
Wu, R., Yan, S., Shan, Y., Dang, Q., and Sun, G. (2015).
Deep image: Scaling up image recognition. arXiv pre-
print arXiv:1501.02876.
Xue, Y., Xu, T., Zhang, H., Long, L. R., and Huang, X.
(2017). Segan: Adversarial network with multi-scale
$l
1$ loss for medical image segmentation. CoRR,
abs/1706.01805.
Yi, X., Walia, E., and Babyn, P. (2018). Generative Adver-
sarial Network in Medical Imaging: A Review. ArXiv
e-prints.
Generative Adversarial Networks as an Advanced Data Augmentation Technique for MRI Data
59