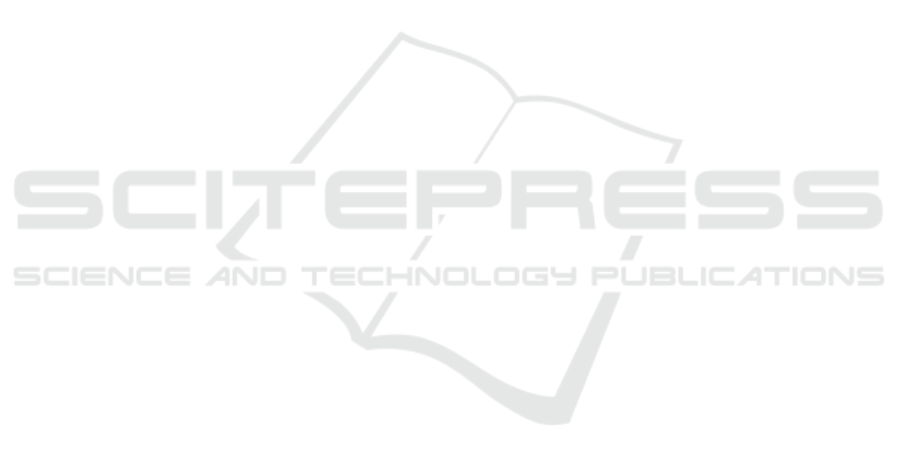
Ghoniem, M., Fekete, J.-D., and Castagliola, P. (2004). A
comparison of the readability of graphs using node-
link and matrix-based representations. In IEEE Symp.
on Information Visualization, INFOVIS, pages 17–24.
Goebel, M. and Gruenwald, L. (1999). A survey of
data mining and knowledge discovery software tools.
SIGKDD Explor. Newsl., 1(1):20–33.
Guo, D. (2009). Flow mapping and multivariate visualiza-
tion of large spatial interaction data. IEEE Transacti-
ons on Visualization and Computer Graphics, 15(6).
Heinke, D. and Hamker, F. H. (1998). Comparing neural
networks: a benchmark on growing neural gas, gro-
wing cell structures, and fuzzy artmap. IEEE Tran-
sactions on Neural Networks, 9(6):1279–1291.
Heinrich, J. and Weiskopf, D. (2013). State-of-the-art of
parallel coordinates. In Eurographics (STARs), pages
95–116.
Hoffman, P., Grinstein, G., Marx, K., Grosse, I., and Stan-
ley, E. (1997). Dna visual and analytic data mi-
ning. In Proceedings. Visualization ’97 (Cat. No.
97CB36155), pages 437–441.
Holten, D. and van Wijk, J. J. (2009). A user study on visu-
alizing directed edges in graphs. In Proceedings of the
SIGCHI Conference on Human Factors in Computing
Systems, pages 2299–2308. ACM.
Holzinger, A. (2016). Interactive machine learning for he-
alth informatics: when do we need the human-in-the-
loop? Brain Informatics, 3(2):119–131.
Hotelling, H. (1933). Analysis of a complex of statistical
variables into principal components. Journal of edu-
cational psychology, 24(6):417.
Inselberg, A. (2009). Parallel coordinates. In Encyclopedia
of Database Systems, pages 2018–2024. Springer.
Inselberg, A. and Dimsdale, B. (1990). Parallel coordina-
tes: A tool for visualizing multi-dimensional geome-
try. In Proc. of the 1st Conf. on Visualization, pages
361–378, Los Alamitos, CA, USA. IEEE Computer
Society Press.
Johansson, J. and Forsell, C. (2016). Evaluation of parallel
coordinates: Overview, categorization and guidelines
for future research. IEEE Transactions on Visualiza-
tion and Computer Graphics, 22(1):579–588.
Kandogan, E. (2000). Star coordinates: A multi-
dimensional visualization technique with uniform tre-
atment of dimensions. In Proceedings of the IEEE
Information Visualization Symposium, volume 650,
page 22.
Kohonen, T. (1997). Self-Organizing Maps. Springer series
in information sciences. Berlin: Springer, 2 edition.
Lu, Y., Garcia, R., Hansen, B., Gleicher, M., and Maciejew-
ski, R. (2017). The state-of-the-art in predictive visual
analytics. In Computer Graphics Forum, volume 36,
pages 539–562. Wiley Online Library.
Maaten, L. v. d. and Hinton, G. (2008). Visualizing data
using t-sne. Journal of machine learning research,
9(Nov):2579–2605.
M
¨
uhlbacher, T., Piringer, H., Gratzl, S., Sedlmair, M., and
Streit, M. (2014). Opening the black box: Strategies
for increased user involvement in existing algorithm
implementations. IEEE Transactions on Visualization
and Computer Graphics, 20(12):1643–1652.
Mulder, J. D., van Wijk, J. J., and van Liere, R. (1999). A
survey of computational steering environments. Fu-
ture Generation Computer Systems, 15(1):119 – 129.
Munzner, T. (2014). Visualization analysis and design.
CRC Press.
Palomo, E. J. and L
´
opez-Rubio, E. (2017). The gro-
wing hierarchical neural gas self-organizing neural
network. IEEE Transactions on Neural Networks and
Learning Systems, 28(9):2000–2009.
Patton, M. Q. (2005). Qualitative research. Wiley Online
Library.
Prudent, Y. and Ennaji, A. (2005). An incremental growing
neural gas learns topologies. In Proc. IEEE Int. Joint
Conf. on Neural Networks, volume 2, pages 1211–
1216.
Ribeiro, M. T., Singh, S., and Guestrin, C. (2016). ”Why
should i trust you?”: Explaining the predictions of any
classifier. In Proc. of the 22nd ACM SIGKDD Int.
Conf. on Knowledge Discovery and Data Mining, pa-
ges 1135–1144, New York, NY, USA. ACM.
Riveiro, M., Falkman, G., and Ziemke, T. (2008). Impro-
ving maritime anomaly detection and situation awa-
reness through interactive visualization. In 11th Int.
Conf. on Information Fusion, pages 1–8. IEEE.
Schreck, T., Bernard, J., Von Landesberger, T., and Kohl-
hammer, J. (2009). Visual cluster analysis of trajec-
tory data with interactive kohonen maps. Information
Visualization, 8(1):14–29.
Sledge, I. J. and Keller, J. M. (2008). Growing neural gas
for temporal clustering. In Pattern Recognition, 2008.
ICPR 2008. 19th International Conference on, pages
1–4. IEEE.
Spence, I. and Efendov, A. (2001). Target detection in
scientific visualization. Journal of Experimental Psy-
chology: Applied, 7(1):13–26.
Spence, R. (2007). Information Visualization: Design for
Interaction. Pearson/Prentice Hall.
Tukey, J. W. (1977). Exploratory data analysis, volume 2.
Reading, Mass.
Van Ham, F. (2003). Using multilevel call matrices in large
software projects. In IEEE Symposium on Information
Visualization, INFOVIS 2003, pages 227–232. IEEE.
van Leeuwen, M. (2014). Interactive data exploration using
pattern mining, pages 169–182. Springer Berlin Hei-
delberg, Berlin, Heidelberg.
Vanetti, M., Gallo, I., and Nodari, A. (2013). Unsupervi-
sed feature learning using self-organizing maps. In
Proceedings of the International Conference on Com-
puter Vision Theory and Applications - Volume 1: VI-
SAPP, (VISIGRAPP 2013), pages 596–601. INSTICC,
SciTePress.
Ventocilla, E., Helldin, T., Riveiro, M., Bae, J., Boeva, V.,
Falkman, G., and Lavesson, N. (2018). Towards a
taxonomy for interpretable and interactive machine le-
arning. In IJCAI/ECAI. Proc. of the 2nd Workshop
on Explainable Artificial Intelligence (XAI-18), pages
151–157.
IVAPP 2019 - 10th International Conference on Information Visualization Theory and Applications
70