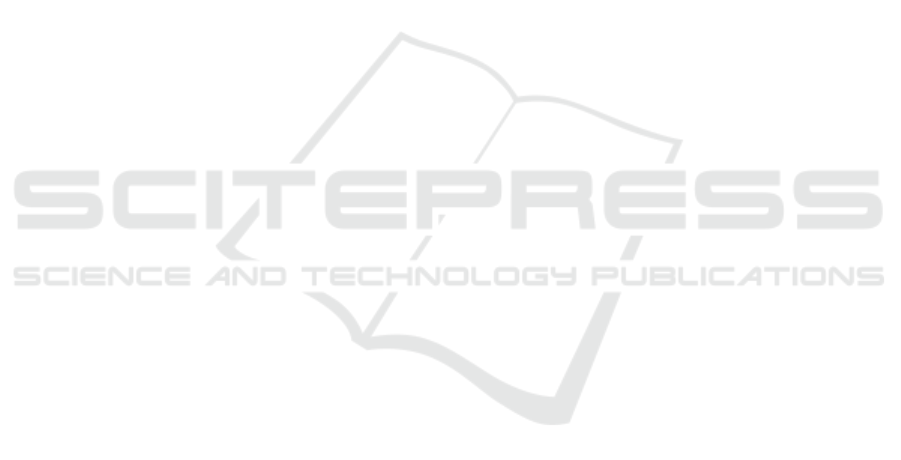
onally rely on image priors such as pre-trained net-
works.
REFERENCES
An, J. and Cho, S. (2015). Variational Autoencoder based
Anomaly Detection using Reconstruction Probability.
SNU Data Mining Center, Tech. Rep.
Arjovsky, M. and Bottou, L. (2017). Towards Principled
Methods for Training Generative Adversarial Net-
works. International Conference on Learning Repre-
sentations.
Baur, C., Wiestler, B., Albarqouni, S., and Navab, N.
(2018). Deep Autoencoding Models for Unsupervised
Anomaly Segmentation in Brain MR Images. arXiv
preprint arXiv:1804.04488.
Boracchi, G., Carrera, D., and Wohlberg, B. (2014). No-
velty Detection in Images by Sparse Representations.
In 2014 IEEE Symposium on Intelligent Embedded Sy-
stems (IES), pages 47–54. IEEE.
Carrera, D., Boracchi, G., Foi, A., and Wohlberg, B.
(2015). Detecting anomalous structures by convo-
lutional sparse models. In 2015 International Joint
Conference on Neural Networks (IJCNN), pages 1–8.
IEEE.
Carrera, D., Boracchi, G., Foi, A., and Wohlberg, B.
(2016). Scale-invariant anomaly detection with mul-
tiscale group-sparse models. In 2016 IEEE Interna-
tional Conference on Image Processing (ICIP), pages
3892–3896. IEEE.
Carrera, D., Manganini, F., Boracchi, G., and Lanzarone,
E. (2017). Defect Detection in SEM Images of Na-
nofibrous Materials. IEEE Transactions on Industrial
Informatics, 13(2):551–561.
Donahue, J., Kr
¨
ahenb
¨
uhl, P., and Darrell, T. (2017). Adver-
sarial Feature Learning. International Conference on
Learning Representations.
Dosovitskiy, A. and Brox, T. (2016). Generating Ima-
ges with Perceptual Similarity Metrics based on Deep
Networks. In Advances in Neural Information Proces-
sing Systems, pages 658–666.
Goodfellow, I., Bengio, Y., and Courville, A. (2016). Deep
Learning. MIT Press.
Goodfellow, I., Pouget-Abadie, J., Mirza, M., Xu, B.,
Warde-Farley, D., Ozair, S., Courville, A., and Ben-
gio, Y. (2014). Generative Adversarial Nets. In Ad-
vances in Neural Information Processing Systems, pa-
ges 2672–2680.
Kingma, D. P. and Ba, J. (2015). Adam: A Method for
Stochastic Optimization. International Conference on
Learning Representations.
Kingma, D. P. and Welling, M. (2014). Auto-Encoding Va-
riational Bayes. International Conference on Lear-
ning Representations.
Krizhevsky, A., Sutskever, I., and Hinton, G. E. (2012).
ImageNet Classification With Deep Convolutional
Neural Networks. In Advances in Neural Information
Processing Systems, pages 1097–1105.
Napoletano, P., Piccoli, F., and Schettini, R. (2018). Ano-
maly Detection in Nanofibrous Materials by CNN-
Based Self-Similarity. Sensors, 18(1):209.
Perera, P. and Patel, V. M. (2018). Learning Deep Fe-
atures for One-Class Classification. arXiv preprint
arXiv:1801.05365.
Pimentel, M. A., Clifton, D. A., Clifton, L., and Tarassenko,
L. (2014). A review of novelty detection. Signal Pro-
cessing, 99:215–249.
Ridgeway, K., Snell, J., Roads, B., Zemel, R. S., and
Mozer, M. C. (2015). Learning to generate ima-
ges with perceptual similarity metrics. arXiv preprint
arXiv:1511.06409.
Russakovsky, O., Deng, J., Su, H., Krause, J., Satheesh, S.,
Ma, S., Huang, Z., Karpathy, A., Khosla, A., Bern-
stein, M., et al. (2015). ImageNet Large Scale Vi-
sual Recognition Challenge. International Journal of
Computer Vision, 115(3):211–252.
Sabokrou, M., Khalooei, M., Fathy, M., and Adeli, E.
(2018). Adversarially Learned One-Class Classifier
for Novelty Detection. In Proceedings of the IEEE
Conference on Computer Vision and Pattern Recogni-
tion, pages 3379–3388.
Schlegl, T., Seeb
¨
ock, P., Waldstein, S. M., Schmidt-Erfurth,
U., and Langs, G. (2017). Unsupervised Anomaly
Detection with Generative Adversarial Networks to
Guide Marker Discovery. In International Conference
on Information Processing in Medical Imaging, pages
146–157. Springer.
Soukup, D. and Pinetz, T. (2018). Reliably Decoding
Autoencoders’ Latent Spaces for One-Class Learning
Image Inspection Scenarios. In OAGM Workshop
2018. Verlag der Technischen Universit
¨
at Graz.
Steger, C., Ulrich, M., and Wiedemann, C. (2018). Ma-
chine Vision Algorithms and Applications. Wiley-
VCH, Weinheim, 2nd edition.
Vasilev, A., Golkov, V., Lipp, I., Sgarlata, E., Tomassini, V.,
Jones, D. K., and Cremers, D. (2018). q-Space No-
velty Detection with Variational Autoencoders. arXiv
preprint arXiv:1806.02997.
Wang, Z., Bovik, A. C., Sheikh, H. R., and Simoncelli, E. P.
(2004). Image quality assessment: from error visi-
bility to structural similarity. IEEE transactions on
image processing, 13(4):600–612.
Wang, Z., Simoncelli, E. P., and Bovik, A. C. (2003). Mul-
tiscale structural similarity for image quality asses-
sment. In Record of the Thirty-Seventh Asilomar Con-
ference on Signals, Systems and Computers, volume 2,
pages 1398–1402. Ieee.
Zenati, H., Foo, C. S., Lecouat, B., Manek, G.,
and Chandrasekhar, V. R. (2018). Efficient
GAN-Based Anomaly Detection. arXiv preprint
arXiv:1802.06222.
VISAPP 2019 - 14th International Conference on Computer Vision Theory and Applications
380