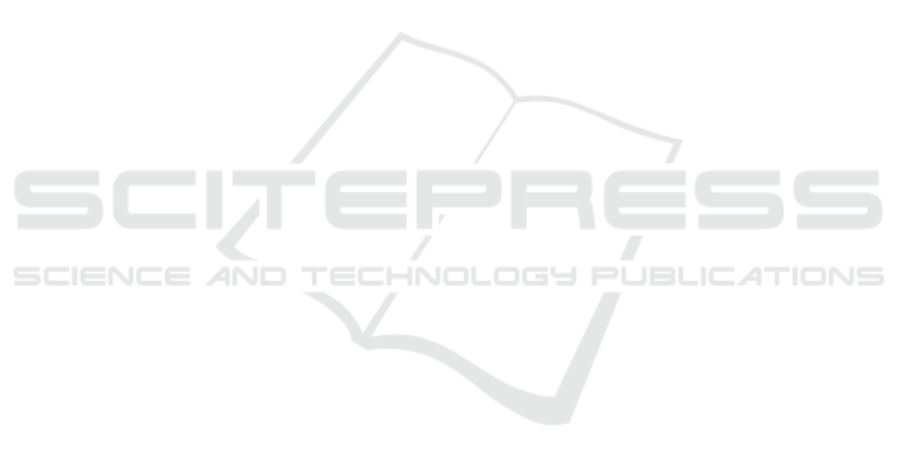
in several training and test frames. Experiments show
that the two methods achieve complementary perfor-
mance, which suggests that more improvement can be
obtained by combining the two approaches. Future
works will focus on integrating the two approaches to
improve point of interest recognition results.
ACKNOWLEDGEMENTS
This research is supported by PON MISE - Horizon
2020, Project VEDI - Vision Exploitation for Data
Interpretation, Prog. n. F/050457/02/X32 - CUP:
B68I17000800008 - COR: 128032, and Piano della
Ricerca 2016-2018 linea di Intervento 2 of DMI of
the University of Catania. The authors would like to
thank Francesca Del Zoppo and Lucia Cacciola for
the support in the labeling of the UNICT-VEDI data-
set.
REFERENCES
Ahmetovic, D., Gleason, C., Kitani, K. M., Takagi, H., and
Asakawa, C. (2016). Navcog: Turn-by-turn smartp-
hone navigation assistant for people with visual im-
pairments or blindness. In Proceedings of the 13th
Web for All Conference, W4A ’16, pages 9:1–9:2,
New York, NY, USA. ACM.
Alahi, A., Haque, A., and Fei-Fei, L. (2015). RGB-W:
When vision meets wireless. 2015 IEEE Internati-
onal Conference on Computer Vision (ICCV), pages
3289–3297.
Colace, F., De Santo, M., Greco, L., Lemma, S., Lombardi,
M., Moscato, V., and Picariello, A. (2014). A context-
aware framework for cultural heritage applications. In
Signal-Image Technology and Internet-Based Systems
(SITIS), 2014 Tenth International Conference on, pa-
ges 469–476. IEEE.
Cucchiara, R. and Del Bimbo, A. (2014). Visions for aug-
mented cultural heritage experience. IEEE MultiMe-
dia, 21(1):74–82.
Gallo, G., Signorello, G., Farinella, G., and Torrisi, A.
(2017). Exploiting social images to understand tou-
rist behaviour. In International Conference on Image
Analysis and Processing, volume LNCS 10485, pages
707–717. Springer.
Girshick, R. (2015). Fast r-cnn. In Proceedings of the IEEE
international conference on computer vision, pages
1440–1448.
Girshick, R., Donahue, J., Darrell, T., and Malik, J. (2014).
Rich feature hierarchies for accurate object detection
and semantic segmentation. In Proceedings of the
IEEE conference on computer vision and pattern re-
cognition, pages 580–587.
He, K., Gkioxari, G., Doll
´
ar, P., and Girshick, R. (2017).
Mask r-cnn. arXiv preprint arXiv:1703.06870.
He, K., Zhang, X., Ren, S., and Sun, J. (2014). Spatial
pyramid pooling in deep convolutional networks for
visual recognition. CoRR, abs/1406.4729.
Jiang, B., Luo, R., Mao, J., Xiao, T., and Jiang, Y. (2018).
Acquisition of localization confidence for accurate ob-
ject detection. In The European Conference on Com-
puter Vision (ECCV).
Koniusz, P., Tas, Y., Zhang, H., Harandi, M. T., Porikli, F.,
and Zhang, R. (2018). Museum exhibit identification
challenge for domain adaptation and beyond. CoRR,
abs/1802.01093.
Kuflik, T., Boger, Z., and Zancanaro, M. (2012). Analysis
and prediction of museum visitors’ behavioral pattern
types. In Ubiquitous Display Environments.
Law, H. and Deng, J. (2018). Cornernet: Detecting objects
as paired keypoints. In The European Conference on
Computer Vision (ECCV).
Liu, W., Anguelov, D., Erhan, D., Szegedy, C., Reed, S., Fu,
C.-Y., and Berg, A. C. (2016). Ssd: Single shot mul-
tibox detector. In European conference on computer
vision, pages 21–37. Springer.
Portaz, M., Kohl, M., Qu
´
enot, G., and Chevallet, J.-P.
(2017). Fully convolutional network and region pro-
posal for instance identification with egocentric vi-
sion. In Proceedings of the IEEE Conference on Com-
puter Vision and Pattern Recognition, pages 2383–
2391.
Ragusa, F., Furnari, A., Battiato, S., Signorello, G., and Fa-
rinella, G. M. (2018a). Egocentric visitors localization
in cultural sites. ACM Journal on Computing and Cul-
tural Heritage.
Ragusa, F., Guarnera, L., Furnari, A., Battiato, S., Signo-
rello, G., and Farinella, G. M. (2018b). Localiza-
tion of visitors for cultural sites management. In Pro-
ceedings of the 15th International Joint Conference
on e-Business and Telecommunications - Volume 2:
ICETE,, pages 407–413. INSTICC, SciTePress.
Raptis, D., Tselios, N. K., and Avouris, N. M. (2005).
Context-based design of mobile applications for mu-
seums: a survey of existing practices. In Mobile HCI.
Razavian, A. S., Aghazadeh, O., Sullivan, J., and Carlsson,
S. (2014). Estimating attention in exhibitions using
wearable cameras. 2014 22nd International Confe-
rence on Pattern Recognition, pages 2691–2696.
Redmon, J. and Farhadi, A. (2016). Yolo9000: Better, fas-
ter, stronger. arXiv preprint arXiv:1612.08242.
Redmon, J. and Farhadi, A. (2018). Yolov3: An incremental
improvement. CoRR, abs/1804.02767.
Ren, S., He, K., Girshick, R., and Sun, J. (2015). Faster R-
CNN: Towards real-time object detection with region
proposal networks. In Advances in neural information
processing systems, pages 91–99.
Seidenari, L., Baecchi, C., Uricchio, T., Ferracani, A., Ber-
tini, M., and Bimbo, A. D. (2017). Deep artwork de-
tection and retrieval for automatic context-aware au-
dio guides. ACM Transactions on Multimedia Com-
puting, Communications, and Applications (TOMM),
13(3s):35.
Sermanet, P., Eigen, D., Zhang, X., Mathieu, M., Fergus,
R., and Lecun, Y. (2014). Overfeat: Integrated recog-
Egocentric Point of Interest Recognition in Cultural Sites
391