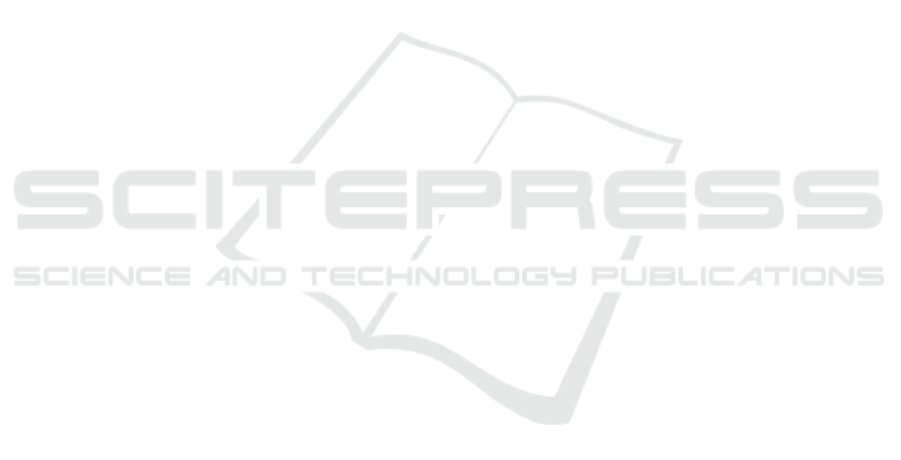
5 DISCUSSION
We demonstrated that, while the general problem of
identifying mitotic figures in whole slide images with
high accuracy, is still far from being achieved, the
outcomes of mitosis detection approaches might well
serve as an intermediate step. In preselecting the field
of interest containing the highest mitotic figure den-
sity, the algorithm can help the pathologist in deter-
mining the area of the tumor where the proliferation
is the highest. Hence, we expect that such approaches
can lead to a more reproducible grading and thus po-
tentially better tailored treatment of the patient.
The most crucial slides for the approach are slides
3 to 5, as also shown in Fig. 6(a) to 6(c). Because
the mitotic figure distribution in these slides is rather
patchy i.e. with strong regional differences (see also
Fig. 4 for absolute numbers) an arbitrary selection is
likely to not yield the area with highest mitotic count,
and thus the grading is subject to a possible strong
variance. For all of these cases, both approaches were
well able to pick an area with very high mitotic ac-
tivity, with only minor differences in performance.
The U-Net approach, while leading to a considerably
higher correlation coefficient on the overall data set,
did not lead to a significantly better overall perfor-
mance.
Our approach did not employ stain normalization
methods, as done in the majority of recent mitosis de-
tection approaches (Veta et al., 2018). This was done
in part, because the staining quality of our dataset is
relatively stable due to the usage of a tissue stainer
(ST5010 Autostainer XL, Leica, Germany) and all
slides being created and scanned in the same lab. Ad-
ditionally, we assume that with the high number of
included WSI in the present study, natural variance of
stain becomes less relavant. For application of this
approach on another (possibly smaller) data set, how-
ever, we would recommend investigating a positive
influence of such methods.
It is important to state that the results of this work
were achieved on a limited test data set for canine
mast cell tumors. While, theoretically, we would not
assume different performance on different tumors, tis-
sues or species, this should certainly be investigated.
Another important question is to what degree the im-
proved stability of region proposal, as shown in this
work, would lead to a lower inter-rater-variability in
grading, which we aim to deal with in future work.
REFERENCES
Aubreville, M., Bertram, C., Klopfleisch, R., and Maier, A.
(2018a). SlideRunner - A Tool for Massive Cell Anno-
tations in Whole Slide Images. In Maier, A., Deserno,
T. M., Handels, H., Maier-Hein, K. H., Palm, C., and
Tolxdorff, T., editors, Bildverarbeitung f
¨
ur die Medi-
zin 2018. Proceedings des Workshops vom 11. bis 13.
M
¨
arz 2018 in Erlangen, pages 309–314.
Aubreville, M., Bertram, C. A., Klopfleisch, R., and Maier,
A. (2018b). Augmented mitotic cell count using field
of interest proposal. Bildverarbeitung f
¨
ur die Medizin
2019 (accepted), arXiv:1810.00850.
Baak, J. P. A., Gudlaugsson, E., Skaland, I., Guo, L.
H. R., Klos, J., Lende, T. H., Søiland, H., Janssen, E.
A. M., and zur Hausen, A. (2008). Proliferation is the
strongest prognosticator in node-negative breast can-
cer: significance, error sources, alternatives and com-
parison with molecular prognostic markers. Breast
Cancer Research and Treatment, 115(2):241–254.
Boiesen, P., Bendahl, P. O., Anagnostaki, L., Domanski,
H., Holm, E., Idvall, I., Johansson, S., Ljungberg, O.,
Ringberg, A.,
¨
Ostberg, G., and Fern
¨
o, M. (2000). His-
tologic grading in breast cancer: reproducibility be-
tween seven pathologic departments. Acta Oncolog-
ica, 39(1):41–45.
Bonert, M. and Tate, A. J. (2017). Mitotic counts in breast
cancer should be standardized with a uniform sample
area. BioMedical Engineering OnLine, 16(1):28.
Breininger, K., Albarqouni, S., Kurzendorfer, T., Pfister,
M., Kowarschik, M., and Maier, A. K. (2018). Intra-
operative stent segmentation in X-ray fluoroscopy for
endovascular aortic repair. Int. J. Computer Assisted
Radiology and Surgery.
Chen, S., Roth, H., Dorn, S., May, M., Cavallaro, A., Lell,
M., Kachelrie
¨
s, M., Oda, H., Mori, K., and Maier, A.
(2018). Towards Automatic Abdominal Multi-Organ
Segmentation in Dual Energy CT using Cascaded 3D
Fully Convolutional Network. In Noo, F., editor, the
fifth edition of The International Conference on Im-
age Formation in X-ray Computed Tomography, pages
395–398.
Cires¸an, D. C., Giusti, A., Gambardella, L. M., and Schmid-
huber, J. (2013). Mitosis detection in breast can-
cer histology images with deep neural networks. In-
ternational Conference on Medical Image Computing
and Computer-Assisted Intervention (MICCAI), 16(Pt
2):411–418.
Edmondson, E. F., Hess, A. M., and Powers, B. E. (2014).
Prognostic Significance of Histologic Features in Ca-
nine Renal Cell Carcinomas. Veterinary Pathology,
52(2):260–268.
Elston, C. W. and Ellis, I. O. (1991). Pathological prog-
nostic factors in breast cancer. i. the value of his-
tological grade in breast cancer: experience from a
large study with long-term follow-up. Histopathology,
19(5):403–410.
Horobin, R. W. (1969). The impurities of biological dyes:
their detection, removal, occurrence and histological
significance? A review. The Histochemical Journal,
1(3):231–265.
BIOIMAGING 2019 - 6th International Conference on Bioimaging
36