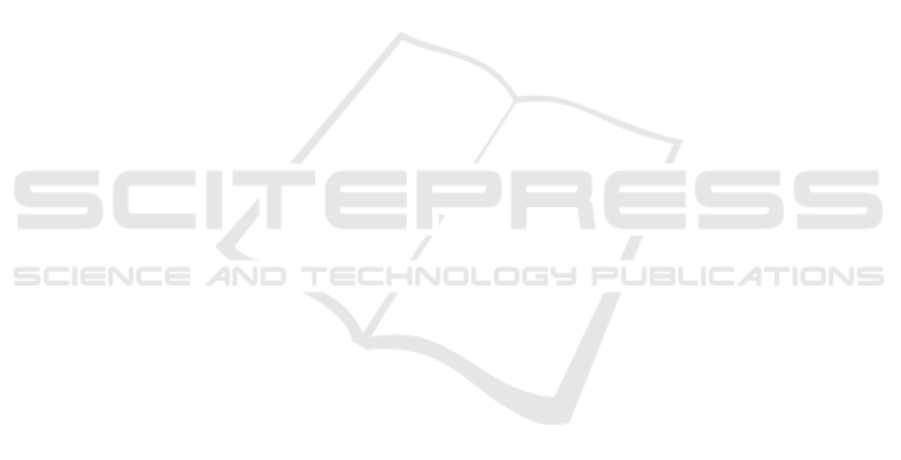
viewpoint, a reliable and high pose estimation accu-
racy is achievable.
For future improvement, we are looking for-
ward to evaluating the proposed method with multi-
dimension elevation angles as to compare with our
current single elevation angle implementation in this
paper. The improvement for obtaining a higher
pose estimation accuracy by expanding the two vie-
wpoints into several points has also been projected
as our upcoming task. To overcome the non-cascade
next viewpoint in specific cases, we also consider to
have “several” best next viewpoint where the multi-
dimensional elevation angles are utilized. This appro-
ach may be utilized with a different class of multiple
objects for having a wider scope of application and
could help the development of the human helper ro-
bot field.
ACKNOWLEDGEMENT
The authors would like to thank Toyota Motor Corpo-
ration, Ministry of Education of Government of Ma-
laysia (MOE), and Universiti Teknikal Malaysia Me-
laka (UTeM). Parts of this research were supported by
MEXT, Grant-in-Aid for Scientific Research.
REFERENCES
Chang, A. X., Funkhouser, T., Guibas, L., Hanrahan, P.,
Huang, Q., Li, Z., Savarese, S., Savva, M., Song,
S., Su, H., Xiao, J., Yi, L., and Yu, F. (2015).
ShapeNet: An information-rich 3D model repository.
arXiv:1512.03012.
Chin, R. T. and Dyer, C. R. (1986). Model-based re-
cognition in robot vision. ACM Computing Surveys,
18(1):67–108.
Collet, A. and Srinivasa, S. S. (2010). Efficient multi-view
object recognition and full pose estimation. In Pro-
ceedings of the 2010 IEEE International Conference
on Robotics and Automation, pages 2050–2055.
Doumanoglou, A., Kouskouridas, R., Malassiotis, S., and
Kim, T.-K. (2016). Recovering 6D object pose and
predicting next-best-view in the crowd. In Procee-
dings of the 2016 IEEE International Conference
on Computer Vision and Pattern Recognition, pages
3583–3592.
Erkent,
¨
O., Shukla, D., and Piater, J. (2016). Integration
of probabilistic pose estimates from multiple views.
In Proceedings of the 2016 European Conference on
Computer Vision, volume 7, pages 154–170.
Gall, J. and Lempitsky, V. (2009). Class-specific Hough fo-
rests for object detection. In Proceedings of the 2009
IEEE International Conference on Computer Vision
and Pattern Recognition, pages 1022–1029.
Kanezaki, A., Matsushita, Y., and Nishida, Y. (2018). Rota-
tionNet: Joint object categorization and pose estima-
tion using multiviews from unsupervised viewpoints.
In Proceedings of the 2018 IEEE International Con-
ference on Computer Vision and Pattern Recognition,
pages 5010–5019.
Krizhevsky, A., Sutskever, I., and Hinton, G. E. (2012).
Imagenet classification with deep convolutional neu-
ral networks. In Proceedings of the 25th Internatio-
nal Conference on Neural Information Processing Sy-
stems, pages 1097–1105.
Murase, H. and Nayar, S. K. (1995). Visual learning and
recognition of 3-D objects from appearance. Interna-
tional Journal of Computer Vision, 14(1):5–24.
Ninomiya, H., Kawanishi, Y., Deguchi, D., Ide, I., Murase,
H., Kobori, N., and Nakano, Y. (2017). Deep ma-
nifold embedding for 3D object pose estimation. In
Proceedings of the 12th Joint Conference on Compu-
ter Vision, Imaging and Computer Graphics Theory
and Applications, pages 173–178.
Sock, J., Kasaei, S. H., Lopes, L. S., and Kim, T. K. (2017).
Multi-view 6D object pose estimation and camera mo-
tion planning using RGBD images. In Proceedings of
the 2017 IEEE International Conference on Computer
Vision Workshops, pages 2228–2235.
Vikst
´
en, F., S
¨
oderberg, R., Nordberg, K., and Perwass, C.
(2006). Increasing pose estimation performance using
multi-cue integration. In Proceedings of the 2006
IEEE International Conference on Robotics and Au-
tomation, pages 3760–3767.
Zeng, A., Song, S., Yu, K., Donlon, E., Hogan, F. R.,
Bauz
´
a, M., Ma, D., Taylor, O., Liu, M., Romo, E.,
Fazeli, N., Alet, F., Dafle, N. C., Holladay, R., Mo-
rona, I., Nair, P. Q., Green, D., Taylor, I., Liu, W.,
Funkhouser, T. A., and Rodriguez, A. (2017a). Ro-
botic pick-and-place of novel objects in clutter with
multi-affordance grasping and cross-domain image
matching. arXiv/1710.01330.
Zeng, A., Yu, K.-T., Song, S., Suo, D., Walker, E., Ro-
driguez, A., and Xiao, J. (2017b). Multi-view self-
supervised deep learning for 6D pose estimation in the
amazon picking challenge. In Proceedings of the 2017
IEEE International Conference on Robotics and Auto-
mation, pages 1386–1383.
Next Viewpoint Recommendation by Pose Ambiguity Minimization for Accurate Object Pose Estimation
67