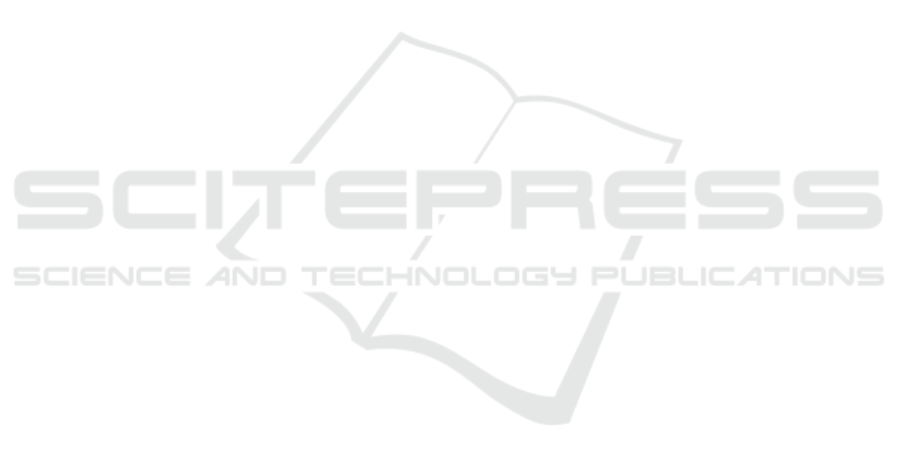
Ghojogh, B., Mohammadzade, H., and Mokari, M. (2018).
Fisherposes for human action recognition using kinect
sensor data. IEEE Sensors Journal, 18(4):1612–1627.
Gupta, A., Martinez, J., Little, J. J., and Woodham, R. J.
(2014). 3d pose from motion for cross-view action
recognition via non-linear circulant temporal encod-
ing. In Proceedings of the IEEE conference on com-
puter vision and pattern recognition, pages 2601–
2608.
Han, J., Shao, L., Xu, D., and Shotton, J. (2013). Enhanced
computer vision with microsoft kinect sensor: A re-
view. volume 43, pages 1318–1334. IEEE.
Han, S. and Lee, S. (2013). A vision-based motion capture
and recognition framework for behavior-based safety
management. Automation in Construction, 35:131–
141.
Han, Y., Zhang, P., Zhuo, T., Huang, W., and Zhang,
Y. (2018). Going deeper with two-stream convnets
for action recognition in video surveillance. Pattern
Recognition Letters, 107:83–90.
Harandi, M. T., Sanderson, C., Shirazi, S., and Lovell,
B. C. (2011). Graph embedding discriminant analy-
sis on grassmannian manifolds for improved image set
matching. In Computer Vision and Pattern Recogni-
tion (CVPR), 2011 IEEE Conference on, pages 2705–
2712. IEEE.
Hou, Y., Li, Z., Wang, P., and Li, W. (2018). Skeleton op-
tical spectra-based action recognition using convolu-
tional neural networks. IEEE Transactions on Circuits
and Systems for Video Technology, 28(3):807–811.
Hu, H., Ma, B., Shen, J., and Shao, L. (2018). Man-
ifold regularized correlation object tracking. IEEE
transactions on neural networks and learning systems,
29(5):1786–1795.
Huang, Z., Wan, C., Probst, T., and Van Gool, L. (2017).
Deep learning on lie groups for skeleton-based action
recognition. In Proceedings of the 2017 IEEE Con-
ference on Computer Vision and Pattern Recognition
(CVPR), pages 6099–6108. IEEE computer Society.
Kendall, D. G. (1984). Shape manifolds, procrustean met-
rics, and complex projective spaces. Bulletin of the
London Mathematical Society, 16(2):81–121.
Li, B., He, M., Cheng, X., Chen, Y., and Dai, Y. (2017).
Skeleton based action recognition using translation-
scale invariant image mapping and multi-scale deep
cnn. arXiv preprint arXiv:1704.05645.
Li, B., He, M., Dai, Y., Cheng, X., and Chen, Y. (2018).
3d skeleton based action recognition by video-domain
translation-scale invariant mapping and multi-scale
dilated cnn. Multimedia Tools and Applications, pages
1–21.
Lun, R. and Zhao, W. (2015). A survey of applications and
human motion recognition with microsoft kinect. In-
ternational Journal of Pattern Recognition and Artifi-
cial Intelligence, 29(05):1555008.
Marinoiu, E., Zanfir, M., Olaru, V., and Sminchisescu,
C. (2018). 3d human sensing, action and emotion
recognition in robot assisted therapy of children with
autism. In Proceedings of the IEEE Conference
on Computer Vision and Pattern Recognition, pages
2158–2167.
Meng, M., Drira, H., and Boonaert, J. (2018). Distances
evolution analysis for online and off-line human ob-
ject interaction recognition. Image and Vision Com-
puting, 70:32–45.
Meng, M., Drira, H., Daoudi, M., and Boonaert, J. (2015).
Human-object interaction recognition by learning the
distances between the object and the skeleton joints.
In Automatic Face and Gesture Recognition (FG),
2015 11th IEEE International Conference and Work-
shops on, volume 7, pages 1–6. IEEE.
Mitchel, T., Ruan, S., Gao, Y., and Chirikjian, G. S. (2018).
The globally optimal reparameterization algorithm:
an alternative to fast dynamic time warping for action
recognition in video sequences. The Computing Re-
search Repository (CoRR), pages 1807–05485.
Mora, S. V. and Knottenbelt, W. J. (2017). Deep learning
for domain-specific action recognition in tennis. In
Computer Vision and Pattern Recognition Workshops
(CVPRW), 2017 IEEE Conference on, pages 170–178.
IEEE.
Natarajan, P. and Nevatia, R. (2008). View and scale in-
variant action recognition using multiview shape-flow
models. In Computer Vision and Pattern Recognition,
2008. CVPR 2008. IEEE Conference on, pages 1–8.
IEEE.
Ofli, F., Chaudhry, R., Kurillo, G., Vidal, R., and Bajcsy, R.
(2013). Berkeley mhad: A comprehensive multimodal
human action database. In Applications of Computer
Vision (WACV), 2013 IEEE Workshop on, pages 53–
60. IEEE.
Ohn-Bar, E. and Trivedi, M. (2013). Joint angles similari-
ties and hog2 for action recognition. In Proceedings of
the IEEE conference on computer vision and pattern
recognition workshops, pages 465–470.
Oreifej, O. and Liu, Z. (2013). Hon4d: Histogram of ori-
ented 4d normals for activity recognition from depth
sequences. In Proceedings of the IEEE conference on
computer vision and pattern recognition, pages 716–
723.
Poppe, R. (2010). A survey on vision-based human action
recognition. Image and vision computing, 28(6):976–
990.
Qiao, R., Liu, L., Shen, C., and van den Hengel, A. (2017).
Learning discriminative trajectorylet detector sets for
accurate skeleton-based action recognition. Pattern
Recognition, 66:202–212.
Rahimi, S., Aghagolzadeh, A., and Ezoji, M. (2018). Hu-
man action recognition based on the grassmann multi-
graph embedding. Signal, Image and Video Process-
ing, pages 1–9.
Rahmani, H. and Bennamoun, M. (2017). Learning action
recognition model from depth and skeleton videos. In
The IEEE International Conference on Computer Vi-
sion (ICCV).
Ramanathan, M., Yau, W.-Y., and Teoh, E. K. (2014). Hu-
man action recognition with video data: research and
evaluation challenges. IEEE Transactions on human-
machine systems, 44(5):650–663.
VISAPP 2019 - 14th International Conference on Computer Vision Theory and Applications
702