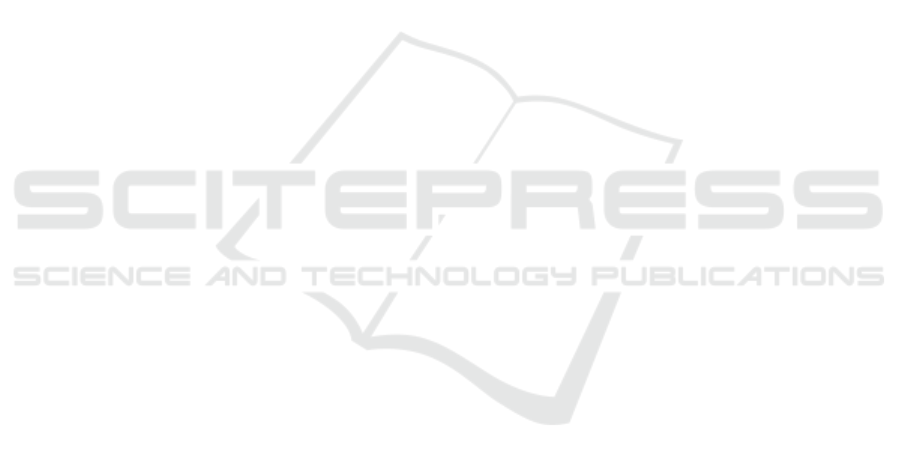
for heterogeneous distributed systems. arXiv preprint
arXiv:1512.01274.
Chen, Y., Chen, Y., Wang, X., and Tang, X. (2014). Deep
learning face representation by joint identification-
verification. In International Conference on Neural
Information Processing Systems, pages 1988–1996.
He, K., Zhang, X., Ren, S., and Sun, J. (2016). Deep resi-
dual learning for image recognition. In Proceedings of
the IEEE conference on computer vision and pattern
recognition, pages 770–778.
Hong, S., Im, W., Ryu, J., and Yang, H. S. (2017). Sspp-
dan: deep domain adaptation network for face recog-
nition with single sample per person. In Image Pro-
cessing (ICIP), 2017 IEEE International Conference
on, pages 825–829. IEEE.
Huang, G. B., Mattar, M., Berg, T., and Learned-Miller, E.
(2008). Labeled faces in the wild: A database forstu-
dying face recognition in unconstrained environments.
In Workshop on faces in’Real-Life’Images: detection,
alignment, and recognition.
Kemelmacher-Shlizerman, I., Seitz, S. M., Miller, D., and
Brossard, E. (2016). The megaface benchmark: 1 mil-
lion faces for recognition at scale. In Proceedings of
the IEEE Conference on Computer Vision and Pattern
Recognition, pages 4873–4882.
Liu, W., Wen, Y., Yu, Z., Li, M., Raj, B., and Song, L.
(2017). Sphereface: Deep hypersphere embedding for
face recognition. In The IEEE Conference on Compu-
ter Vision and Pattern Recognition (CVPR), volume 1,
page 1.
Liu, W., Wen, Y., Yu, Z., and Yang, M. (2016). Large-
margin softmax loss for convolutional neural net-
works. In ICML, pages 507–516.
Lu, C. and Tang, X. (2014). Surpassing human-level face
verification performance on lfw with gaussianface.
Computer Science.
Schroff, F., Kalenichenko, D., and Philbin, J. (2015). Fa-
cenet: A unified embedding for face recognition and
clustering. pages 815–823.
Simonyan, K. and Zisserman, A. (2014). Very deep con-
volutional networks for large-scale image recognition.
arXiv preprint arXiv:1409.1556.
Sun, Y., Wang, X., and Tang, X. (2014). Deep learning
face representation from predicting 10,000 classes. In
IEEE Conference on Computer Vision and Pattern Re-
cognition, pages 1891–1898.
Szegedy, C., Liu, W., Jia, Y., Sermanet, P., Reed, S., Angue-
lov, D., Erhan, D., Vanhoucke, V., and Rabinovich, A.
(2015). Going deeper with convolutions. In Procee-
dings of the IEEE conference on computer vision and
pattern recognition, pages 1–9.
Taigman, Y., Yang, M., Marc, and Wolf, L. (2014). Deep-
face: Closing the gap to human-level performance in
face verification. In IEEE Conference on Computer
Vision and Pattern Recognition, pages 1701–1708.
Wang, F., Xiang, X., Cheng, J., and Yuille, A. L. (2017).
Normface: l 2 hypersphere embedding for face verifi-
cation. In Proceedings of the 2017 ACM on Multime-
dia Conference, pages 1041–1049. ACM.
Wang, H., Wang, Y., Zhou, Z., Ji, X., Li, Z., Gong, D.,
Zhou, J., and Liu, W. (2018). Cosface: Large margin
cosine loss for deep face recognition. arXiv preprint
arXiv:1801.09414.
Wen, Y., Zhang, K., Li, Z., and Qiao, Y. (2016). A Discri-
minative Feature Learning Approach for Deep Face
Recognition. Springer International Publishing.
Xie, X., Cao, Z., Xiao, Y., Zhu, M., and Lu, H. (2015).
Blurred image recognition using domain adaptation.
In IEEE International Conference on Image Proces-
sing.
Yi, D., Lei, Z., Liao, S., and Li, S. Z. (2014). Lear-
ning face representation from scratch. arXiv preprint
arXiv:1411.7923.
Zhang, K., Zhang, Z., Li, Z., and Qiao, Y. (2016). Joint
face detection and alignment using multitask casca-
ded convolutional networks. IEEE Signal Processing
Letters, 23(10):1499–1503.
Zhang, S., He, R., Sun, Z., and Tan, T. (2017). Demeshnet:
Blind face inpainting for deep meshface verification.
IEEE Transactions on Information Forensics & Secu-
rity, 13(3):637–647.
Zhou, E., Cao, Z., and Yin, Q. (2015). Naive-deep face
recognition: Touching the limit of lfw benchmark or
not? Computer Science.
ID-Softmax: A Softmax-like Loss for ID Face Recognition
419