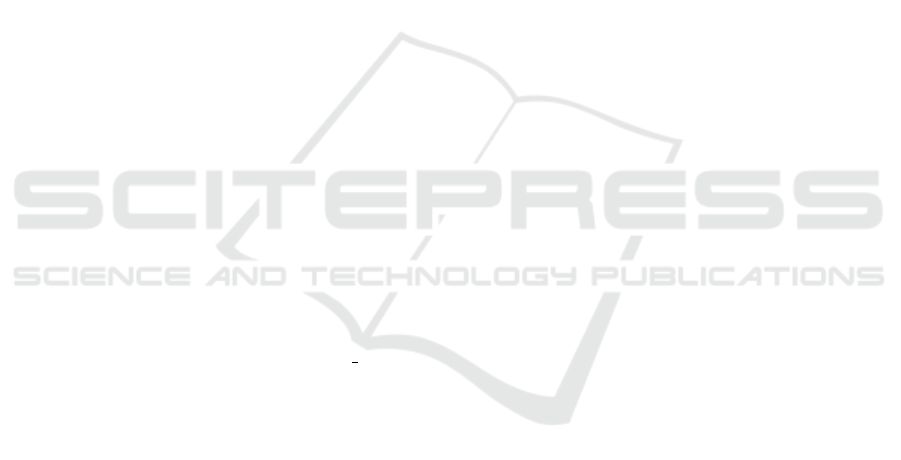
sults and provide state-of-the-art performance for
HMDB-51 dataset. Feichtenhofer et al. (Feichten-
hofer et al., 2017a) used ResNet-50 for appearance
and ResNet-152 for motion in their proposed archi-
tecture for HMDB-51 dataset. They concluded that
for the HMDB-51 dataset deeper appearance network
degrades performance while for deeper motion net-
work sizable gain is observed. The performance of
our proposed network can also be improved by using
ResNet-152 for motion stream. The highest accuracy
shown in Table 4. was released by DeepMind in July
2017 which uses 240K Kinetics dataset pre-training
with end-to-end finetuning on HMDB-51 (Carreira
and Zisserman, 2017), without Kinetics pretraining
model, their proposed two-stream I3D shows 66.4%
accuracy on the split-1 of HMDB-51. The better per-
formance is explainable by the better quality of Kine-
tics dataset that is used for pre-training the network.
5 CONCLUSION
In this paper we proposed a new method to improve
the performance for human action recognition. The
proposed method is based on an existing state-of-the-
art deep learning network, T-ResNet, which recently
shows noticeable performance for the task of human
action recognition task. We utilized the prevalent va-
riations of residual network for the extraction of mo-
tion and appearance features for benchmark dataset.
We extracted features from popular ResNet, spatio-
temporal ResNet and temporal ResNet. We evaluated
multi-kernel learning approach in comparison with
SoftMax and experiments shows that MKL SVM al-
ways outperforms SoftMax. In future, we can train
the state-of-the-art residual networks on larger data-
sets like Kinetics600 to improve the performance for
action recognition.
ACKNOWLEDGEMENTS
Sergio A Velastin has received funding from the Uni-
versidad Carlos III de Madrid, the European Unions
Seventh Framework Programme for research, techno-
logical development and demonstration under grant
agreement n
◦
600371, el Ministerio de Econom
´
ıa, In-
dustria y Competitividad (COFUND2013-51509) el
Ministerio de Educaci
´
on, cultura y Deporte (CEI-15-
17) and Banco Santander. Authors also acknowledge
support from the Higher Education Commission, Pa-
kistan.
REFERENCES
(2012). Mosek toolbox. http://docs.mosek.com/6.0/
toolbox/]. Last accessed on: 2018-06-30.
Carreira, J. and Zisserman, A. (2017). Quo vadis, action
recognition? a new model and the kinetics dataset.
In Computer Vision and Pattern Recognition (CVPR),
2017 IEEE Conference on, pages 4724–4733. IEEE.
Deng, J., Dong, W., Socher, R., Li, L.-J., Li, K., and Fei-Fei,
L. (2009). Imagenet: A large-scale hierarchical image
database. In Computer Vision and Pattern Recogni-
tion, 2009. CVPR 2009. IEEE Conference on, pages
248–255. Ieee.
Feichtenhofer, C., Pinz, A., and Wildes, R. (2016a). Spa-
tiotemporal residual networks for video action recog-
nition. In Advances in neural information processing
systems, pages 3468–3476.
Feichtenhofer, C., Pinz, A., and Wildes, R. P. (2017a). Spa-
tiotemporal multiplier networks for video action re-
cognition. In 2017 IEEE Conference on Computer
Vision and Pattern Recognition (CVPR), pages 7445–
7454. IEEE.
Feichtenhofer, C., Pinz, A., and Wildes, R. P. (2017b). Tem-
poral residual networks for dynamic scene recogni-
tion. In CVPR, volume 1, page 2.
Feichtenhofer, C., Pinz, A., and Zisserman, A. (2016b).
Convolutional two-stream network fusion for video
action recognition. In Proceedings of the IEEE Con-
ference on Computer Vision and Pattern Recognition,
pages 1933–1941.
Fernando, B. and Gould, S. (2017). Discriminatively lear-
ned hierarchical rank pooling networks. International
Journal of Computer Vision, 124(3):335–355.
Hara, K., Kataoka, H., and Satoh, Y. (2017). Learning
spatio-temporal features with 3d residual networks for
action recognition. In Proceedings of the ICCV Works-
hop on Action, Gesture, and Emotion Recognition, vo-
lume 2, page 4.
He, K., Zhang, X., Ren, S., and Sun, J. (2016). Deep resi-
dual learning for image recognition. In Proceedings of
the IEEE conference on computer vision and pattern
recognition, pages 770–778.
Herath, S., Harandi, M., and Porikli, F. (2017). Going dee-
per into action recognition: A survey. Image and vi-
sion computing, 60:4–21.
Krizhevsky, A., Sutskever, I., and Hinton, G. E. (2012).
Imagenet classification with deep convolutional neu-
ral networks. In Advances in neural information pro-
cessing systems, pages 1097–1105.
Kuehne, H., Jhuang, H., Garrote, E., Poggio, T., and Serre,
T. (2011). Hmdb: a large video database for human
motion recognition. In Computer Vision (ICCV), 2011
IEEE International Conference on, pages 2556–2563.
IEEE.
Laptev, I. (2005). On space-time interest points. Internati-
onal journal of computer vision, 64(2-3):107–123.
Ma, C.-Y., Chen, M.-H., Kira, Z., and AlRegib, G. (2017).
Ts-lstm and temporal-inception: Exploiting spatio-
temporal dynamics for activity recognition. arXiv pre-
print arXiv:1703.10667.
Human Action Recognition using Multi-Kernel Learning for Temporal Residual Network
425