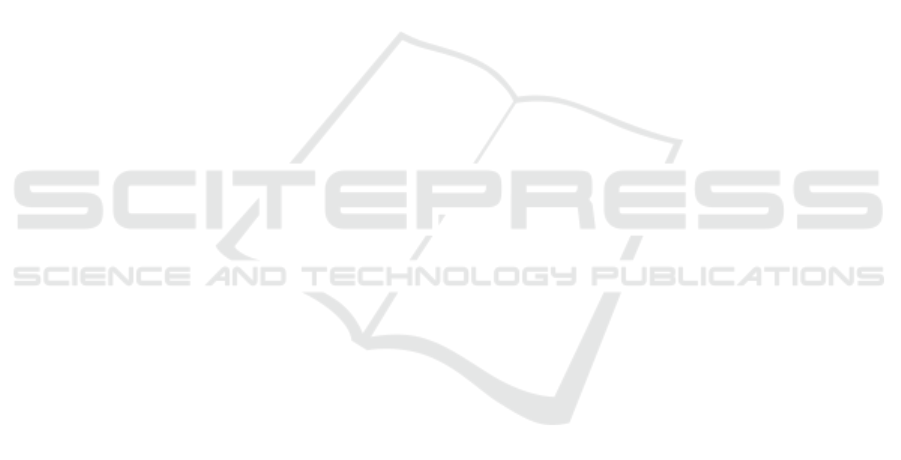
most significant results reported by the gene set analy-
sis methods revealed that the majority of the methods
reported gene sets that are not related to the known
biology of JIA. GAGE was the only method with all
of its top 20 gene sets relevant to the biology of juve-
nile arthritis. In addition, GSEA, ORA, and PAGE re-
ported relevant gene sets, with GSEA reporting fewer
but more specific gene sets. This supports the util-
ity of these methods for gene set analysis. However,
any more general conclusion would require a broader
study.
REFERENCES
Ackermann, M. and Strimmer, K. (2009). A general modu-
lar framework for gene set enrichment analysis. BMC
Bioinformatics, 10(1):47.
Bakus, G. J. (2007). Quantitative analysis of marine bio-
logical communities: field biology and environment.
John Wiley & Sons.
Barbie, D. A., Tamayo, P., Boehm, J. S., Kim, S. Y., Moody,
S. E., Dunn, I. F., Schinzel, A. C., Sandy, P., Meylan,
E., Scholl, C., et al. (2009). Systematic RNA inter-
ference reveals that oncogenic kras-driven cancers re-
quire tbk1. Nature, 462(7269):108.
Benjamini, Y. and Hochberg, Y. (1995). Controlling the
false discovery rate: a practical and powerful ap-
proach to multiple testing. Journal of the Royal Sta-
tistical Society: Series B (Statistical Methodology),
57(1):289–300.
Demmer, R. T., Behle, J. H., Wolf, D. L., Handfield, M.,
Kebschull, M., Celenti, R., Pavlidis, P., and Papa-
panou, P. N. (2008). Transcriptomes in healthy and
diseased gingival tissues. Journal of Periodontology,
79(11):2112–2124.
Dr
˘
aghici, S. (2016). Statistics and data analysis for mi-
croarrays using R and bioconductor. CRC Press.
Efron, B. and Tibshirani, R. (2007). On testing the signifi-
cance of sets of genes. The Annals of Applied Statis-
tics, 1(1):107–129.
Fung, E., Smyth, D., Howson, J., Cooper, J., Walker, N.,
Stevens, H., Wicker, L., and Todd, J. (2009). Analysis
of 17 autoimmune disease-associated variants in type
1 diabetes identifies 6q23/tnfaip3 as a susceptibility
locus. Genes and immunity, 10(2):188.
H
¨
anzelmann, S., Castelo, R., and Guinney, J. (2013).
GSVA: gene set variation analysis for microarray and
RNA-seq data. BMC Bioinformatics, 14(1):7.
Irizarry, R. A., Bolstad, B. M., Collin, F., Cope, L. M.,
Hobbs, B., and Speed, T. P. (2003). Summaries of
affymetrix genechip probe level data. Nucleic Acids
Research, 31(4):e15–e15.
Jorde, L. (2000). Linkage disequilibrium and the search
for complex disease genes. Genome research,
10(10):1435–1444.
Kim, S.-Y. and Volsky, D. J. (2005). PAGE: parametric
analysis of gene set enrichment. BMC Bioinformat-
ics, 6(1):144.
Luo, W., Friedman, M. S., Shedden, K., Hankenson, K. D.,
and Woolf, P. J. (2009). GAGE: generally applica-
ble gene set enrichment for pathway analysis. BMC
Bioinformatics, 10(1):161.
Martinez, A., Varade, J., Marquez, A., Cenit, M., Espino,
L., Perdigones, N., Santiago, J., Fern
´
andez-Arquero,
M., De La Calle, H., Arroyo, R., et al. (2008). As-
sociation of the stat4 gene with increased susceptibil-
ity for some immune-mediated diseases. Arthritis &
Rheumatism, 58(9):2598–2602.
Mathur, R., Rotroff, D., Ma, J., Shojaie, A., and Motsinger-
Reif, A. (2018). Gene set analysis methods: a system-
atic comparison. BioData Mining, 11(1):8.
Nam, D. and Kim, S.-Y. (2008). Gene-set approach for ex-
pression pattern analysis. Briefings in Bioinformatics,
9(3):189–197.
Neely, B. A. and Anderson, P. E. (2017). Complementary
domain prioritization: A method to improve biolog-
ically relevant detection in multi-omic data sets. In
Proceedings of the 10th International Joint Confer-
ence on Biomedical Engineering Systems and Tech-
nologies - Volume 3: BIOINFORMATICS, (BIOSTEC
2017), pages 68–80. INSTICC, SciTePress.
Petty, R., Laxer, R., Lindsley, C., and Wedderburn, L.
(2015). Textbook of Pediatric Rheumatology. Elsevier
Health Sciences.
Petty, R. E., Southwood, T. R., Manners, P., Baum, J., Glass,
D. N., Goldenberg, J., He, X., Maldonado-Cocco, J.,
Orozco-Alcala, J., Prieur, A.-M., et al. (2004). Inter-
national league of associations for rheumatology clas-
sification of juvenile idiopathic arthritis: second revi-
sion, edmonton, 2001. The Journal of rheumatology,
31(2):390.
Phelan, J., Thompson, S., and Glass, D. (2006). Suscepti-
bility to jra/jia: complementing general autoimmune
and arthritis traits. Genes and immunity, 7(1):1.
Prahalad, S. (2004). Genetics of juvenile idiopathic arthri-
tis: an update. Current opinion in rheumatology,
16(5):588–594.
Prahalad, S. (2006). Genetic analysis of juvenile rheuma-
toid arthritis: approaches to complex traits. Cur-
rent problems in pediatric and adolescent health care,
36(3):83.
Prahalad, S. and Glass, D. N. (2008). A comprehensive
review of the genetics of juvenile idiopathic arthritis.
Pediatric Rheumatology, 6(1):11.
Prahalad, S., Ryan, M. H., Shear, E. S., Thompson, S. D.,
Giannini, E. H., and Glass, D. N. (2000). Juve-
nile rheumatoid arthritis: linkage to hla demonstrated
by allele sharing in affected sibpairs. Arthritis &
Rheumatism: Official Journal of the American Col-
lege of Rheumatology, 43(10):2335–2338.
Ritchie, M. E., Phipson, B., Wu, D., Hu, Y., Law, C. W., Shi,
W., and Smyth, G. K. (2015). limma powers differen-
tial expression analyses for RNA-sequencing and mi-
croarray studies. Nucleic Acids Research, 43(7):e47–
e47.
Method Choice in Gene Set Analysis Has Important Consequences for Analysis Outcome
51