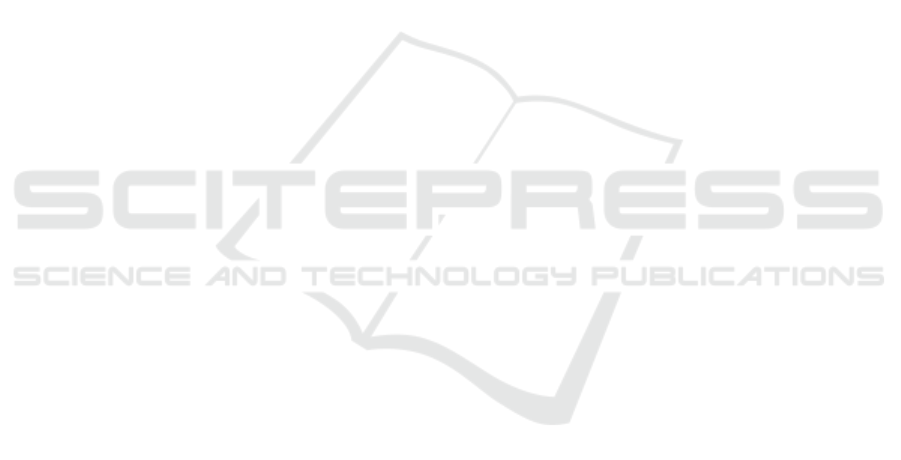
proaches reviewed in the literature. Notwithstand-
ing, a general approach has the value of being eas-
ily adapted in order to be applied in different do-
mains and in repetitive time series with different mor-
phologies, such as the case of different workstations
in manufacture environments. In human motion in-
dustrial scenarios, which are dominated by repeti-
tive movements, it was possible to detect anomalies
in multivariate time series using accelerometer, gyro-
scope and magnetometer data. However, the detec-
tion depends on the correct feature selection in or-
der to be accurate and still it may present low pre-
cision. Another important aspect is the adequate se-
lection of DBSCAN hyper-parameters. This work
demonstrated that a high volume of data and cycles
are required in order to properly automate the hyper-
parameter selection. For challenges with relatively
low volume of data either the hyper-parameter opti-
misation was achieved by user selection (at the cost
of low generalisation properties despite high perfor-
mance values) or by a Leave-One-Out methodology
which resulted in difficulties to achieve a set of val-
ues which maintain optimal characteristics for a wide
range of signals.
Future work will consist in validating the frame-
work over a more exhaustive volume of data,
which should facilitate the process of proper hyper-
parameter optimisation. Furthermore, this work was
focused on the development of the described anomaly
detection framework, but it would be important to as-
sess the impact of this system in Industrial production
lines in long-term.
ACKNOWLEDGEMENTS
This work was supported by North Portugal Regional
Operational Programme (NORTE 2020), Portugal
2020 and the European Regional Development Fund
(ERDF) from European Union through the project
Symbiotic technology for societal efficiency gains:
Deus ex Machina (DEM) [NORTE-01-0145-FEDER-
000026].
REFERENCES
Ahmad, S., Lavin, A., Purdy, S., and Agha, Z. (2017). Un-
supervised real-time anomaly detection for streaming
data. Neurocomputing, 262:134 – 147. Online Real-
Time Learning Strategies for Data Streams.
Chandola, V., Banerjee, A., and Kumar, V. (2009).
Anomaly Detection: A Survey. ACM Computing Sur-
veys, 41(3):1–58.
Chen, Z. and Li, Y. F. (2011). Anomaly detection based on
enhanced dbscan algorithm. Procedia Engineering,
15:178 – 182. CEIS 2011.
Cola, G., Avvenuti, M., Vecchio, A., Yang, G.-Z., and Lo,
B. P. L. (2015). An on-node processing approach
for anomaly detection in gait. IEEE Sensors Journal,
15:6640–6649.
Dheeru, D. and Karra Taniskidou, E. (2017). UCI machine
learning repository.
Ester, M., Kriegel, H.-P., Sander, J., and Xu, X. (1996).
A density-based algorithm for discovering clusters a
density-based algorithm for discovering clusters in
large spatial databases with noise. In Proceedings of
the Second International Conference on Knowledge
Discovery and Data Mining, KDD’96, pages 226–
231. AAAI Press.
Folgado, D., Barandas, M., Matias, R., Martins, R., Car-
valho, M., and Gamboa, H. (2018). Time Alignment
Measurement for Time Series. Pattern Recognition,
81:268–279.
Goldberger, A. L., Amaral, L. A. N., Glass, L., Hausdorff,
J. M., Ivanov, P. C., Mark, R. G., Mietus, J. E., Moody,
G. B., Peng, C.-K., and Stanley, H. E. (2000). Phys-
iobank, physiotoolkit, and physionet. Circulation,
101(23):e215–e220.
Huysmans, D., Smets, E., Raedt, W. D., Hoof, C. V., Bo-
gaerts, K., Diest, I. V., and Helic, D. (2018). Unsu-
pervised learning for mental stress detection. In Pro-
ceedings of the 11th International Joint Conference
on Biomedical Engineering Systems and Technologies
- Volume 4: BIOSIGNALS, (BIOSTEC 2018), pages
26–35. INSTICC, SciTePress.
Ren, H., Liao, X., Li, Z., and AI-Ahmari, A. (2018).
Anomaly detection using piecewise aggregate approx-
imation in the amplitude domain. Applied Intelli-
gence, 48(5):1097–1110.
Ren, H., Liu, M., Li, Z., and Pedrycz, W. (2017). A Piece-
wise Aggregate Pattern Representation Approach for
Anomaly Detection in Time Series. Knowledge-Based
Systems, 135:29–39.
Teng, M. (2010). Anomaly detection on time series. 2010
IEEE International Conference on Progress in Infor-
matics and Computing, 1:603–608.
Thuy, H. T. T., Anh, D. T., and Chau, V. T. N. (2018).
Comparing three time series segmentation methods
via novel evaluation criteria. In Proceedings - 2017
2nd International Conferences on Information Tech-
nology, Information Systems and Electrical Engineer-
ing, ICITISEE 2017, volume 2018-Janua, pages 171–
176.
BIOSIGNALS 2019 - 12th International Conference on Bio-inspired Systems and Signal Processing
170