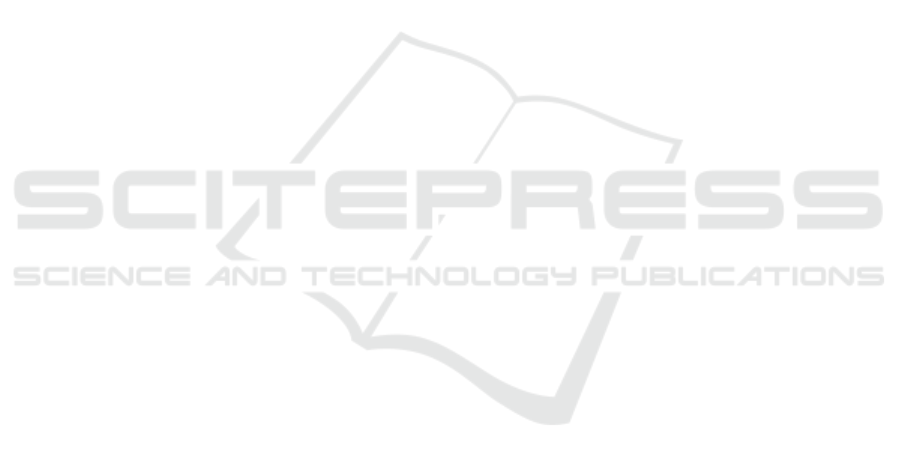
ability to adjust from scratch to the data, to choose a
new set of features and also reach great results in ac-
curacy. Even though it is necessary to test with more
activities, we are confident about the ability of this
framework to adapt to any given activity, becoming a
personalized tool for each user.
In online recognition, the solution underwent pre-
liminary tests, using three of the eight initial users.
The classifier was calibrated, by the percentile 70 of
offline results, to allow the distinction between activ-
ities and Empty periods. The classifier’s performance
that the framework is able to detect activities within
a continuous stream with an F
1
score of 74 ± 26%.
To improve the classification inside true activities, the
metric Top 2 Activity could be used as an additional
criteria, for the prediction phase. To improve Activity
Spotting, Light could serve as a trigger to identify the
beginning of an activity, which was then combined to
a binary classifier to perform Empty/Activity distinc-
tion.
The purpose of this work was to reach further
than current recognition systems, and observe activi-
ties usually ignored or classified as Walking (Door) or
Sitting (Mouse and Keyboard). Moreover, the recog-
nition of BrushTeeth could indicate if the time spent
on this activity was adequate or if it was too short.
Beyond that, the recognition of NailBiting could be
helpful in the control of this impulse.
In the future, the dataset should be increased to
more users. Also, other activities should be tested.
Considering the application in a real live situation, our
framework could be integrated into a wearable sens-
ing device with an android interface.
ACKNOWLEDGEMENTS
This work was supported by North Portugal Regional
Operational Programme (NORTE 2020), Portugal
2020 and the European Regional Development Fund
(ERDF) from European Union through the project
Symbiotic technology for societal efficiency gains:
Deus ex Machina (DEM) [NORTE-01-0145-FEDER-
000026]
REFERENCES
B, Y. F., Chang, C. K., and Chang, H. (2016). Inclusive
Smart Cities and Digital Health. 9677:148–158.
Cardoso, H. and Mendes-Moreira, J. (2016). Improving
Human Activity Classification through Online Semi-
Supervised Learning. pages 1–12.
Cilla, R., Patricio, M. A., Garc
´
ıa, J., Berlanga, A., and
Molina, J. M. (2009). Recognizing human activi-
ties from sensors using hidden markov models con-
structed by feature selection techniques. Algorithms,
2(1):282–300.
Figueira, C. (2016). Body Location Independent Activity
Monitoring. Master’s thesis.
Gaikwad, K. and Narawade, V. (2012). HMM Classifier for
Human Activity Recognition. Computer Science and
Engineering, 2(4):27–36.
Junker, H., Amft, O., Lukowicz, P., and Tr
¨
oster, G. (2010).
Gesture spotting with body-worn inertial sensors to
detect user activities. 41(2008):2010–2024.
Kabir, M. H., Hoque, M. R., Thapa, K., and Yang, S.-
H. (2016). Two-layer hidden markov model for hu-
man activity recognition in home environments. In-
ternational Journal of Distributed Sensor Networks,
12(1):4560365.
Karaman, S., Benois-Pineau, J., Dovgalecs, V., M
´
egret,
R., Pinquier, J., Andr
´
e-Obrecht, R., Ga
¨
estel, Y., and
Dartigues, J. F. (2014). Hierarchical Hidden Markov
Model in detecting activities of daily living in wear-
able videos for studies of dementia. Multimedia Tools
and Applications, 69(3):743–771.
Kreil, M., Sick, B., and Lukowicz, P. (2014). Dealing with
human variability in motion based, wearable activ-
ity recognition. 2014 IEEE International Conference
on Pervasive Computing and Communication Work-
shops, PERCOM WORKSHOPS 2014, pages 36–40.
Lara, O. D. and Labrador, M. a. (2012). A Survey on
Human Activity Recognition using Wearable Sensors.
IEEE Communications Surveys & Tutorials, pages 1–
18.
Leonardo, R. M. P. (2018). Contextual information based
on pervasive sound analysis. Master’s thesis.
Li, Z., Wei, Z., Yue, Y., Wang, H., Jia, W., Burke, L. E.,
Baranowski, T., and Sun, M. (2015). An Adap-
tive Hidden Markov Model for Activity Recognition
Based on a Wearable Multi-Sensor Device. Journal of
Medical Systems, 39(5).
Machado, I. P., Gomes, A. L., Gamboa, H., Paix
˜
ao, V.,
and Costa, R. M. (2015). Human activity data
discovery from triaxial accelerometer sensor: Non-
supervised learning sensitivity to feature extraction
parametrization. Information Processing & Manage-
ment, 51(2):204–214.
McInnes, L., Healy, J., and Astels, S. (2017). hdbscan: Hi-
erarchical density based clustering. The Journal of
Open Source Software, 2(11):205.
Rabiner, L. R. (1989). A Tutorial on Hidden Markov Mod-
els and Selected Applications in Speech Recognition.
Tapia, E. M., Intille, S. S., and Larson, K. (2004). Activity
recognisation in Home Using Simple state changing
sensors. Pervasive Computing, 3001:158–175.
van Kasteren, T. L. M., Alemdar, H., and Ersoy, C. (2011).
Effective performance metrics for evaluating activity
recognition methods. Arcs 2011, pages 1–34.
BIOSIGNALS 2019 - 12th International Conference on Bio-inspired Systems and Signal Processing
178