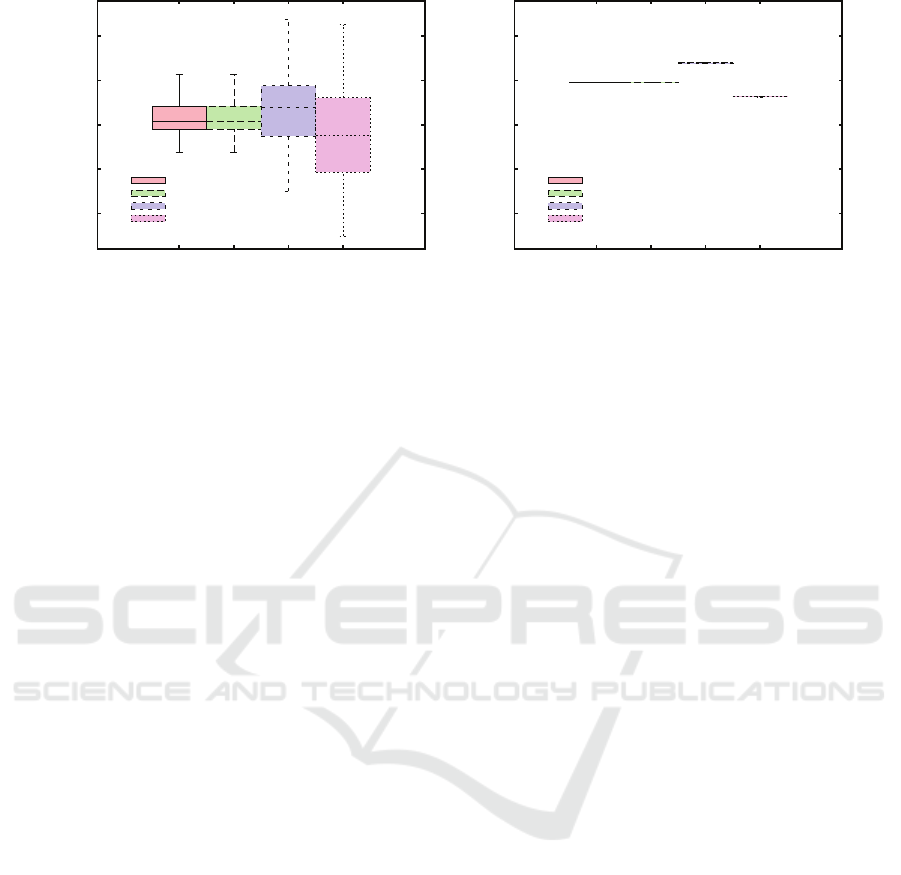
4
4.5
5
5.5
6
1 2 3 4
height [m]
CAM1
CAM2
CAM3
CAM4
(a) Experiment X
3
: camera heights of SET
(3)
4
4.5
5
5.5
6
1 2 3 4
height [m]
CAM1
CAM2
CAM3
CAM4
(b) Experiment X
4
: camera heights of SET
(3)
Figure 6: Mean and standard deviation for 100 evaluations of the camera height for experiments X
3
(a) and X
4
(b)
(cf. Section 4.1). Here, the most challenging SET
(3)
is shown. For X
4
, much smaller standard deviations are achieved.
REFERENCES
Brahmi, N., Frye, T., Marti
˜
nan, D., Dafonte, P., Abad,
X., S
´
aez, M., Cellarius, B., Gangakhedkar, S., Cao,
H., Sequeira, L., Berger, K., Theillaud, R., Otter-
bach, J., Grudnitsky, A., Villeforceix, B., Allio, S.,
Lefebvre, M., Odinot, J.-M., Tiphene, J., Servel, A.,
Fern
´
andez Barciela, A. E., Broszio, H., Cordes, K.,
and Abbas, T. (2018). 5GCAR Demonstration guide-
lines. https://5gcar.eu/. [Online; accessed 20-October-
2018].
Cordes, K., Hockner, M., Ackermann, H., Rosenhahn, B.,
and Ostermann, J. (2015). WM-SBA: Weighted multi-
body sparse bundle adjustment. In IAPR International
Conference on Machine Vision Applications (MVA),
pages 162–165.
Cordes, K., Mikulastik, P., Vais, A., and Ostermann, J.
(2009). Extrinsic calibration of a stereo camera sy-
stem using a 3D CAD model considering the uncer-
tainties of estimated feature points. In European Con-
ference on Visual Media Production (CVMP) , pages
135–143.
Das, S., Konar, A., and Chakraborty, U. K. (2005). Two im-
proved differential evolution schemes for faster global
search. In Annual Conference on Genetic and Evo-
lutionary Computation, GECCO 05, pages 991–998.
ACM.
Fallgren, M., Dillinger, M., Li, Z., Vivier, G., Abbas, T.,
Alonso-Zarate, J., Mahmoodi, T., Alli, S., Svensson,
T., and Fodor, G. (2018). On selected V2X technology
components and enablers from the 5GCAR project. In
IEEE International Symposium on Broadband Multi-
media Systems and Broadcasting (BMSB), pages 1–5.
Fern
´
andez Barciela, A. E., Servel, A., Tiphene, J., Fallgren,
M., Sun, W., Brahmi, N., Str
¨
om, E., Svensson, T.,
Bernardez, D., Zarate, J. A., Kousaridas, A., Boban,
M., Dillinger, M., Condoluci, M., Mahmoodi, T., Li,
Z., Otterbach, J., Lefebvre, M., Vivier, G., Abbas, T.,
and Wing
˚
ard, P. (2017). 5GCAR scenarios, use ca-
ses, requirements and KPIs. https://5gcar.eu/. [Online;
accessed 20-October-2018].
Hartley, R. I. and Zisserman, A. (2003). Multiple View Ge-
ometry. Cambridge University Press, second edition.
Leal-Taix
´
e, L., Milan, A., Schindler, K., Cremers, D., Reid,
I. D., and Roth, S. (2017). Tracking the trackers:
An analysis of the state of the art in multiple object
tracking. arXiv:1704.02781.
Luo, Y., Xiang, Y., Cao, K., and Li, K. (2016). A dynamic
automated lane change maneuver based on vehicle-
to-vehicle communication. Transportation Research
Part C: Emerging Technologies, 62:87–102.
Milan, A., Leal-Taix
´
e, L., Reid, I. D., Roth, S., and Schind-
ler, K. (2016). MOT16: A benchmark for multi-object
tracking. arXiv:1603.00831.
Price, K. V., Storn, R., and Lampinen, J. A. (2005). Dif-
ferential Evolution - A Practical Approach to Global
Optimization. Natural Computing Series. Springer,
Berlin, Germany.
Tang, Z., Wang, G., Liu, T., Lee, Y., Jahn, A., Liu, X., He,
X., and Hwang, J. (2017). Multiple-kernel based vehi-
cle tracking using 3D deformable model and camera
self-calibration. arXiv:1708.06831.
Tang, Z., Wang, G., Xiao, H., Zheng, A., and Hwang, J.-
N. (2018). Single-camera and inter-camera vehicle
tracking and 3D speed estimation based on fusion of
visual and semantic features. In IEEE Conference on
Computer Vision and Pattern Recognition Workshop
(CVPRw), pages 108–115.
Thorm
¨
ahlen, T., Broszio, H., and Wassermann, I. (2003).
Robust line-based calibration of lens distortion from a
single view. Proceedings of Mirage, pages 105–112.
Tsai, R. (1987). A versatile camera calibration technique
for high-accuracy 3D machine vision metrology using
off-the-shelf tv cameras and lenses. IEEE Journal on
Robotics and Automation, 3(4):323–344.
Wen, L., Du, D., Cai, Z., Lei, Z., Chang, M., Qi, H., Lim,
J., Yang, M., and Lyu, S. (2015). UA-DETRAC:
A new benchmark and protocol for multi-object de-
tection and tracking.
arXiv:1511.04136
.
VISAPP 2019 - 14th International Conference on Computer Vision Theory and Applications
536