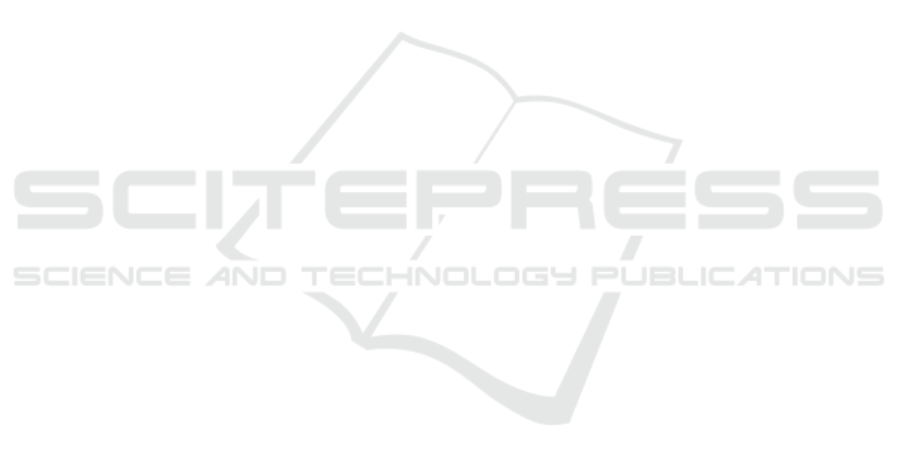
bor classifier for intrusion detection1. Computers &
security, 21(5):439–448.
Liaw, A., Wiener, M., et al. (2002). Classification and re-
gression by randomforest. R news, 2(3):18–22.
Marani, R. and Perri, A. G. (2012). An intelligent sys-
tem for continuous blood pressure monitoring on re-
mote multi-patients in real time. arXiv preprint
arXiv:1212.0651.
McBride, J. C., Zhao, X., Munro, N. B., Smith, C. D.,
Jicha, G. A., Hively, L., Broster, L. S., Schmitt, F. A.,
Kryscio, R. J., and Jiang, Y. (2014). Spectral and com-
plexity analysis of scalp eeg characteristics for mild
cognitive impairment and early alzheimer’s disease.
Computer methods and programs in biomedicine,
114(2):153–163.
Monge-
´
Alvarez, J. (2015). Higuchi and katz fractal dimen-
sion measures. https://www.mathworks.com/matlab
central/fileexchange/50290-higuchi-and-katz-fractal-
dimension-measures/content/Fractal dimension
measures/Higuchi FD.m.
Morabito, F. C., Labate, D., La Foresta, F., Bramanti, A.,
Morabito, G., and Palamara, I. (2012). Multivariate
multi-scale permutation entropy for complexity anal-
ysis of alzheimer’s disease eeg. Entropy, 14(7):1186–
1202.
Mouradian, V., Poghosyan, A., and Hovhannisyan, L.
(2015). Noninvasive continuous mobile blood pres-
sure monitoring using novel ppg optical sensor. In
Biomedical Wireless Technologies, Networks, and
Sensing Systems (BioWireleSS), 2015 IEEE Topical
Conference on, pages 1–3. IEEE.
Nitzan, M. (2011). Automatic noninvasive measurement
of arterial blood pressure. IEEE instrumentation &
measurement magazine, 14(1).
Nye, R., Zhang, Z., and Fang, Q. (2015). Continuous
non-invasive blood pressure monitoring using pho-
toplethysmography: A review. In Bioelectronics
and Bioinformatics (ISBB), 2015 International Sym-
posium on, pages 176–179. IEEE.
Patil, T. R. and Sherekar, S. (2013). Performance analy-
sis of naive bayes and j48 classification algorithm for
data classification. International journal of computer
science and applications, 6(2):256–261.
Payne, R., Symeonides, C., Webb, D., and Maxwell, S.
(2006). Pulse transit time measured from the ECG:
an unreliable marker of beat-to-beat blood pressure.
Journal of Applied Physiology, 100(1):136–141.
Powers, D. M. (2011). Evaluation: from precision, recall
and f-measure to roc, informedness, markedness and
correlation.
Program, N. H. B. P. E. et al. (2004). The seventh report of
the joint national committee on prevention, detection,
evaluation, and treatment of high blood pressure.
Rish, I. et al. (2001). An empirical study of the naive bayes
classifier. In IJCAI 2001 workshop on empirical meth-
ods in artificial intelligence, volume 3, pages 41–46.
IBM New York.
Sackl-Pietsch, E. (2010). Continuous non-invasive arterial
pressure shows high accuracy in comparison to inva-
sive intra-arterial blood pressure measurement. Un-
published manuscript.
Sahani, A. K., Ravi, V., and Sivaprakasam, M. (2014). Au-
tomatic estimation of carotid arterial pressure in art-
sens. In India Conference (INDICON), 2014 Annual
IEEE, pages 1–6. IEEE.
Sahoo, A., Manimegalai, P., and Thanushkodi, K. (2011).
Wavelet based pulse rate and blood pressure esti-
mation system from ECG and PPG signals. In
Computer, Communication and Electrical Technology
(ICCCET), 2011 International Conference on, pages
285–289. IEEE.
Schroeder, E. B., Liao, D., Chambless, L. E., Prineas, R. J.,
Evans, G. W., and Heiss, G. (2003). Hypertension,
blood pressure, and heart rate variability. Hyperten-
sion, 42(6):1106–1111.
Seo, J., Pietrangelo, S. J., Lee, H.-S., and Sodini, C. G.
(2015). Noninvasive arterial blood pressure wave-
form monitoring using two-element ultrasound sys-
tem. IEEE transactions on ultrasonics, ferroelectrics,
and frequency control, 62(4):776–784.
Shdefat, A. Y., Joo, M.-I., Choi, S.-H., and Kim, H.-C.
(2018). Utilizing ECG waveform features as new
biometric authentication method. International Jour-
nal of Electrical and Computer Engineering (IJECE),
8(2).
Shriram, R., Wakankar, A., Daimiwal, N., and Ramdasi, D.
(2010). Continuous cuffless blood pressure monitor-
ing based on ptt. In Bioinformatics and Biomedical
Technology (ICBBT), 2010 International Conference
on, pages 51–55. IEEE.
Simjanoska, M., Gjoreski, M., Gams, M., and Made-
vska Bogdanova, A. (2018). Non-invasive blood pres-
sure estimation from ECG using machine learning
techniques. Sensors, 18(4):1160.
Takahashi, N., Kuriyama, A., Kanazawa, H., Takahashi, Y.,
and Nakayama, T. (2017). Validity of spectral analysis
based on heart rate variability from 1-minute or less
ECG recordings. Pacing and Clinical Electrophysiol-
ogy.
Tanaka, S., Nogawa, M., Yamakoshi, T., and Yamakoshi,
K.-i. (2007). Accuracy assessment of a noninvasive
device for monitoring beat-by-beat blood pressure
in the radial artery using the volume-compensation
method. IEEE Transactions on Biomedical engineer-
ing, 54(10):1892–1895.
Technology, Z. (2017). Zephyr bioharness 3.0 user manual.
https://www.zephyranywhere.com/media/download/
bioharness3-user-manual.pdf.
Thomas, S. S., Nathan, V., Zong, C., Soundarapandian, K.,
Shi, X., and Jafari, R. (2016). Biowatch: A noninva-
sive wrist-based blood pressure monitor that incorpo-
rates training techniques for posture and subject vari-
ability. IEEE journal of biomedical and health infor-
matics, 20(5):1291–1300.
Trobec, R., Toma
ˇ
si
´
c, I., Rashkovska, A., Depolli, M., and
Avbelj, V. (2018a). Commercial ecg systems. In
Body Sensors and Electrocardiography, pages 101–
114. Springer.
Comparing Different Settings of Parameters Needed for Pre-processing of ECG Signals used for Blood Pressure Classification
71