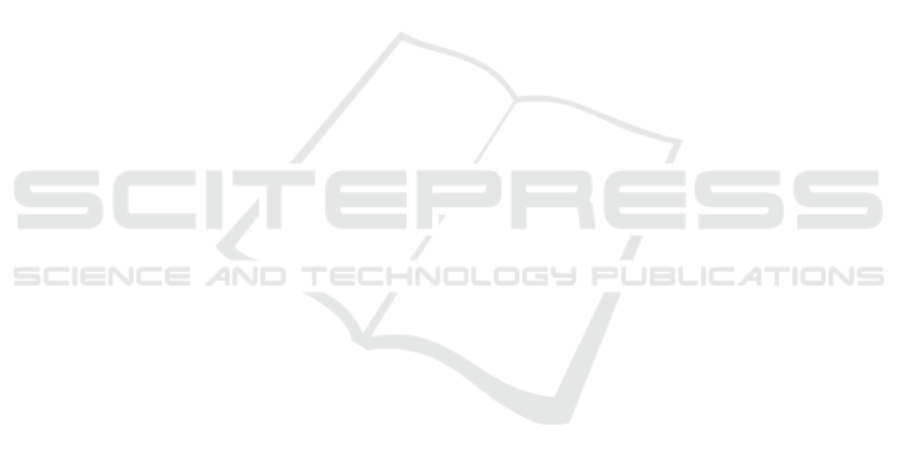
Protein- and peptide-based biosensors in artificial ol-
faction. Trends in Biotechnology, 36:1244–1258.
Dutta, R. and Dutta, R. (2006). Intelligent Bayes Classi-
fier (IBC) for ENT infection classification in hospital
environment. BioMedical Engineering Online, 5.
Ghasemi-Varnamkhasti, M., Mohtasebi, S. S., Siadat, M.,
Ahmadi, H., and Razavi, S. H. (2015). Research pa-
per: From simple classification methods to machine
learning for the binary discrimination of beers using
electronic nose data. Engineering in Agriculture, En-
vironment and Food, 8:44 – 51.
He, Q., Yan, J., Shen, Y., Bi, Y., Ye, G., Tian, F., and
Wang, Z. (2012). Classification of Electronic Nose
Data in Wound Infection Detection Based on PSO-
SVM Combined with Wavelet Transform. Intelligent
Automation and Soft Computing, 18(7):967–979.
Holmberg, M., Gustafsson, F., H
¨
ornsten, E. G., Winquist,
F., Nilsson, L. E., Ljung, L., and Lundstr
¨
om, I.
(1998). Bacteria classification based on feature ex-
traction from sensor data. Biotechnology Techniques,
12(4):319–324.
Hussain, A., Semeano, A. T. S., Palma, S. I. C. J., Pina,
A. S., Almeida, J., Medrado, B. F., P
´
adua, A. C.
C. S., Carvalho, A. L., Dion
´
ısio, M., Li, R. W. C.,
Gamboa, H., Ulijn, R. V., Gruber, J., and Roque,
A. C. A. (2017). Tunable gas sensing gels by co-
operative assembly. Advanced Functional Materials,
1700803:1–9. ¡p¿n/a¡/p¿.
Jovic, A., Brkic, K., and Bogunovic, N. (2015). A re-
view of feature selection methods with applications.
In 2015 38th International Convention on Information
and Communication Technology, Electronics and Mi-
croelectronics (MIPRO), pages 1200–1205.
Ordukaya, E. and Karlik, B. (2017). Quality control of olive
oils using machine learning and electronic nose. Jour-
nal of Food Quality, Vol 2017 (2017).
P
´
adua, A. C., Os
´
orio, D., Rodrigues, J., Santos, G., Porteira,
A., Palma, S., Roque, A., and Gamboa, H. (2018a).
Scalable and easy-to-use system architecture for elec-
tronic noses. In Proceedings of the 11th International
Joint Conference on Biomedical Engineering Systems
and Technologies, pages 179–186.
P
´
adua, A. C., Palma, S., Gruber, J., Gamboa, H., and
Roque, A. C. A. (2018b). Design and evolution of
an opto-electronic device for vocs detection. Pro-
ceedings of the 11th International Joint Conference on
Biomedical Engineering Systems and Technologies,
pages 48–55.
R
¨
ock, F., Barsan, N., and Weimar, U. (2008). Electronic
nose: Current status and future trends. Chemical Re-
views, 108(2):705 – 725.
Scott, S. M., James, D., and Ali, Z. (2007). Data analy-
sis for electronic nose systems. Microchimica Acta,
156(3/4):183 – 207.
Sebastian, R. (2015). Python Machine Learning. Packt Pub-
lishing Ltd.
Uc¸ar, A. and
¨
Ozalp, R. (2017). Efficient android elec-
tronic nose design for recognition and perception of
fruit odors using kernel extreme learning machines.
Chemometrics and Intelligent Laboratory Systems,
166:69 – 80.
Wasilewski, T., Ge¸bicki, J., and Kamysz, W. (2017).
Bioelectronic nose: Current status and perspectives.
Biosensors and Bioelectronics, 87:480–494.
Wilson, A. D. (2012). Review of electronic-nose tech-
nologies and algorithms to detect hazardous chemi-
cals in the environment. Procedia Technology, 1(First
World Conference on Innovation and Computer Sci-
ences (INSODE 2011)):453 – 463.
Xu, L., He, J., Duan, S., Wu, X., and Wang, Q. (2016).
Comparison of machine learning algorithms for con-
centration detection and prediction of formaldehyde
based on electronic nose. Sensor Review, 36(2):207.
Yan, J., Xiuzhen, G., Shukai, D., Pengfei, J., Lidan, W.,
Chao, P., and Songlin, Z. (2015). Electronic nose fea-
ture extraction methods: A review. Sensors, Vol 15,
Iss 11, Pp 27804-27831 (2015), (11):27804.
BIODEVICES 2019 - 12th International Conference on Biomedical Electronics and Devices
46