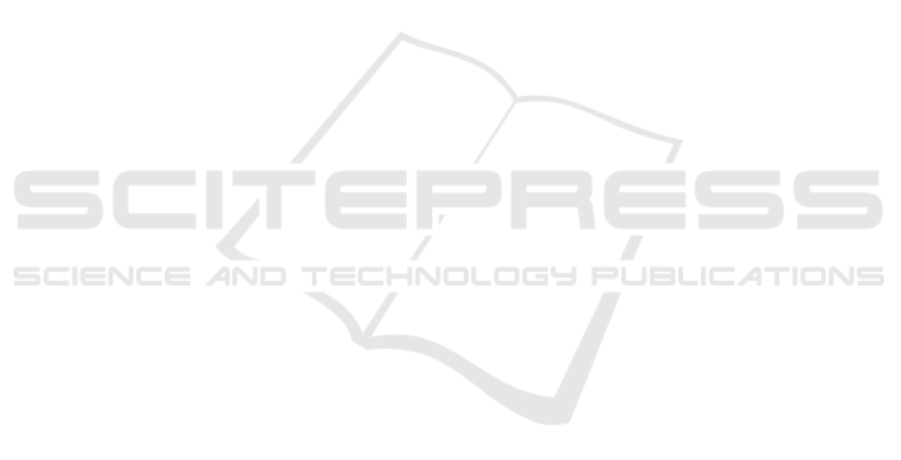
interesting to test our approach on another genome-
wide PCHi-C interaction dataset, in order to assess
both: 1) the applicability of Base11 metrics for dis-
crimination between cell types, other than blood cells
studied here; and 2) to analyse the similarity of topo-
logical structure and component behaviour of interac-
tion graphs in order to assess how the properties ob-
served for haematopoietic cells generalises to other
cell types. Unfortunately, as far as we know, an-
other appropriate PCHi-C dataset covering multiple
cell types has yet to become available.
There is also a good potential to further extend
the graph topology based approach that we have used
here. It has been quite successful to show that topo-
logical properties alone could be quite informative
for discrimination between different cell types and
also for assigning biological meaning to specific com-
ponents on interaction graphs. At the same time
some useful information in the current graph repre-
sentation is absent, notably, an edge in an interac-
tion graph might represent an interaction that forms
a well-defined loop on a chromosome (if the distance
between interaction segments is limited and there are
no intermediate interactions between them), or it can
represent a long range interaction with a far less ob-
vious biological role. We have plans to further de-
velop the mathematical formalism for description of
interaction graphs in order to incorporate and analyse
such features, although additional studies are needed
to determine the best way to achieve this.
ACKNOWLEDGEMENTS
The research was supported by ERDF project
1.1.1.1/16/A/135.
REFERENCES
Ay, F., Bailey, T., and Noble, W. (2014). Statistical con-
fidence estimation for Hi-C data reveals regulatory
chromatin contacts. Genome Research, 24(6):999–
1011.
Belton, J., McCord, R., et al. (2012). Hi-C: A com-
prehensive technique to capture the conformation of
genomes. Methods, 58(3):268–276. 3D chromatin ar-
chitecture.
Cairns, J., Freire-Pritchett, P., et al. (2016). CHiCAGO: ro-
bust detection of DNA looping interactions in capture
Hi-C data. Genome Biol, 17:127.
Celms, E., Cerans, K., et al. (2018). Application of graph
clustering and visualisation methods to analysis of
biomolecular data. Communications in Computer and
Information Science, 838:243–257.
Chen, E., Tan, C., et al. (2013). Enrichr: interactive and
collaborative html5 gene list enrichment analysis tool.
BMC Bioinformatics, 14:128.
Das, A., Yang, C., et al. (2018). High-resolution map-
ping and dynamics of the transcriptome, transcription
factors, and transcription co-factor networks in classi-
cally and alternatively activated macrophages. Front.
Immunol., 9:22.
Dekker, J., Rippe, K., et al. (2002). Capturing chromosome
conformation. Science, 295(5558):1306–1311.
DeMaere, M. and Darling, A. (2016). Deconvoluting sim-
ulated metagenomes: the performance of hard- and
soft- clustering algorithms applied to metagenomic
chromosome conformation capture (3C). PeerJ,
4:e2676.
Dryden, N., Broome, L., et al. (2014). Unbiased analysis of
potential targets of breast cancer susceptibility loci by
capture Hi-C. Genome Research, 24:1854–1868.
Forcato, M., Nicoletti, C., et al. (2017). Comparison of
computational methods for Hi-C data analysis. Nature
Methods, 14:679–685.
Guimaraes, J. and Zavolan, M. (2016). Patterns of riboso-
mal protein expression specify normal and malignant
human cells. Genome Biology, 17:236.
J
¨
ager, R., Migliorini, G., et al. (2015). Capture Hi-C iden-
tifies the chromatin interactome of colorectal cancer
risk loci. Nature Communications, 6(6178).
Javierre, B., Burren, O., et al. (2016). Lineage-specific
genome architecture links enhancers and non-coding
disease variants to target gene promoters. Cell,
167(5):1369–1384.
Kanki, Y., Nakaki, R., et al. (2017). Dynamically and epige-
netically coordinated GATA/ETS/SOX transcription
factor expression is indispensable for endothelial cell
differentiation. Nucleic Acids Research, 45(8):4344–
4358.
Kuleshow, M., Jones, M., et al. (2016). Enrichr: a compre-
hensive gene set enrichment analysis web server 2016
update. Nucleic Acids Research, 44:W90–W97.
Lajoie, B., Dekker, J., and Kaplan, N. (2015). The hitch-
hiker’s guide to Hi-C analysis: Practical guidelines.
Methods, 72:65–75.
Lavin, Y., Mortha, A., et al. (2016). Regulation of
macrophage development and function in peripheral
tissues. Nature Reviews Immunology, 15(12):731–
744.
Lieberman-Aiden, E. van Berkum, E. et al. (2009). Com-
prehensive mapping of long-range interactions reveals
folding principles of the human genome. Science,
326(5950):289–293.
Martin, P., McGovern, A., et al. (2015). Capture Hi-C re-
veals novel candidate genes and complex long-range
interactions with related autoimmune risk loci. Nature
Communications, 6(10069).
McDonel, P., Demmers, J., et al. (2012). Sin3a is essential
for the genome integrity and viability of pluripotent
cells. Dev. Biol., 363(1):62–73.
Mifsud, B., Tavares-Cadete, F., et al. (2015). Mapping long-
range promoter contacts in human cells with high-
BIOINFORMATICS 2019 - 10th International Conference on Bioinformatics Models, Methods and Algorithms
88