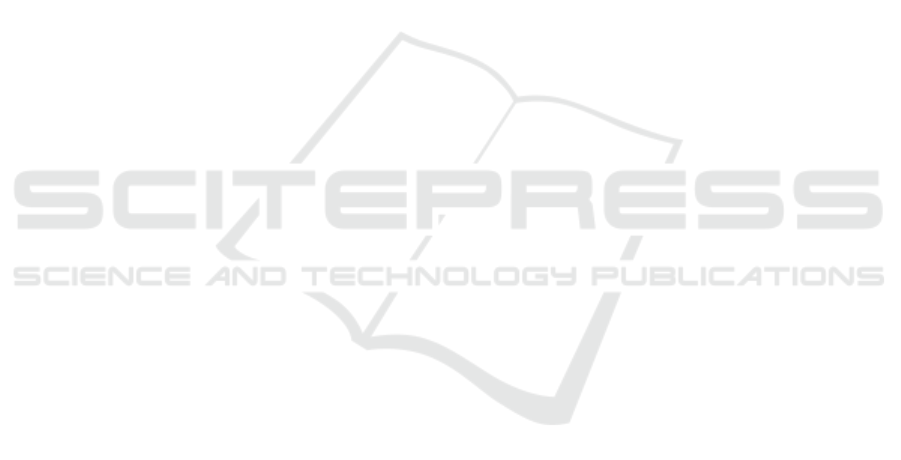
6 CONCLUDING REMARKS
This paper presents the development of a combined
approach based on sEMG and inertial sensors for
the evaluation of physiotherapy exercises. The ap-
plicability of our approach lies in the implementa-
tion on biofeedback systems to optimize home-based
exercise execution. sEMG signal was used to iden-
tify temporal intervals in which muscular activation
was present. This way, exercise repetitions were seg-
mented into time windows where features related with
human posture were extracted. Then, these features
were fed to DT, KNN, RF, and SVM classifiers, which
were able to distinguish between correct execution
and deviations with an accuracy ≥92%. As part of our
ongoing research, we will validate the proposed sys-
tem on more extensive datasets. The sEMG segmen-
tation will be assessed in a more controlled environ-
ment, using simulated data, to permit the evaluation
of the temporal misalignment between the detected
onset/offsets and groundtruth. The models proposed
will be tested on a more extended dataset, comprising
variability in terms of age and clinical history.
ACKNOWLEDGEMENTS
We acknowledge all participants who participated
in data collection. We would like to acknowl-
edge the financial support obtained from the project
Physio@Home: Extending Physiotherapy Programs
to People’s Home, co-funded by Portugal 2020,
framed under the COMPETE 2020 (Operational Pro-
gramme Competitiveness and Internationalization)
and European Regional Development Fund (ERDF)
from European Union (EU), with operation code
POCI-01-0247-FEDER-017863.
REFERENCES
Alexandre, R. and Postolache, O. (2018). Wearable and iot
technologies application for physical rehabilitation. In
2018 International Symposium in Sensing and Instru-
mentation in IoT Era (ISSI), pages 1–6. IEEE.
Barandas, M., Gamboa, H., and Fonseca, J. (2015). A real
time biofeedback system using visual user interface
for physical rehabilitation. Procedia Manufacturing,
3:823–828.
Bassett, S. F. (2003). The assessment of patient adherence
to physiotherapy rehabilitation. New Zealand journal
of physiotherapy, 31(2):60–66.
Bevilacqua, A., Huang, B., Argent, R., Caulfield, B., and
Kechadi, T. (2018). Automatic classification of knee
rehabilitation exercises using a single inertial sensor:
a case study.
De Luca, C. J. (1997). The use of surface electromyography
in biomechanics. Journal of applied biomechanics,
13(2):135–163.
Ferreira, C., Guimar
˜
aes, V., Santos, A., and Sousa, I.
(2014). Gamification of stroke rehabilitation exercises
using a smartphone. In Proceedings of the 8th Inter-
national Conference on Pervasive Computing Tech-
nologies for Healthcare, pages 282–285. ICST (In-
stitute for Computer Sciences, Social-Informatics and
Telecommunications Engineering).
Figueira, C., Matias, R., and Gamboa, H. (2016). Body
location independent activity monitoring. In BIOSIG-
NALS, pages 190–197.
Fraunhofer AICOS (2016). A Day with Pandlets. Technical
report, Fraunhofer Portugal AICOS.
Ghasemzadeh, H., Jafari, R., and Prabhakaran, B. (2010).
A body sensor network with electromyogram and in-
ertial sensors: Multimodal interpretation of muscular
activities. IEEE transactions on information technol-
ogy in biomedicine, 14(2):198–206.
Giggins, O. M., Persson, U. M., and Caulfield, B. (2013).
Biofeedback in rehabilitation. Journal of neuroengi-
neering and rehabilitation, 10(1):60.
Giggins, O. M., Sweeney, K. T., and Caulfield, B. (2014).
Rehabilitation exercise assessment using inertial sen-
sors: a cross-sectional analytical study. Journal of
neuroengineering and rehabilitation, 11(1):158.
Hodges, P. W. and Bui, B. H. (1996). A compari-
son of computer-based methods for the determina-
tion of onset of muscle contraction using electromyo-
graphy. Electroencephalography and Clinical Neu-
rophysiology/Electromyography and Motor Control,
101(6):511–519.
Huang, B., Giggins, O., Kechadi, T., and Caulfield, B.
(2016). The limb movement analysis of rehabilita-
tion exercises using wearable inertial sensors. In En-
gineering in Medicine and Biology Society (EMBC),
2016 IEEE 38th Annual International Conference of
the, pages 4686–4689. IEEE.
Liu, L., Chen, X., Lu, Z., Cao, S., Wu, D., and Zhang,
X. (2017). Development of an emg-acc-based upper
limb rehabilitation training system. IEEE Transac-
tions on Neural Systems and Rehabilitation Engineer-
ing, 25(3):244–253.
Pereira, A., Guimar
˜
aes, V., and Sousa, I. (2017). Joint an-
gles tracking for rehabilitation at home using inertial
sensors: a feasibility study. In Proceedings of the
11th EAI International Conference on Pervasive Com-
puting Technologies for Healthcare, pages 146–154.
ACM.
Rodrigues, J., Folgado, D., Belo, D., and Gamboa, H.
(2019). Ssts: A syntactic tool for pattern search on
time series. Information Processing & Management,
56(1):61–76.
Roy, S. H., Cheng, M. S., Chang, S.-S., Moore, J., De Luca,
G., Nawab, S. H., and De Luca, C. J. (2009). A
combined semg and accelerometer system for mon-
itoring functional activity in stroke. IEEE Transac-
Physiotherapy Exercises Evaluation using a Combined Approach based on sEMG and Wearable Inertial Sensors
81